Representing Pareto optima in preordered spaces: from Shannon entropy to injective monotones
ArXiv(2021)
摘要
Shannon entropy is the most widely used measure of uncertainty. It is used, for example, in Jaynes’ maximum entropy principle which is considered a basis for statistical inference and serves as a justification for many regularization techniques that appear throughout machine learning and decision theory. Entropy is, however, only one possible monotone that does not fully capture the more fundamental notion of uncertainty considered as a preorder on the space of probability distributions, also known as majorization. While the maximum entropy principle therefore cannot yield all Pareto optima of the uncertainty preorder in general, it has the appealing property that its solutions are unique Pareto optima, since it maximizes a strictly concave functional over a convex subset. Here, we investigate a class of monotones on general preordered spaces that preserve this uniqueness property (up to order equivalence) on any subset, without asking for the additional vector space structure required for convexity. We show that the class of preorders for which these so-called injective monotones exist, lies in between the class of preorders with strict monotones and preorders with utility functions. We extend several well-known results for strict monotones (Richter-Peleg functions) to injective monotones, we provide a construction of injective monotones from countable multi-utilities, and relate injective monotones to classic results concerning Debreu denseness and order separability. Along the way, we connect our results to Shannon entropy and the uncertainty preorder, obtaining new insights into how they are related.
更多查看译文
关键词
pareto optima,shannon entropy,spaces
AI 理解论文
溯源树
样例
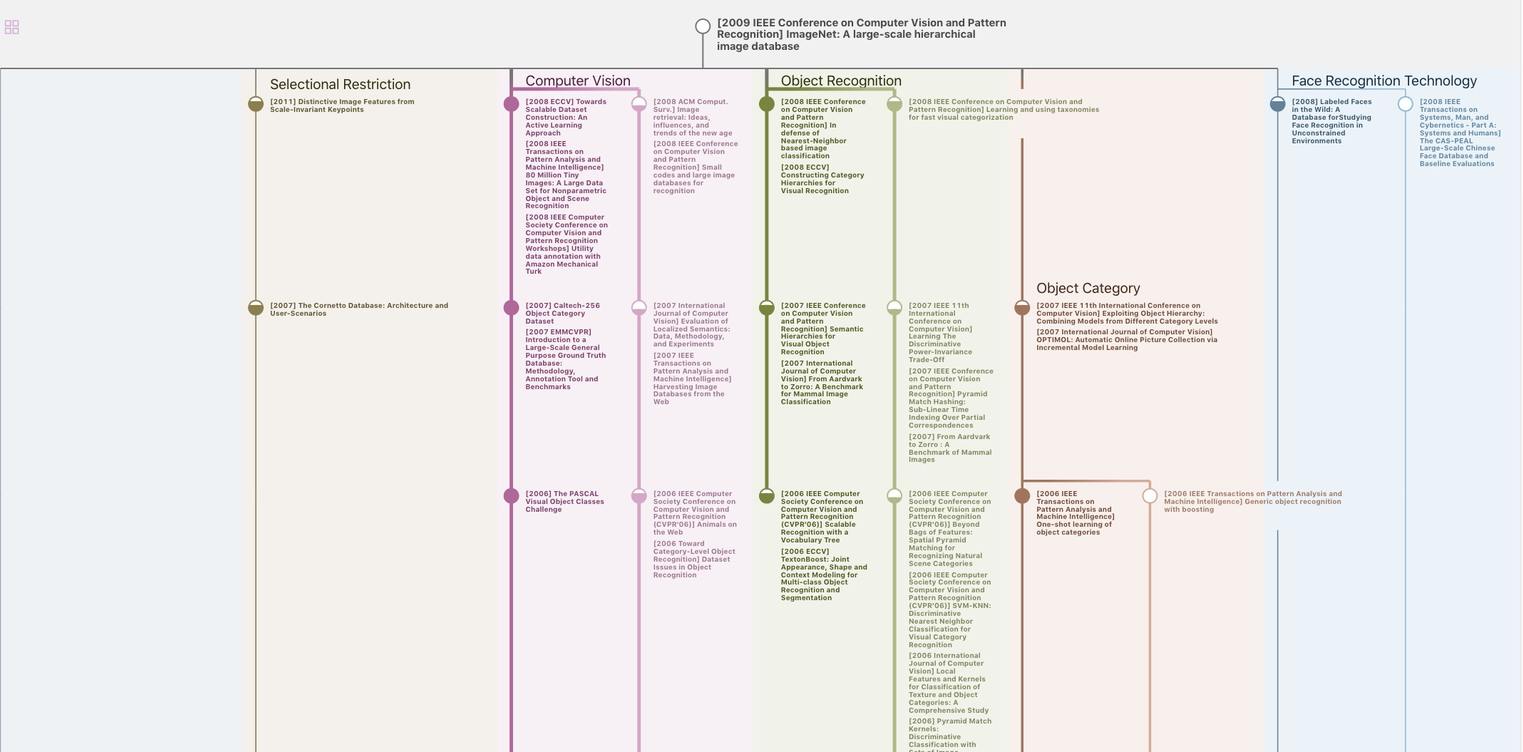
生成溯源树,研究论文发展脉络
Chat Paper
正在生成论文摘要