Reinforcement Learning Contextual Bandits
semanticscholar(2021)
摘要
This note is an introduction to contextual bandits, a class of multi-armed bandits where an agent must take sequential actions at time t = 1, 2, . . . based on observed rewards that are supposed to depend on some unknown parameter θ (the context). In recommander systems for instance, the parameter θ is supposed to characterize the user. This parameter is learnt based on the feedback provided by the user for each proposed item. Each item corresponds to an action of the agent, who must learn the best actions, i.e., the items providing the best rewards. We mainly focus on so-called linear bandits, where expected rewards are linear functions of the actions, then present the extension to logistic bandits.
更多查看译文
AI 理解论文
溯源树
样例
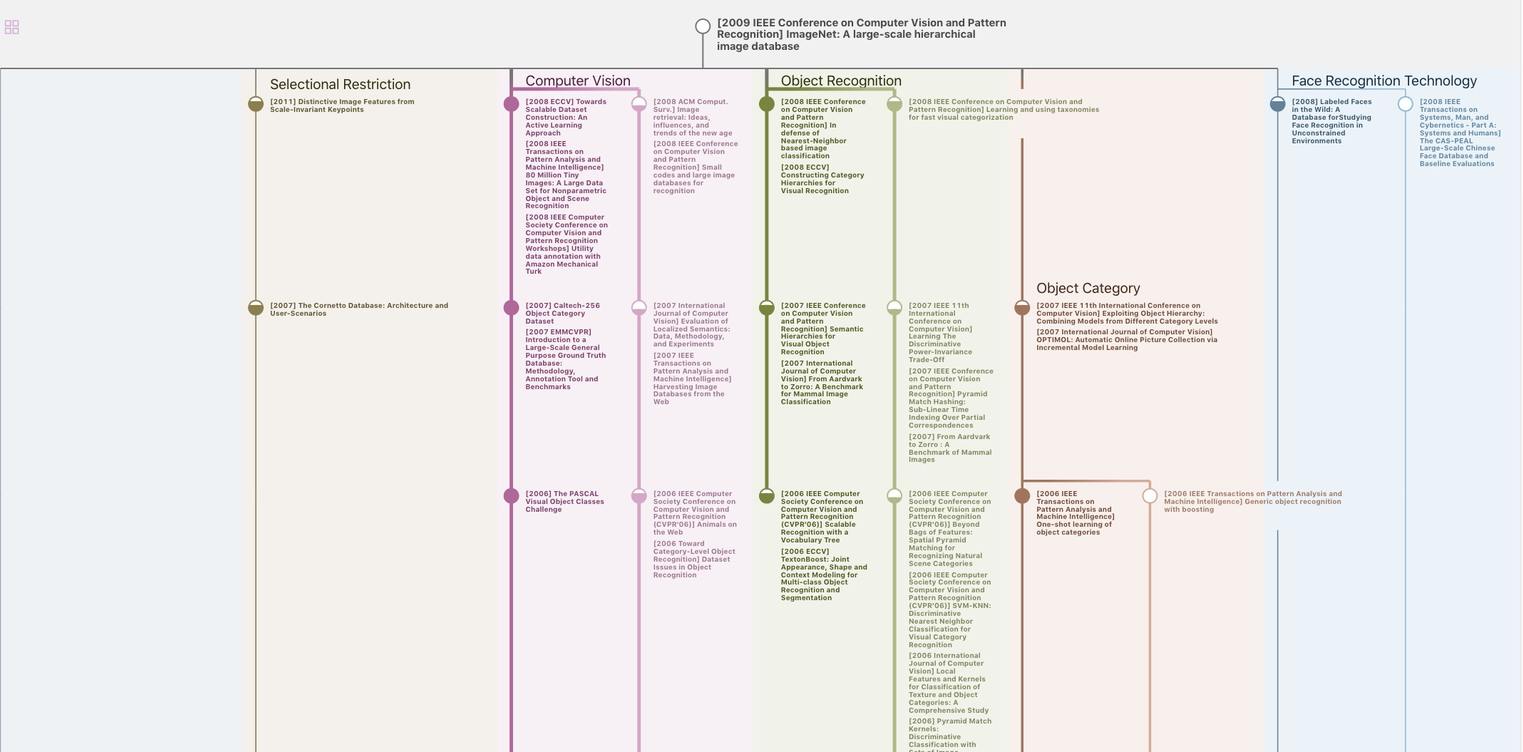
生成溯源树,研究论文发展脉络
Chat Paper
正在生成论文摘要