Asymptotic analysis of ML-covariance parameter estimators based on covariance approximations
arxiv(2023)
摘要
Given a zero-mean Gaussian random field with a covariance function that belongs to a parametric family of covariance functions, we introduce a new notion of likelihood approximations, termed truncated likelihood functions. Truncated-likelihood functions are based on direct functional approximations of the presumed family of covariance functions. For compactly supported covariance functions, within an increasing-domain asymptotic framework, we provide sufficient conditions under which consistency and asymptotic normality of estimators based on truncated-likelihood functions are preserved. We apply our result to the family of generalized Wendland covariance functions and discuss several examples of Wendland approximations. For families of covariance functions that are not compactly supported, we combine our results with the covariance tapering approach and show that ML estimators, based on truncated-tapered likelihood functions, asymptotically minimize the Kullback-Leibler divergence, when the
更多查看译文
关键词
Gaussian random fields,compactly supported covariance functions,likelihood approximations,consistency,asymptotic normality,covariance tapering
AI 理解论文
溯源树
样例
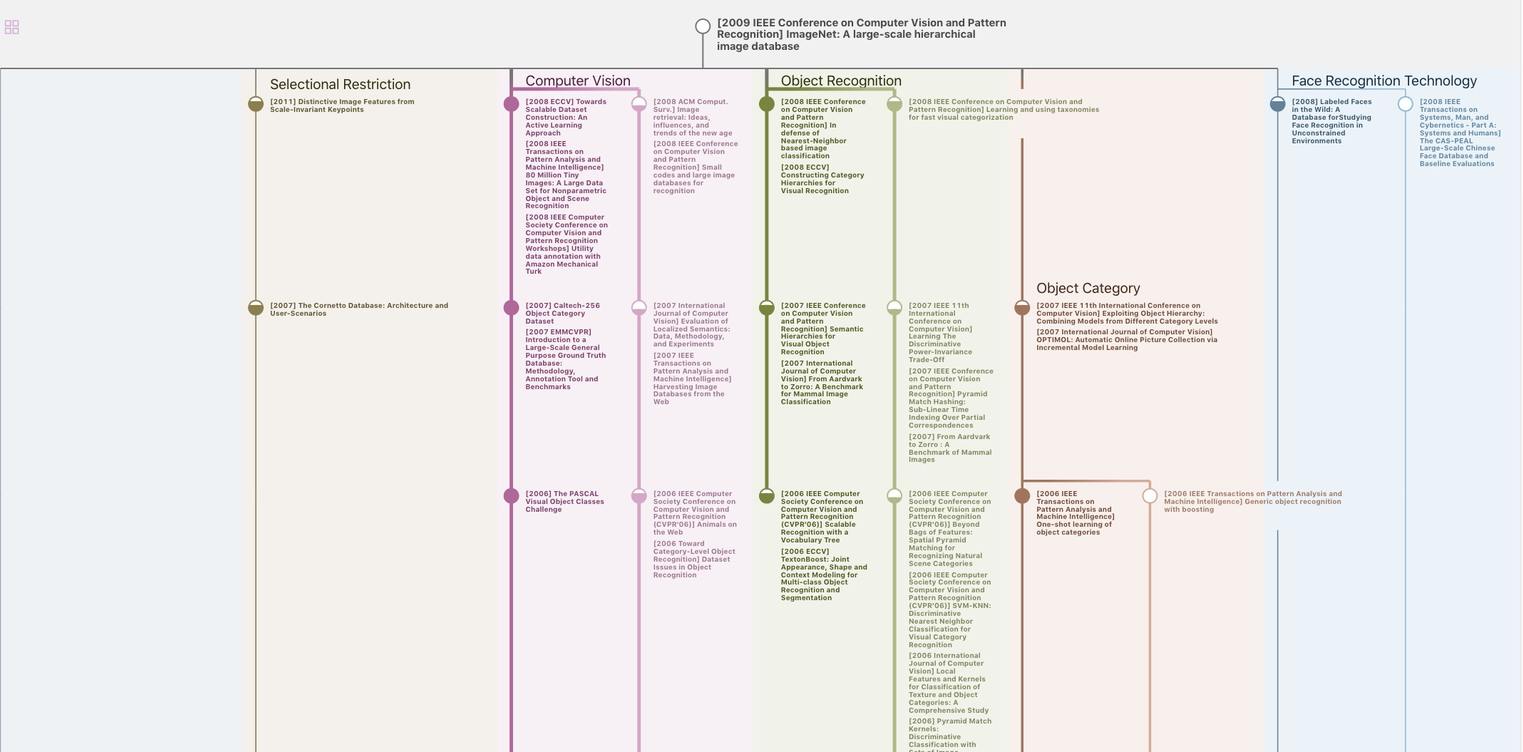
生成溯源树,研究论文发展脉络
Chat Paper
正在生成论文摘要