The challenge of comprehensive nailfold videocapillaroscopy practice: a further contribution
Clinical and experimental rheumatology(2022)
摘要
Objective Although classification systems and scores for capillaroscopy interpretation have been published, there is a lack of homogenisation for the procedure, especially in the way and place the images are taken, the counting of the capillaries and the measuring of their size. Our objective is to provide a deep learning-based software to obtain objective and exhaustive data for the whole nailfold without increasing the time or effort needed to do the examination, or requiring expensive equipment. Methods An automated software to count nailfold capillaries has been designed, through an exploratory image dataset of 2,713 images with 18,000 measurements of 3 different types. Subsequently, application rules have been created to detect the morphology of nailfold videocapillaroscopy images, through a training set of images. The software reliability has been evaluated with standard metrics used in the machine learning field for object detection tasks, comparing automatic and manual counting on the same NVC images. Results A mean average precision (mAP) of 0.473 is achieved for detecting and classifying capillaries and haemorrhages by their shape, and a mAP of 0.515 is achieved for detecting and classifying capillaries by their size. A precision of 83.84% and a recall of 92.44% in the identification of capillaries was estimated. Conclusion Deep learning is a useful tool in nailfold videocapillaroscopy that allows to analyse objectively and homogeneously images taken with multiple devices. It should make the assessment of the capillary morphology in nailfold video capillaroscopy easier, quicker, more complete and accessible to everyone.
更多查看译文
关键词
nailfold capillaroscopy,deep learning,machine learning,Raynaud?s syndrome,systemic sclerosis
AI 理解论文
溯源树
样例
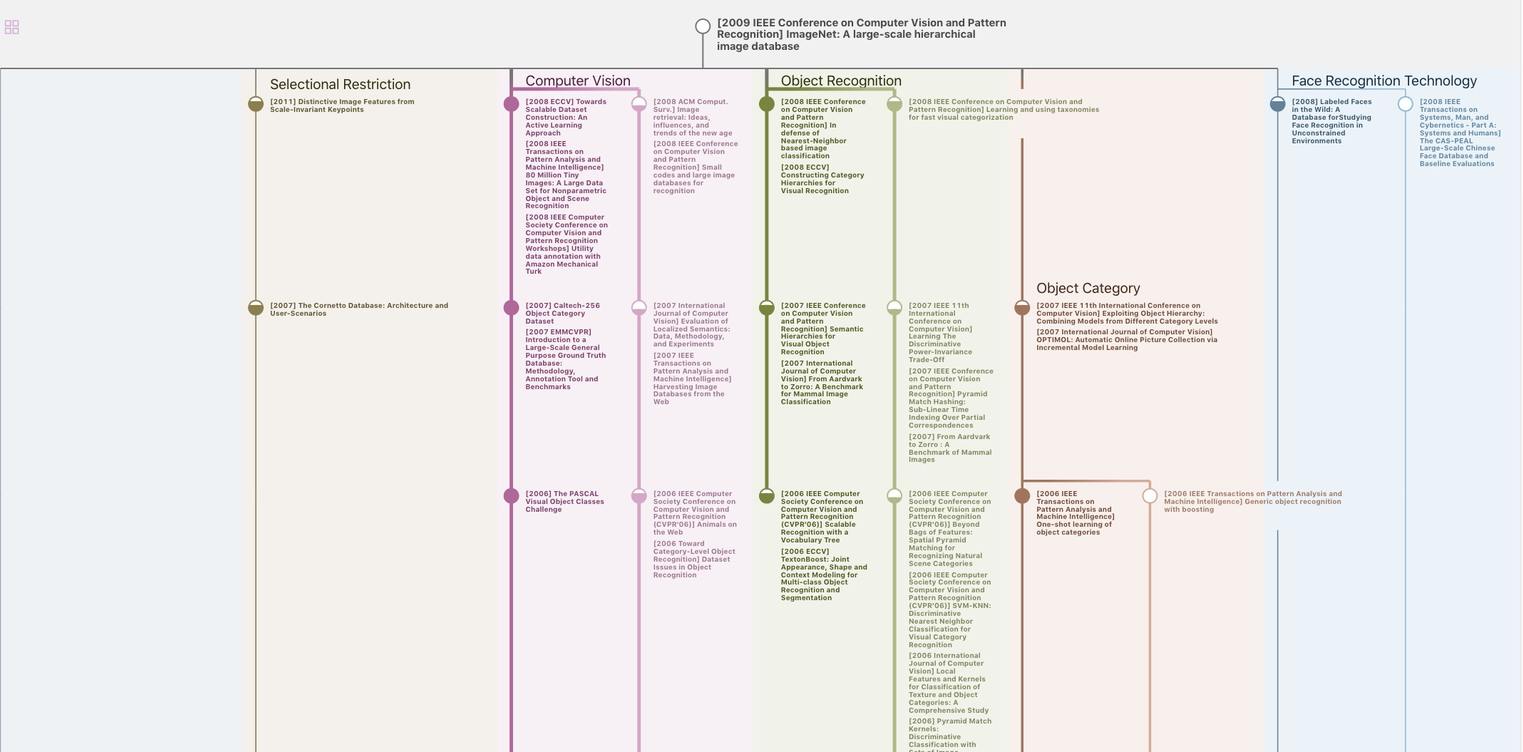
生成溯源树,研究论文发展脉络
Chat Paper
正在生成论文摘要