Meta-Learning of Structured Representation by Proximal Mapping
semanticscholar(2019)
摘要
Underpinning the success of deep learning are the effective regularization techniques that allow a broad range of structures in data to be compactly modeled in a deep architecture. Examples include transformation invariances and correlations between multiple modalities. However, most existing methods incorporate such priors either by auto-encoders, whose result is used to initialize supervised learning, or by augmenting the data with exemplifications of the transformations which, despite the improved performance of supervised learning, leaves it unclear whether the learned latent representation does encode the desired regularities. To address these issues, this work proposes an end-to-end representation learning framework based on meta-learning, which allows prior structures to be encoded explicitly in the hidden layers, and to be trained efficiently in conjunction with the supervised learning task. It extends meta-learning to unsupervised base learners. The resulting technique is applied to generalize dropout and invariant kernel warping, and to develop novel algorithms for multiview modeling and robust temporal learning.
更多查看译文
AI 理解论文
溯源树
样例
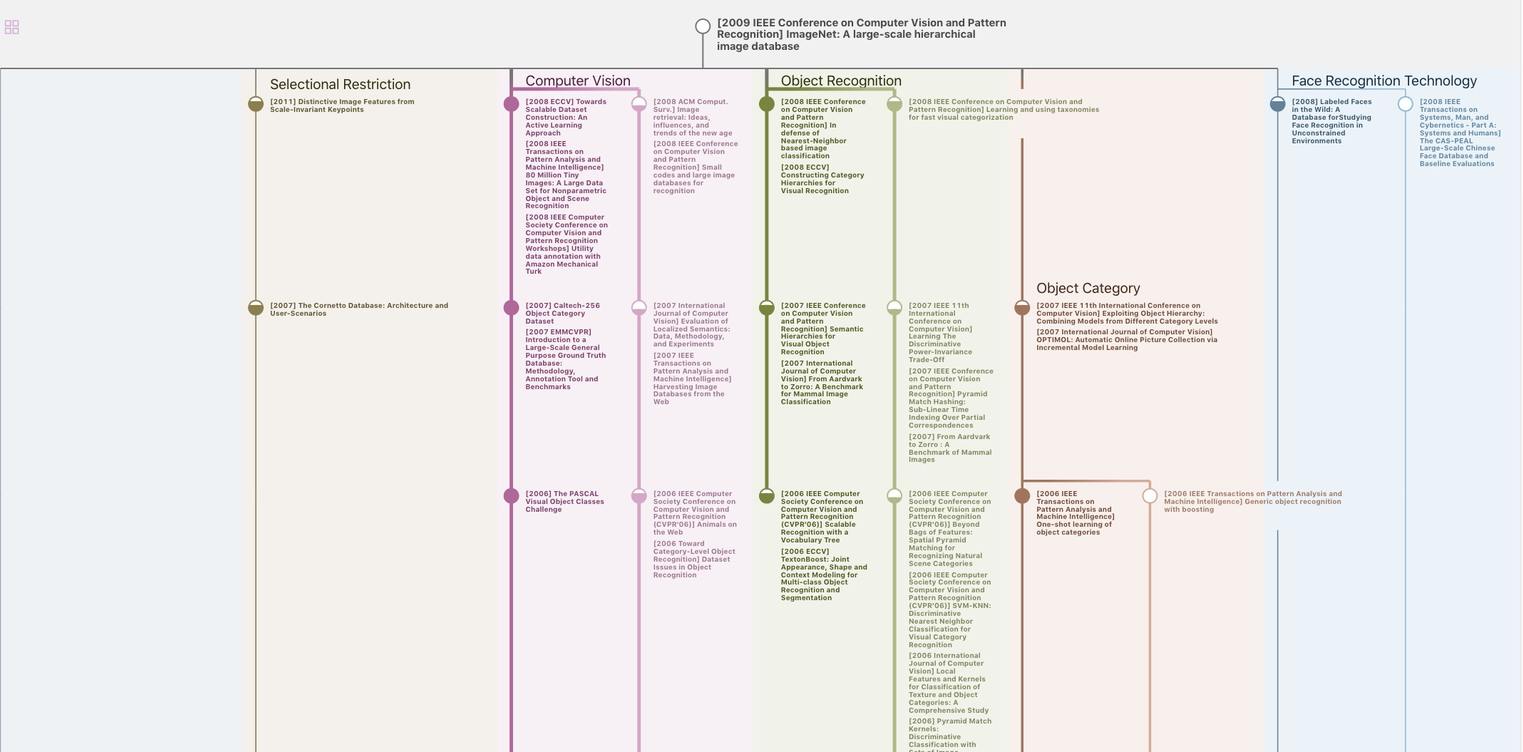
生成溯源树,研究论文发展脉络
Chat Paper
正在生成论文摘要