Profile Inference Revisited
ACM-SIGACT Symposium on Principles of Programming Languages(2022)
摘要
Profile-guided optimization (PGO) is an important component in modern compilers. By allowing the compiler to leverage the program's dynamic behavior, it can often generate substantially faster binaries. Samplingbased profiling is the state-of-the-art technique for collecting execution profiles in data-center environments. However, the lowered profile accuracy caused by sampling fully optimized binary often hurts the benefits of PGO; thus, an important problem is to overcome the inaccuracy in a profile after it is collected. In this paper we tackle the problem, which is also known as profile inference and profile rectification. We investigate the classical approach for profile inference, based on computing minimum-cost maximum flows in a control-flow graph, and develop an extended model capturing the desired properties of real-world profiles. Next we provide a solid theoretical foundation of the corresponding optimization problem by studying its algorithmic aspects. We then describe a new efficient algorithm for the problem along with its implementation in an open-source compiler. An extensive evaluation of the algorithm and existing profile inference techniques on a variety of applications, including Facebook production workloads and SPEC CPU benchmarks, indicates that the new method outperforms its competitors by significantly improving the accuracy of profile data and the performance of generated binaries.
更多查看译文
关键词
compilers, profile-guided optimizations, network flows
AI 理解论文
溯源树
样例
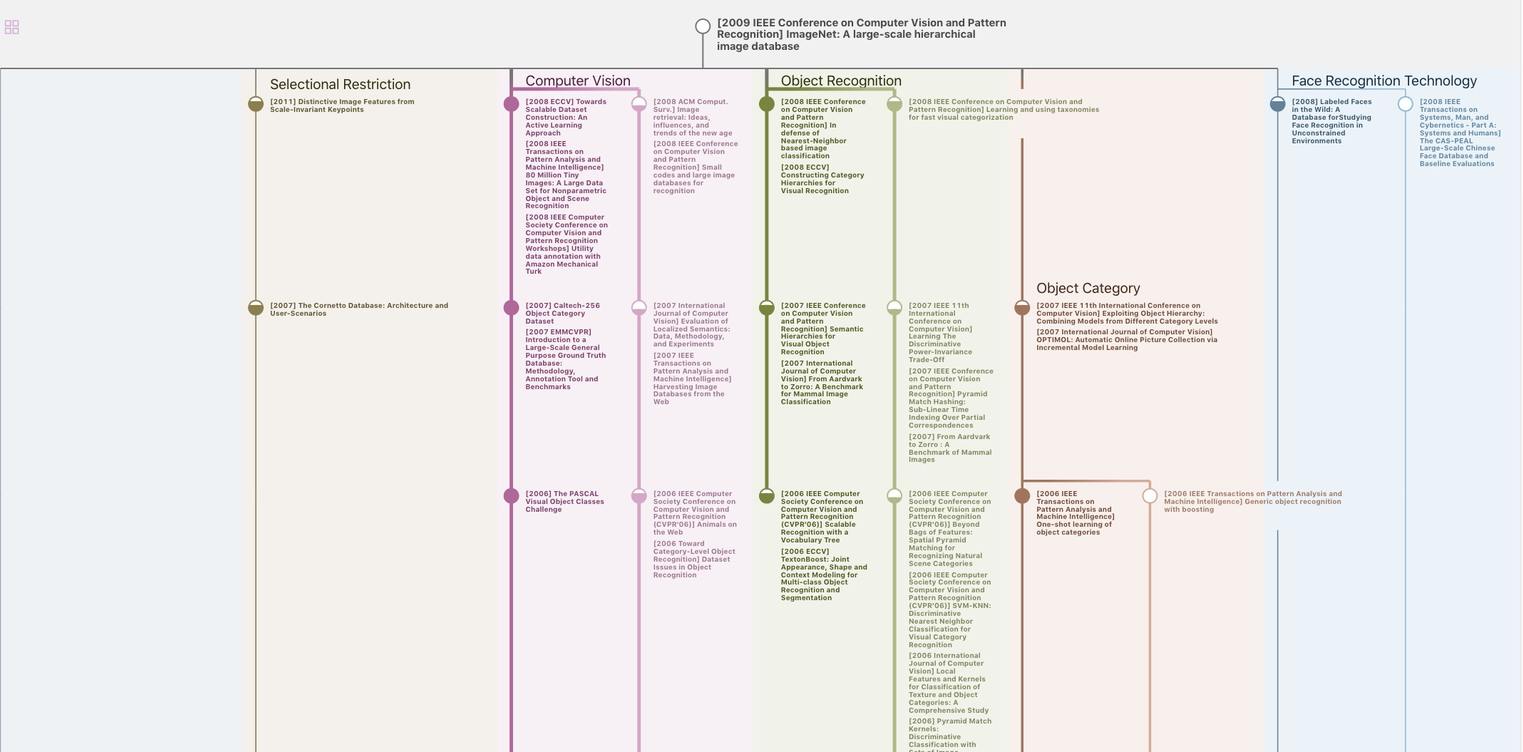
生成溯源树,研究论文发展脉络
Chat Paper
正在生成论文摘要