Expectations and the empirical fit of DSGE models
semanticscholar(2018)
摘要
This paper studies the improvement in empirical fit of dynamic stochastic general equilibrium (DSGE) models that assume adaptive learning in lieu of rational expectations (RE). The literature finds that estimated DSGE models with adaptive learning generate near universal improvements in fit, while inference on structural parameters is mostly unchanged. Improvements are attributed to the increased persistence generated by backward-looking expectations. We show, however, that improvements often result from altered cross-equation restrictions and not additional persistence assumptions. Nested comparisons of Euler-equation and infinitehorizon adaptive learning both significantly improve upon RE but only the latter’s improvements are due to expectation formation. Bounded rationality assumptions offer an intuitive way to improve both in-sample and out-of-sample DSGE model fit. But our results suggest that learning models best-capture persistent deviation in beliefs from fundamentals rather than temporary deviations at business cycle frequencies. JEL Classifications: E31; E32; D84; D83; C13
更多查看译文
AI 理解论文
溯源树
样例
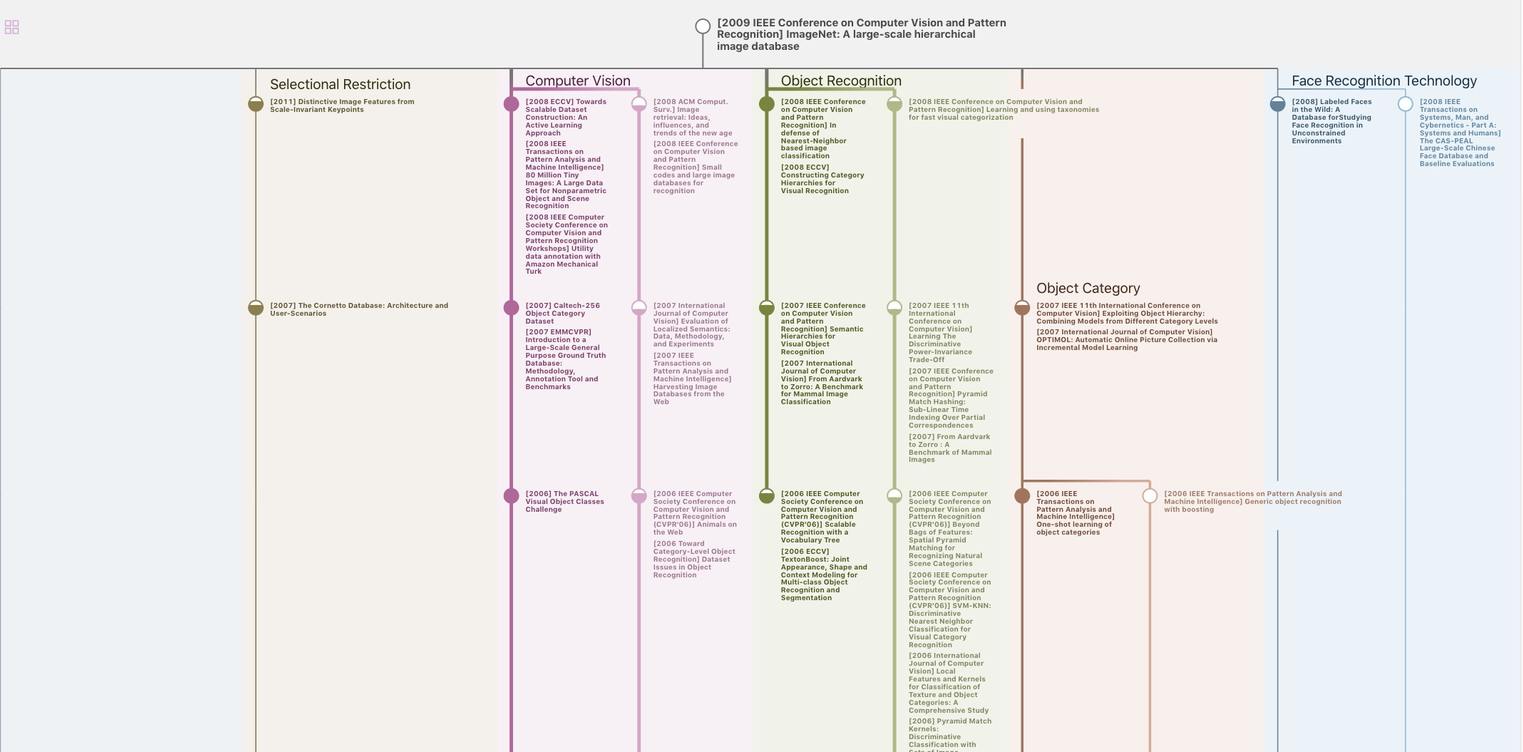
生成溯源树,研究论文发展脉络
Chat Paper
正在生成论文摘要