1 Convolutional Neural Network and Long Short-Term Memory 1 Models for Ice-Jam Prediction 2 3
semanticscholar(2021)
摘要
Convolutional Neural Network and Long Short-Term Memory 1 Models for Ice-Jam Prediction 2 3 Fatemehalsadat Madaeni, Karem Chokmani, Rachid Lhissou, Saied Homayouni, Yves 4 Gauthier, and Simon Tolszczuk-Leclerc 5 INRS-ETE, Université du Québec, Québec City, G1K 9A9, Canada 6 EMGeo Operations, Natural Resources Canada, Ottawa, K1S 5K2, Canada 7 Correspondence to: Fatemehalsadat Madaeni (Fatemehalsadat.Madaeni@ete.inrs.ca) 8 9 Abstract. In cold regions, ice-jam events result in severe flooding due to a rapid rise in water levels upstream of the 10 jam. These floods threaten human safety and damage properties and infrastructures as the floods resulting from ice11 jams are sudden. Hence, the ice-jam prediction tools can give an early warning to increase response time and minimize 12 the possible corresponding damages. However, the ice-jam prediction has always been a challenging problem as there 13 is no analytical method available for this purpose. Nonetheless, ice jams form when some hydro-meteorological 14 conditions happen, a few hours to a few days before the event. The ice-jam prediction problem can be considered as 15 a binary multivariate time-series classification. Deep learning techniques have been successfully applied for time16 series classification in many fields such as finance, engineering, weather forecasting, and medicine. In this research, 17 we successfully applied CNN, LSTM, and combined CN-LSTM networks for ice-jam prediction for all the rivers in 18 Quebec. The results show that the CN-LSTM model yields the best results in the validation and generalization with 19 F1 scores of 0.82 and 0.91, respectively. This demonstrates that CNN and LSTM models are complementary, and a 20 combination of them further improves classification. 21
更多查看译文
AI 理解论文
溯源树
样例
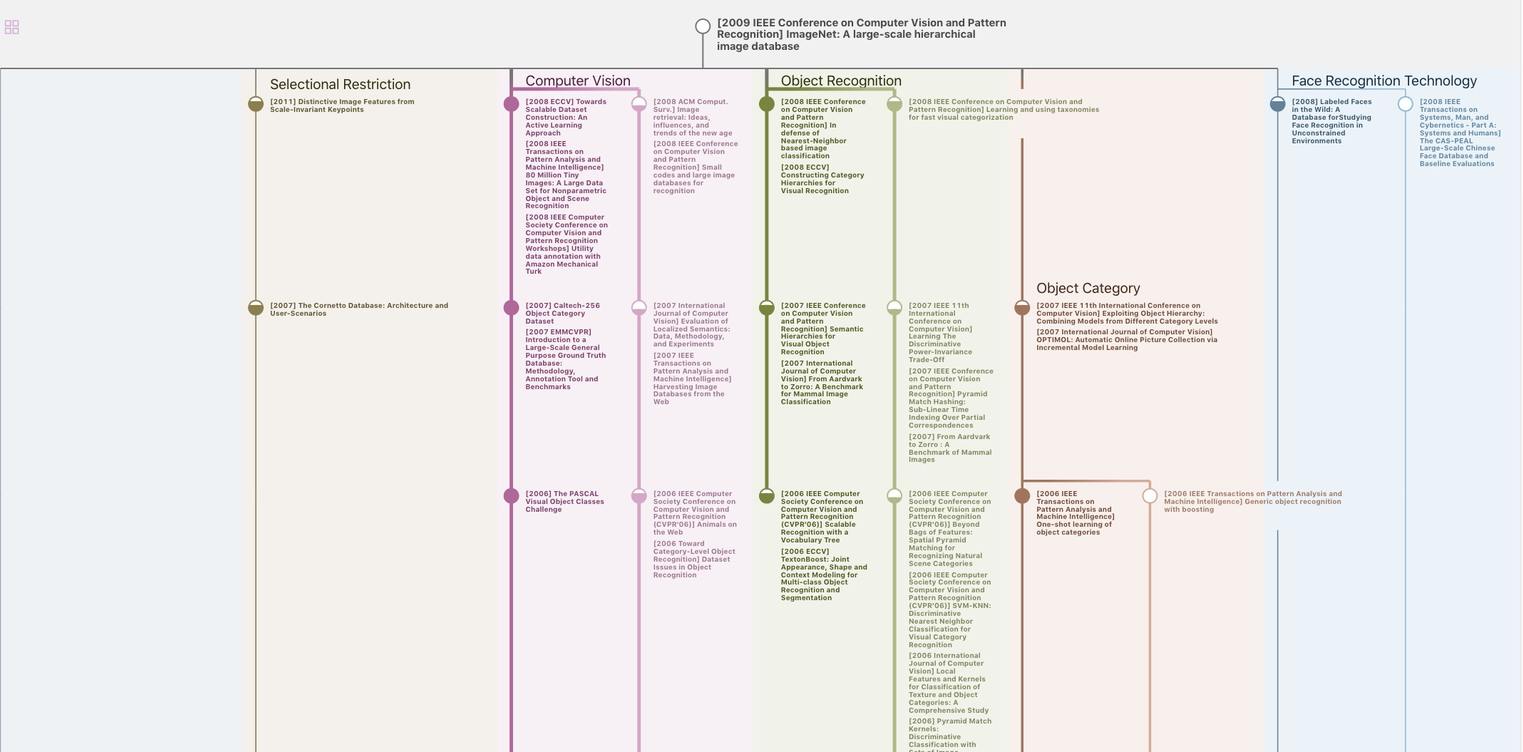
生成溯源树,研究论文发展脉络
Chat Paper
正在生成论文摘要