Explainable Artificial Intelligence Methods in Combating Pandemics: A Systematic Review
IEEE Reviews in Biomedical Engineering(2023)
摘要
Despite the myriad peer-reviewed papers demonstrating novel Artificial Intelligence (AI)-based solutions to COVID-19 challenges during the pandemic, few have made a significant clinical impact, especially in diagnosis and disease precision staging. One major cause for such low impact is the lack of model transparency, significantly limiting the AI adoption in real clinical practice. To solve this problem, AI models need to be explained to users. Thus, we have conducted a comprehensive study of Explainable Artificial Intelligence (XAI) using PRISMA technology. Our findings suggest that XAI can improve model performance, instill trust in the users, and assist users in decision-making. In this systematic review, we introduce common XAI techniques and their utility with specific examples of their application. We discuss the evaluation of XAI results because it is an important step for maximizing the value of AI-based clinical decision support systems. Additionally, we present the traditional, modern, and advanced XAI models to demonstrate the evolution of novel techniques. Finally, we provide a best practice guideline that developers can refer to during the model experimentation. We also offer potential solutions with specific examples for common challenges in AI model experimentation. This comprehensive review, hopefully, can promote AI adoption in biomedicine and healthcare.
更多查看译文
关键词
COVID-19,electronic health records,expla- inable artificial intelligence,explanation evaluation,explanation generation,explanation representation,medical imaging
AI 理解论文
溯源树
样例
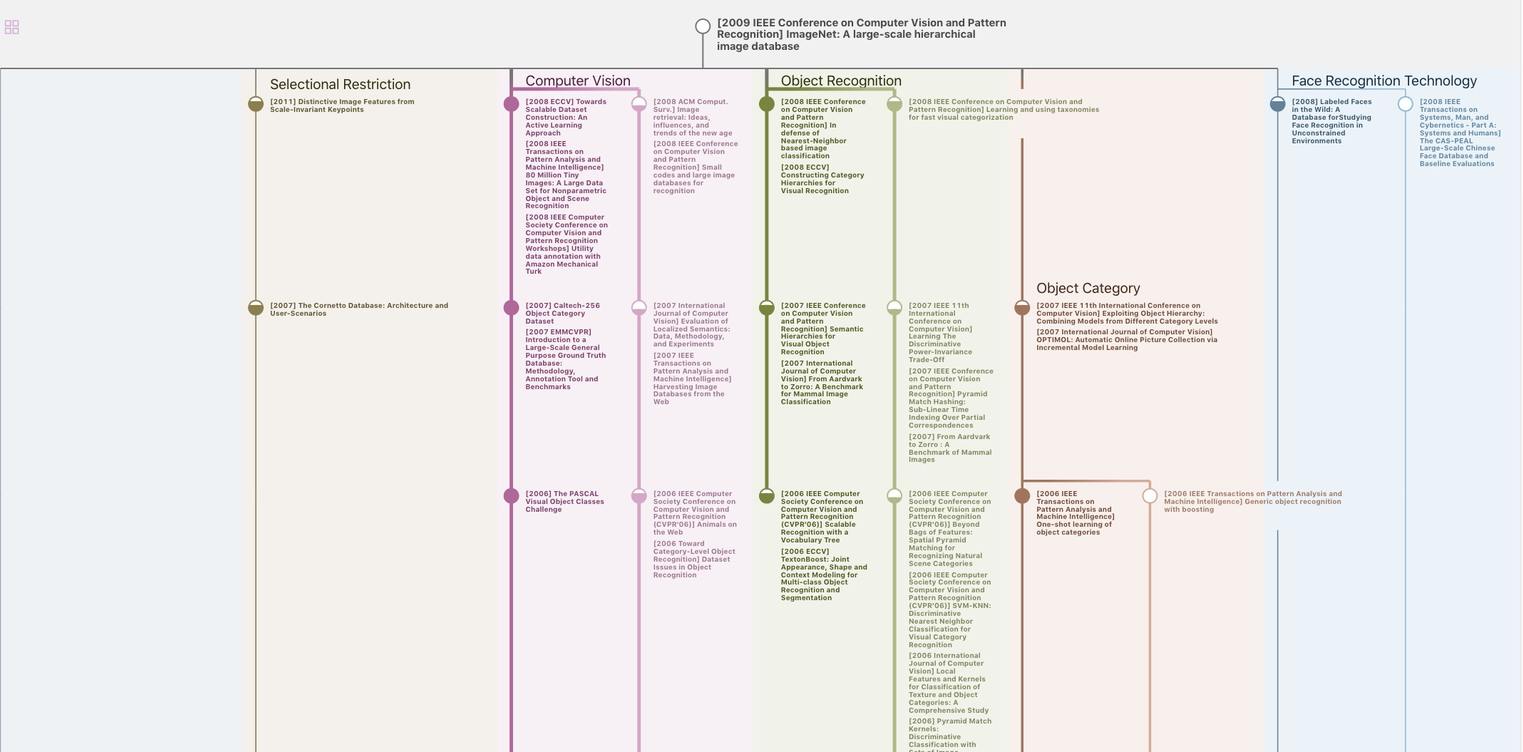
生成溯源树,研究论文发展脉络
Chat Paper
正在生成论文摘要