Sequential Randomization to Develop Personalized and Optimized Interventions in Massively Open Online Courses: A Case Study
semanticscholar(2018)
摘要
In typical experiments, each subject is randomized one time; once a subject has been assigned to a particular treatment option, they remain in that same treatment throughout the rest of the trial. In contrast, sequentially randomized trials are experiments in which a subject is randomized several times to different treatment options throughout the experiment. Sequential randomization is beneficial over one-time randomization because it informs the design of personalized and optimized interventions. Firstly, by rerandomizing, subjects receive a variety of intervention sequences (e.g., treatment A then treatment B or treatment B then treatment A). These various sequences can be compared to discover the optimal intervention sequence. Secondly, instead of only being able to assess the overall effect of getting one particular treatment, sequential randomization lets researchers discover effects at smaller time-scales (e.g., treatment A does better in week 2 of the course, but treatment B does better in week 3 of the course). These discoveries inform at what time points certain interventions are most effective. Thirdly, rerandomization permits the discovery of important variables measured throughout the trial for adapting and personalizing an intervention. As opposed to only being able to discover pre-trial personalization variables (e.g., treatment A works better for women), we can also discover mid-trial personalization variables (treatment A works better for subjects with a large amount of missing data streams).
更多查看译文
AI 理解论文
溯源树
样例
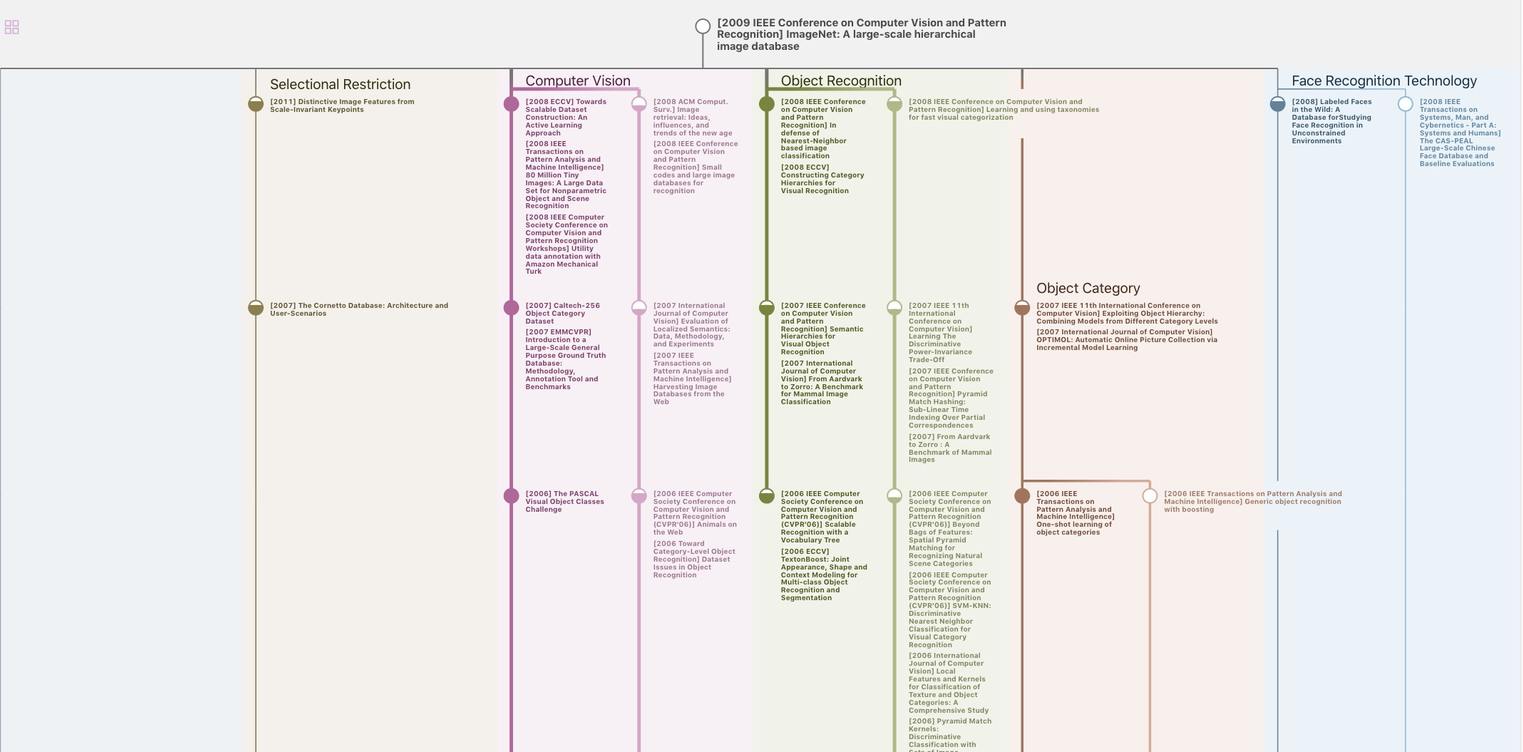
生成溯源树,研究论文发展脉络
Chat Paper
正在生成论文摘要