Machine Learning Methods to Predict Survival in Patients Following Traumatic Aortic Injury
medRxiv(2021)
摘要
The National Trauma Data Bank (NTDB) is a resource of diagnostic, treatment, and outcomes information in trauma patients. We leverage the NTDB and machine learning techniques to predict survival following traumatic aortic injury. We create two predictive models using the NTDB - 1) using all data and, 2) using only data available in the first hour after arrival (prospective data). Seven discriminative model types were tested before and after feature engineering to reduce dimensionality. The top performing model was XGBoost, achieving an overall accuracy of 0.893 using all data and 0.855 using prospective data. Feature engineering improved performance of all models. Glasgow Coma Scale score was the most important factor for survival, and thoracic endovascular aortic repair was more common in patients that survived. Smoking, pneumonia, and urinary tract infection predicted poor survival. We also note concerning disparities in outcomes for black and uninsured patients that may reflect differences in care.
更多查看译文
关键词
traumatic aortic injury,machine learning,survival
AI 理解论文
溯源树
样例
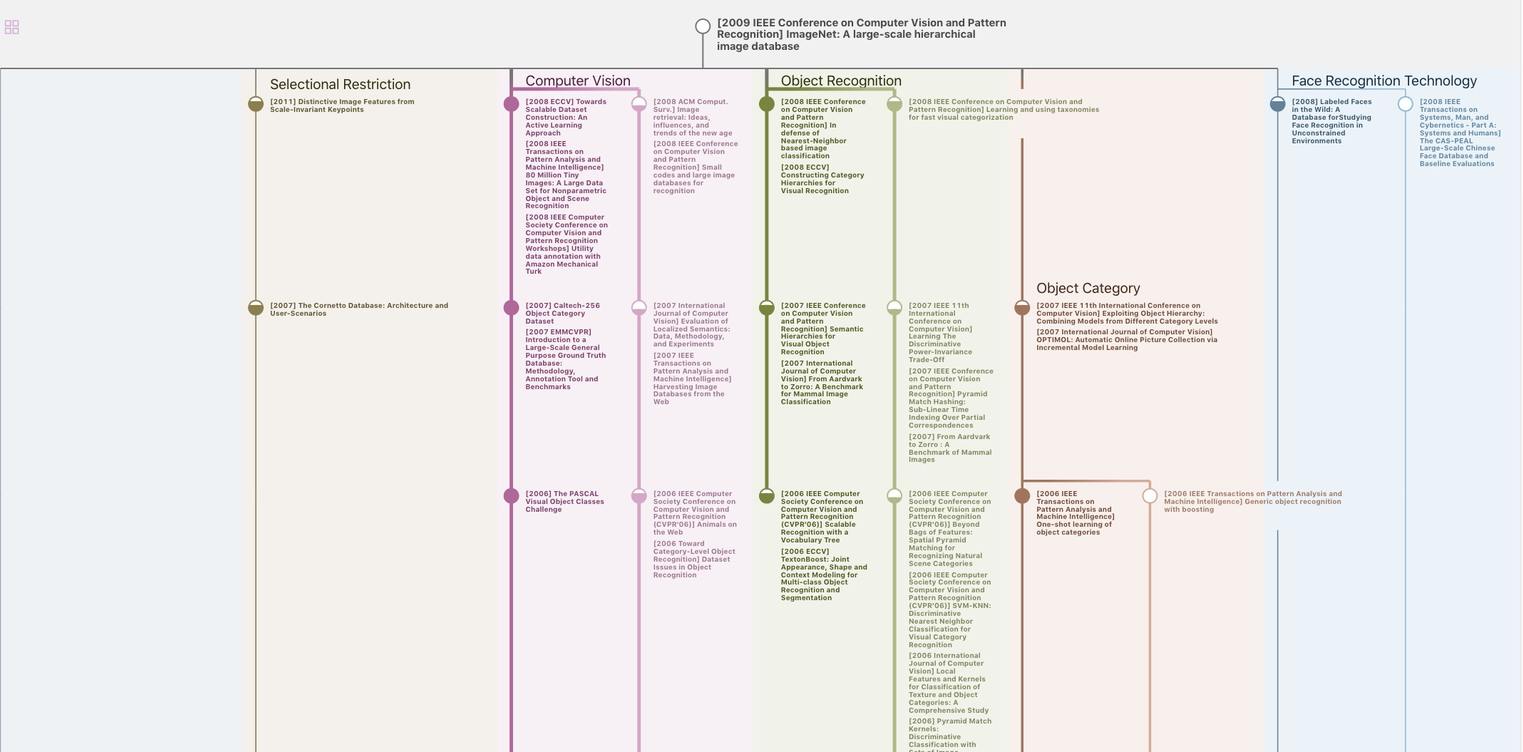
生成溯源树,研究论文发展脉络
Chat Paper
正在生成论文摘要