Learning non-linear spatio-temporal dynamics with convolutional Neural ODEs
semanticscholar(2020)
摘要
Advances in both scientific computing and machine learning have led to techniques to improve, replace, or speed up methods to solve problems in the physical sciences. For many nonlinear systems, traditional methods of calculation often carry a heavy computational burden due to complex dynamics, physical constraints, or multi-scale behavior. We introduce a machine learning approach to vastly decrease computational complexity of modeling spatiotemporal systems, leveraging prior physical knowledge of the system with flexible reduced representations of the underlying dynamics. We demonstrate this technique on three-dimensional turbulent fluid flow, wherein the modeling of such systems continues to be a formidable problem for engineers and physicists. We merge convolutional layers with a Neural ODE approach to form a differentiable ML architecture to build a system model. Here, we test the applicability of this method to model homogeneous isotropic turbulence, as well as the effect of different architectural elements on turbulence statistics. We find that the largest scales of the flow, which are relevant to engineering applications, are well-approximated and some dynamic characteristics of interest are maintained.
更多查看译文
AI 理解论文
溯源树
样例
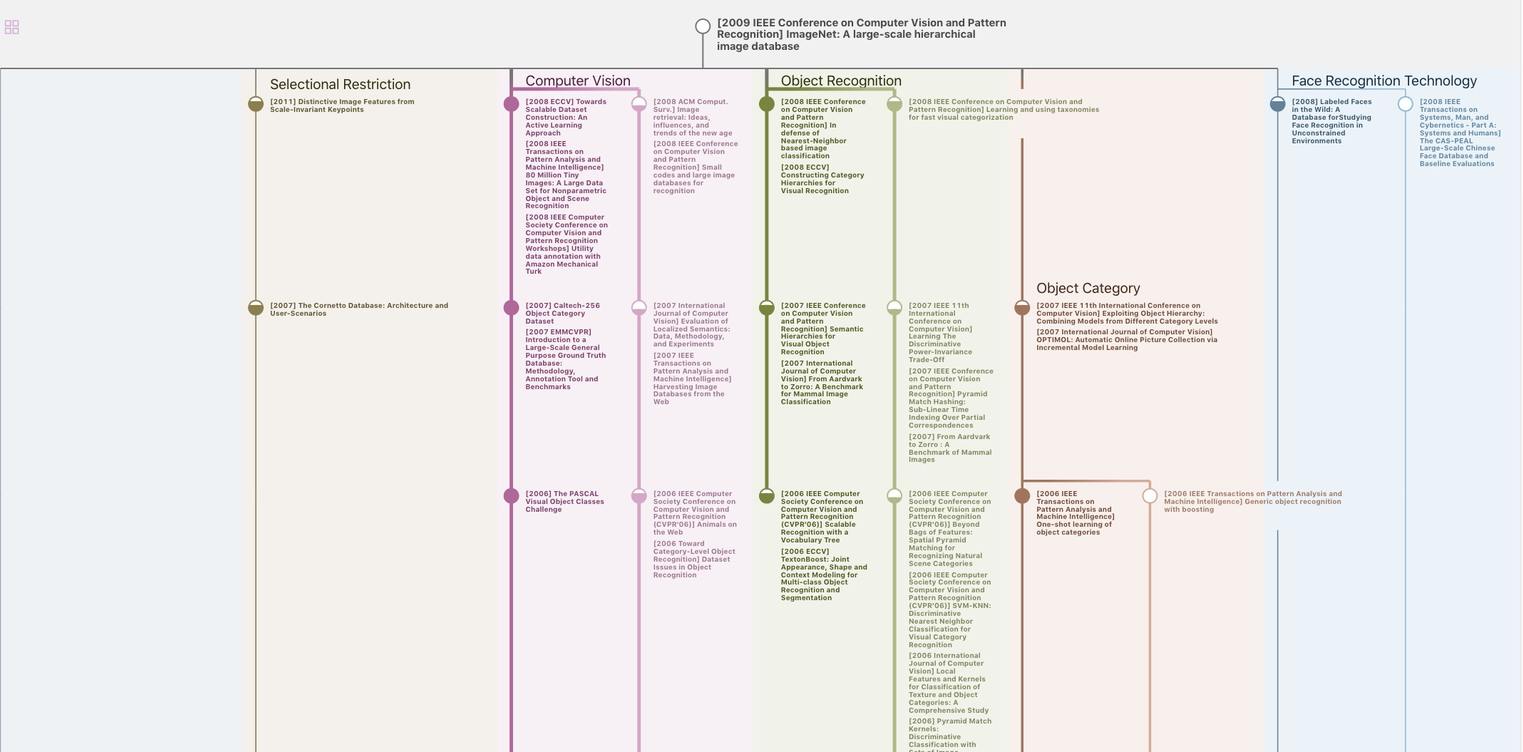
生成溯源树,研究论文发展脉络
Chat Paper
正在生成论文摘要