Using Machine Learning to Predict Mortality for COVID-19 Patients on Day Zero in the ICU
medRxiv(2021)
摘要
Rationale Given the expanding number of COVID-19 cases and the potential for upcoming waves of infection, there is an urgent need for early prediction of the severity of the disease in intensive care unit (ICU) patients to optimize treatment strategies. Objectives Early prediction of mortality using machine learning based on typical laboratory results and clinical data registered on the day of ICU admission. Methods We studied retrospectively 263 COVID-19 ICU patients. To find parameters with the highest predictive values, Kolmogorov-Smirnov and Pearson chi-squared tests were used. Logistic regression and random forest (RF) algorithms were utilized to build classification models. The impact of each marker on the RF model predictions was studied by implementing the local interpretable model-agnostic explanation technique (LIME-SP). Results Among 66 documented parameters, 15 factors with the highest predictive values were identified as follows: gender, age, blood urea nitrogen (BUN), creatinine, international normalized ratio (INR), albumin, mean corpuscular volume, white blood cell count, segmented neutrophil count, lymphocyte count, red cell distribution width (RDW), and mean cell hemoglobin along with a history of neurological, cardiovascular, and respiratory disorders. Our RF model can predict patients outcomes with a sensitivity of 70% and a specificity of 75%. Conclusions The most decisive variables in our model were increased levels of BUN, lowered albumin levels, increased creatinine, INR, and RDW along with gender and age. Complete blood count parameters were also crucial for some patients. Considering the importance of early triage decisions, this model can be a useful tool in COVID-19 ICU decision-making.
更多查看译文
关键词
mortality,icu,machine learning,day zero
AI 理解论文
溯源树
样例
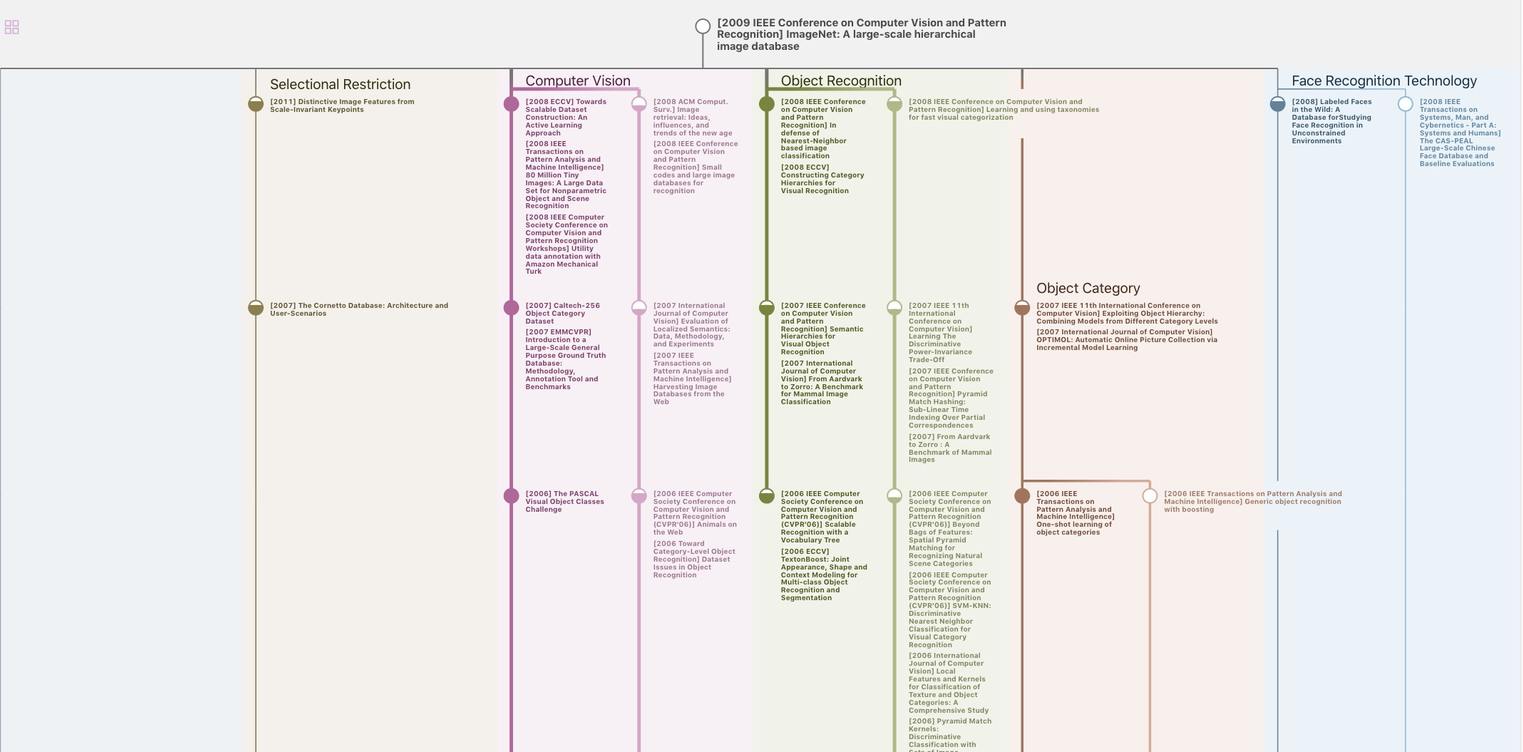
生成溯源树,研究论文发展脉络
Chat Paper
正在生成论文摘要