Selection of 51 predictors from 13,782 candidate multimodal features using machine learning improves coronary artery disease prediction
PATTERNS(2021)
摘要
Current cardiovascular risk assessment tools use a small number of predictors. Here, we study how machine learning might: (1) enable principled selection from a large multimodal set of candidate variables and (2) improve prediction of incident coronary artery disease (CAD) events. An elastic net-based Cox model (ML4H(EN-COX)) trained and evaluated in 173,274 UK Biobank participants selected 51 predictors from 13,782 candidates. Beyond most traditional risk factors, ML4H(EN-COX) selected a polygenic score, waist circumference, socioeconomic deprivation, and several hematologic indices. A more than 30-fold gradient in 10-year risk estimates was noted across ML4H(EN-COX) quintiles, ranging from 0.25% to L8%. ML4H(EN-COX) improved discrimination of incident CAD (C-statistic = 0.796) compared with the Framingham risk score, pooled cohort equations, and QRISK3 (range 0.754-0.761). This approach to variable selection and model assessment is readily generalizable to a broad range of complex datasets and disease endpoints.
更多查看译文
关键词
Framingham risk score,QRISK3,coronary artery disease,machine learning,pooled cohort equations,risk prediction
AI 理解论文
溯源树
样例
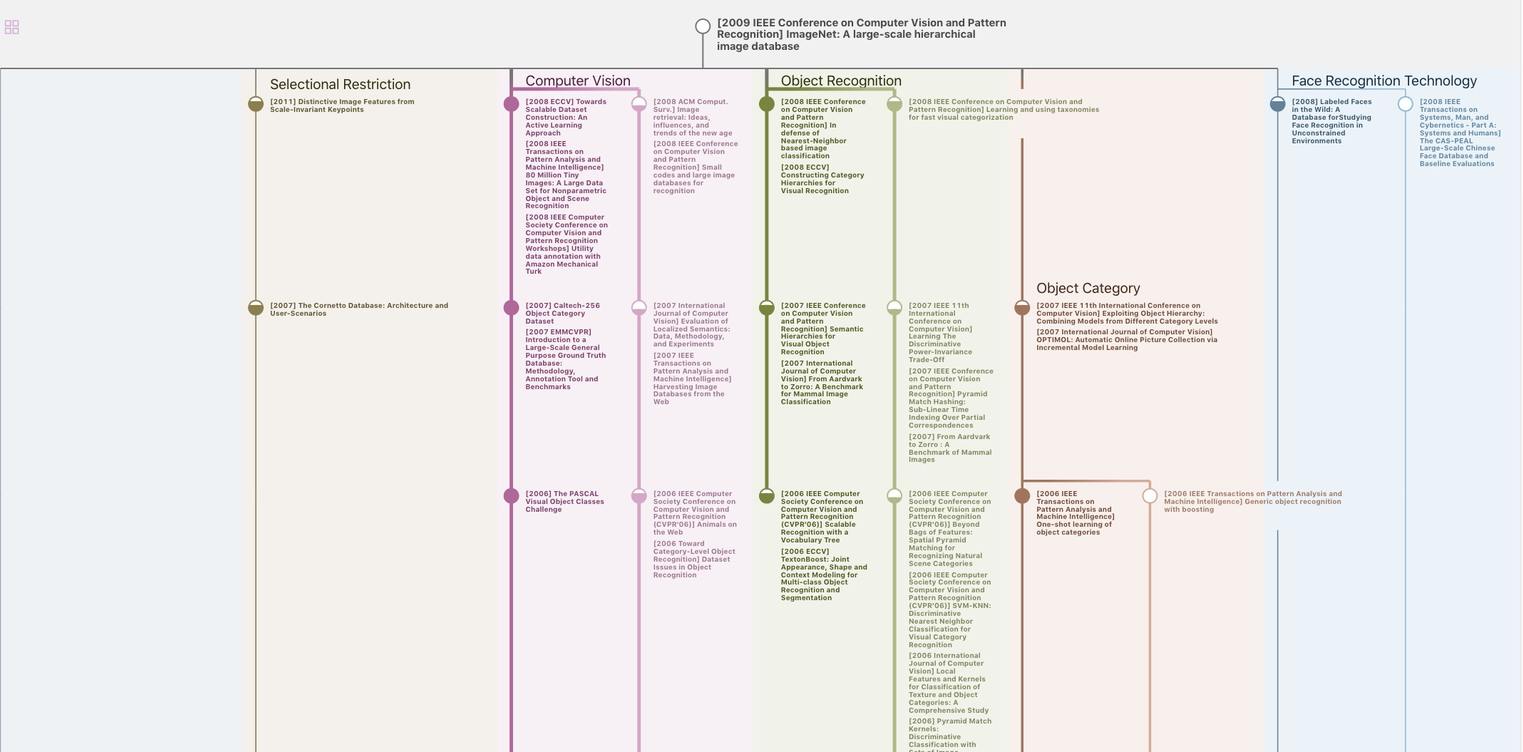
生成溯源树,研究论文发展脉络
Chat Paper
正在生成论文摘要