Fine-Grained Localization, Classification and Segmentation of Lungs with Various Diseases
semanticscholar(2021)
摘要
The fine-grained localization and classification of various lung abnormalities is a challenging yet important task for combating diseases and, also, pandemics. In this paper, we present one way to detect and classify abnormalities within chest X-ray scans. In particular, we investigate the use of binary image classification (to distinguish between healthy and infected chests) and the weighted box fusion (which constructs a detection box using the proposed boxes within range). We observe that both methods increase the performance of a base model significantly. Furthermore, we improve state of the art on lung segmentation, even in the presence of abnormalities. We do so using transfer learning to fine-tune a UNet model on the Montgomery and Shenzhen datasets. In our experiments, we compare standard augmentations (like crop, pad, rotate, warp, zoom, brightness, and contrast variations) to more complex ones (for example, block masking and diffused noise augmentations). This way, we obtain a state-of-the-art model with a dice score of 97.9%. In particular, we show that simple augmentations outperform complex ones in our setting.
更多查看译文
AI 理解论文
溯源树
样例
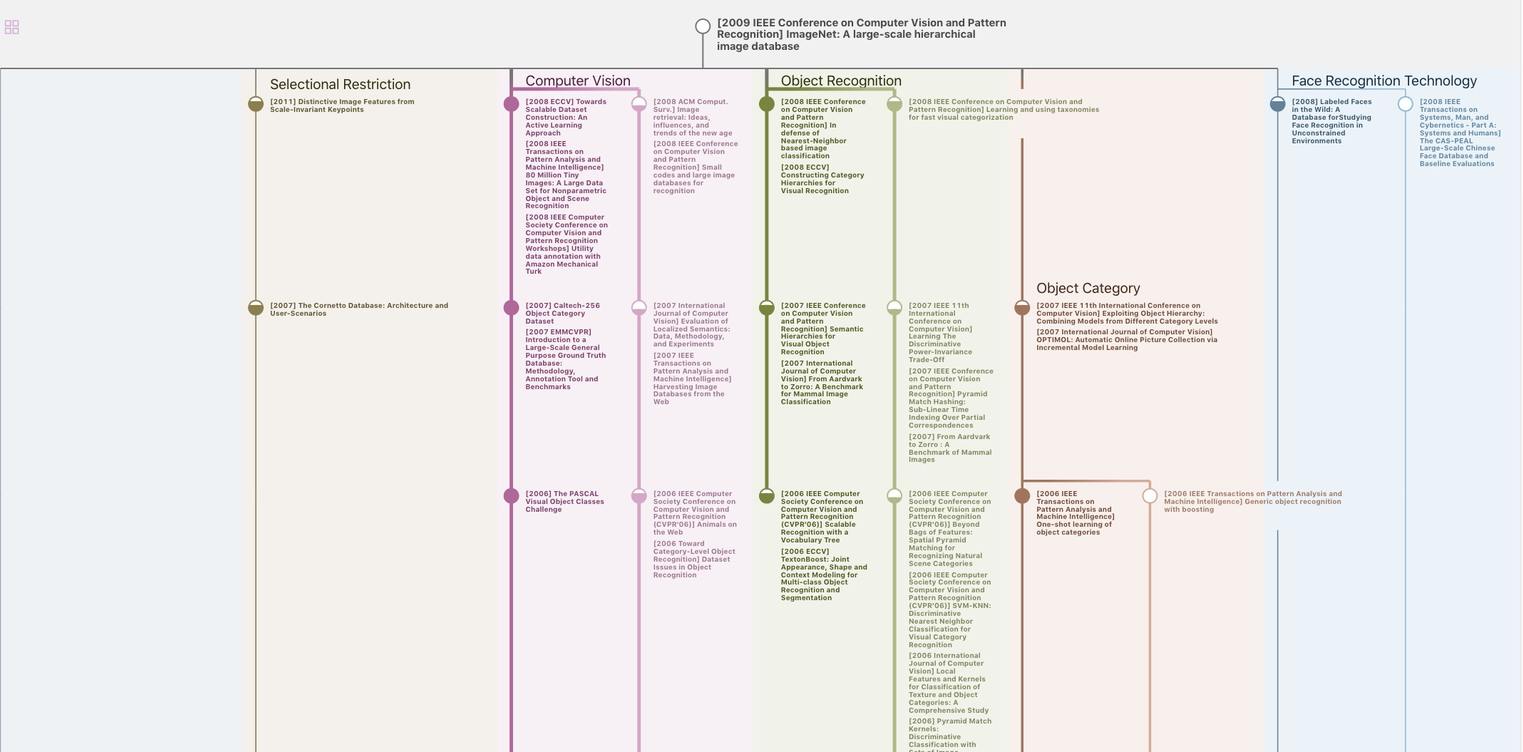
生成溯源树,研究论文发展脉络
Chat Paper
正在生成论文摘要