Uncertainty Quantification of a Classification Network for Contact-Rich Manipulation
semanticscholar(2020)
摘要
This project is an extension of a project that is being developed in the Interactive Perception and Robotic Learning lab at Stanford. Most robots in industry today can only accomplish tasks with little to no uncertainty. The aim of the original project is to develop a method which extends the set of tasks which robots can accomplish using deep learning methods to include tasks with certain forms of options uncertainty. An example of options uncertainty is selecting a tool for a task. For example, when you have to change your tire and have to choose between a set of hex wrenches. The options uncertainty is over which tool to select. For this project, we will be using a 7 DOF robotic arm in simulation to accomplish the task of peg insertion with multiple hole options for the robot to choose from. The robot will need to interact with the peg holes and quantify its uncertainty for each peg hole over the hole’s shape using only force and position data. For our CS230 project we looked into different training objectives and network architectures for quantifying uncertainty of a discrete probability distribution. This should improve the performance of the method we are developing for the original research project.
更多查看译文
AI 理解论文
溯源树
样例
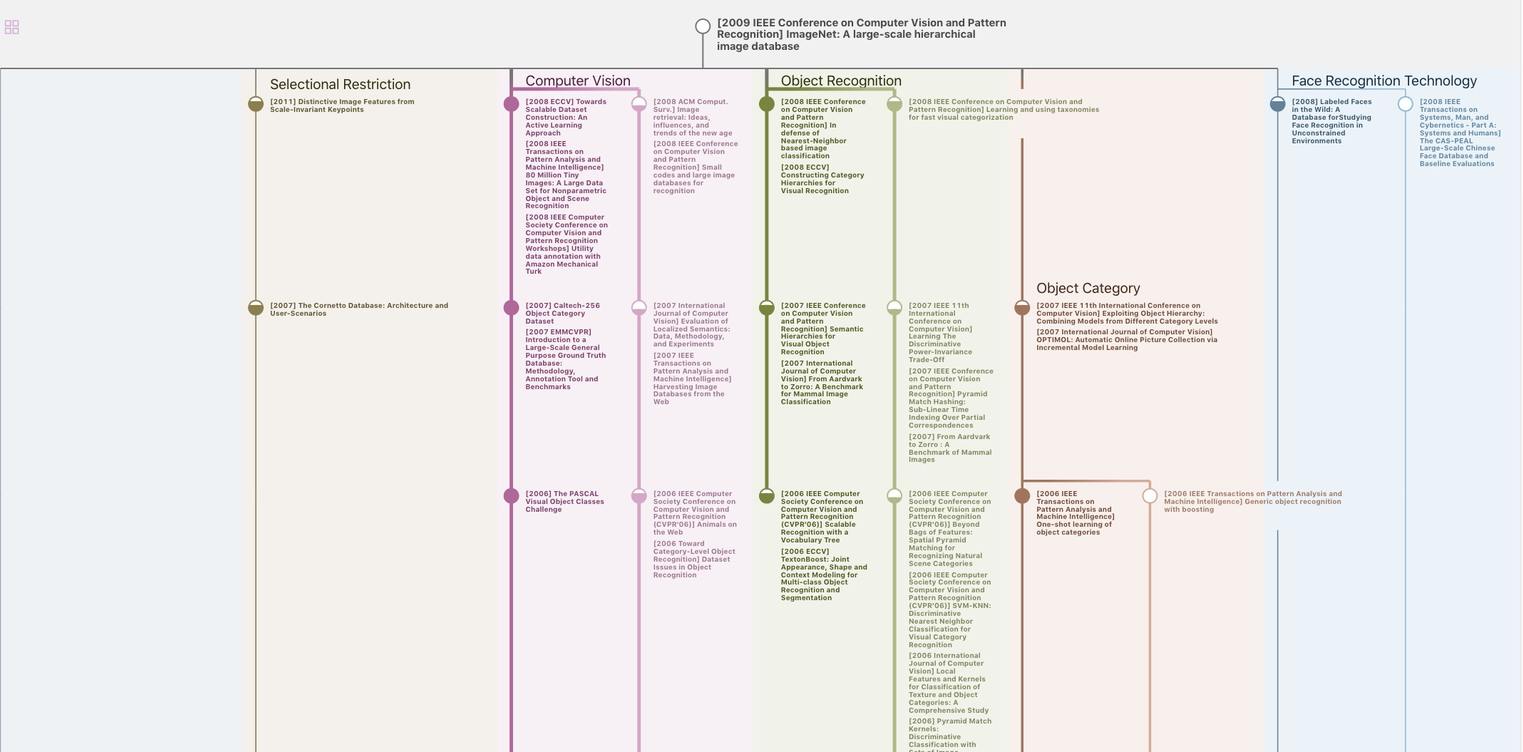
生成溯源树,研究论文发展脉络
Chat Paper
正在生成论文摘要