XAI for Analyzing and Unlearning Spurious Correlations in ImageNet
semanticscholar(2020)
摘要
Contemporary learning models for computer vision are typically trained on very large data sets with millions of samples. There may, however, be biases, artifacts, or errors in the data that have gone unnoticed and are exploitable by the model, which in turn becomes a biased ‘Clever-Hans‘ predictor. In this paper, we contribute by providing a comprehensive analysis framework based on a scalable statistical analysis of attributions from explanation methods for large data corpora, here ImageNet. Based on Spectral Relevance Analysis we propose the following technical contributions and resulting findings: (a) a scalable quantification of artifactual classes where the ML models under study exhibit Clever-Hans behavior, (b) an approach denoted as Class-Artifact Compensation (ClArC) that allows to fine-tune an existing model to effectively eliminate its focus on artifacts and biases yielding significantly reduced Clever-Hans behavior.
更多查看译文
AI 理解论文
溯源树
样例
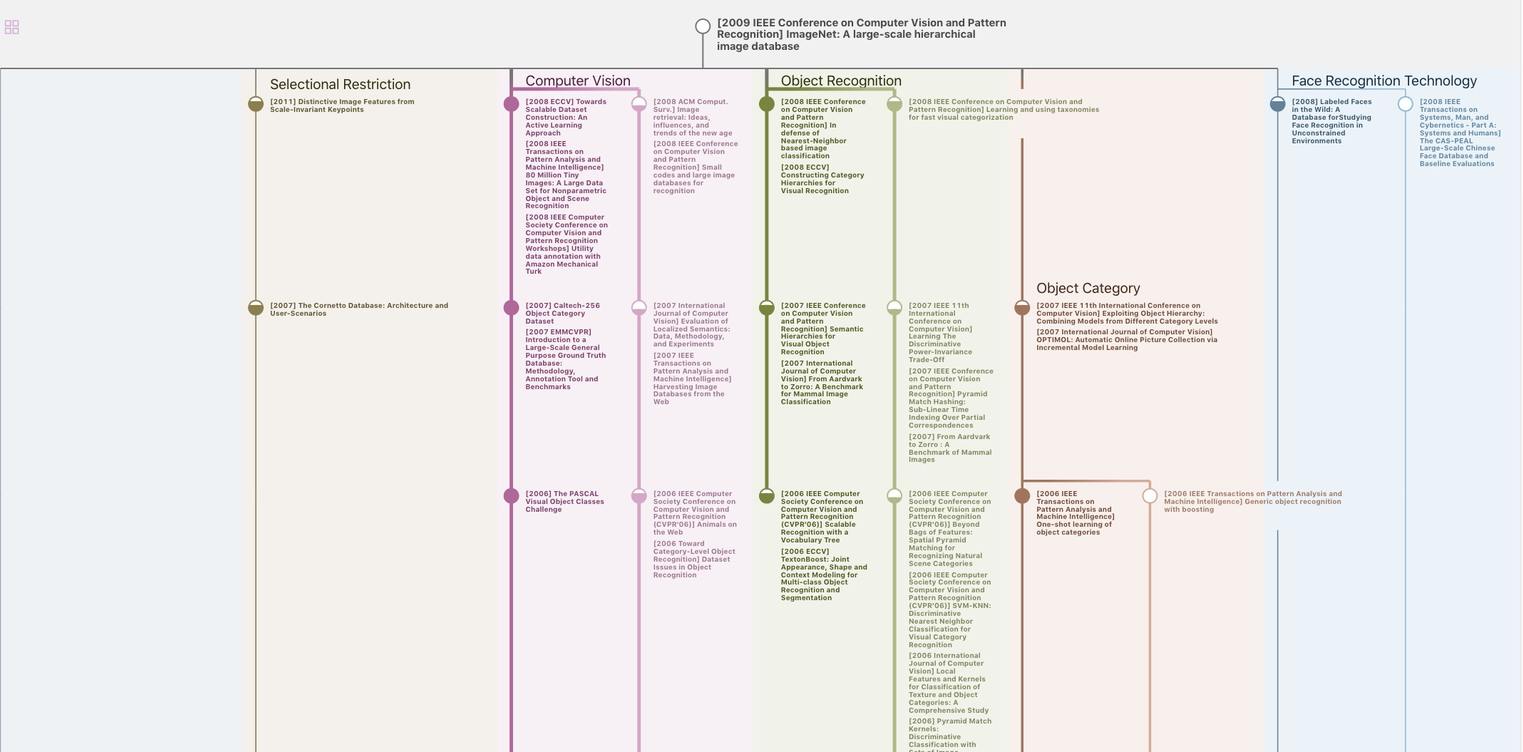
生成溯源树,研究论文发展脉络
Chat Paper
正在生成论文摘要