Towards an articulatory-driven neural vocoder for speech synthesis
HAL (Le Centre pour la Communication Scientifique Directe)(2020)
摘要
High-quality articulatory synthesis is increasingly required for both fundamental objectives, such as better understanding speech production and speech development, and applications that require to relate gestures and sounds, such as teaching, handicap remediation or augmented reality [1]. One possible approach to build such synthesizers is to exploit datasets containing “parallel” articulatory-acoustic data, i.e., speech sounds recorded simultaneously with the movements of the main speech articulators (tongue, lips, jaw, velum) using specific motion capture systems such as electro-magnetic articulography (EMA). The complex and non-linear relationship between the articulatory configuration and the spectral envelope of the corresponding speech sound is learned using supervised machine learning techniques such as Gaussian Mixture Models [2], Hidden Markov Models [3, 4], or Deep Neural Networks (DNN) [5]. The acoustic signal is finally synthetized by deriving an autoregressive filter (e.g., the MLSA vocoder) from the predicted spectral envelope, and exciting this filter with a source signal encoding the glottal activity. We claim that an articulatory synthesizer built following this approach has two main drawbacks. First, its input control parameters (i.e., 2D or 3D coordinates of EMA-coils) are not articulatory parameters per se in the sense that they do not control explicitly the degrees of freedom of the vocal apparatus (i.e., the limited set of movements that each articulator can execute independently from the other articulators). Second, the synthesized speech sounds muffled. This is likely due to the vocoding process and the quality of the excitation signal. This study aims at addressing these two issues. We propose a new approach for building a synthesizer driven by explicit articulatory parameters and able to produce high-quality speech sounds. This work relies on recent developments on so-called neural vocoders. A neural vocoder is a deep autoregressive neural network that synthesizes a sequence of time-domain signal samples. Neural vocoders such as WaveNet or LPCNet [6] have recently led to an impressive gain of performance in text-to-speech synthesis. Here, we propose to drive such a vocoder by a set of articulatory parameters. An overview of the proposed system is shown in Fig 1. The following paragraphs describe the different processing steps.
更多查看译文
关键词
neural vocoder,synthesis,articulatory-driven
AI 理解论文
溯源树
样例
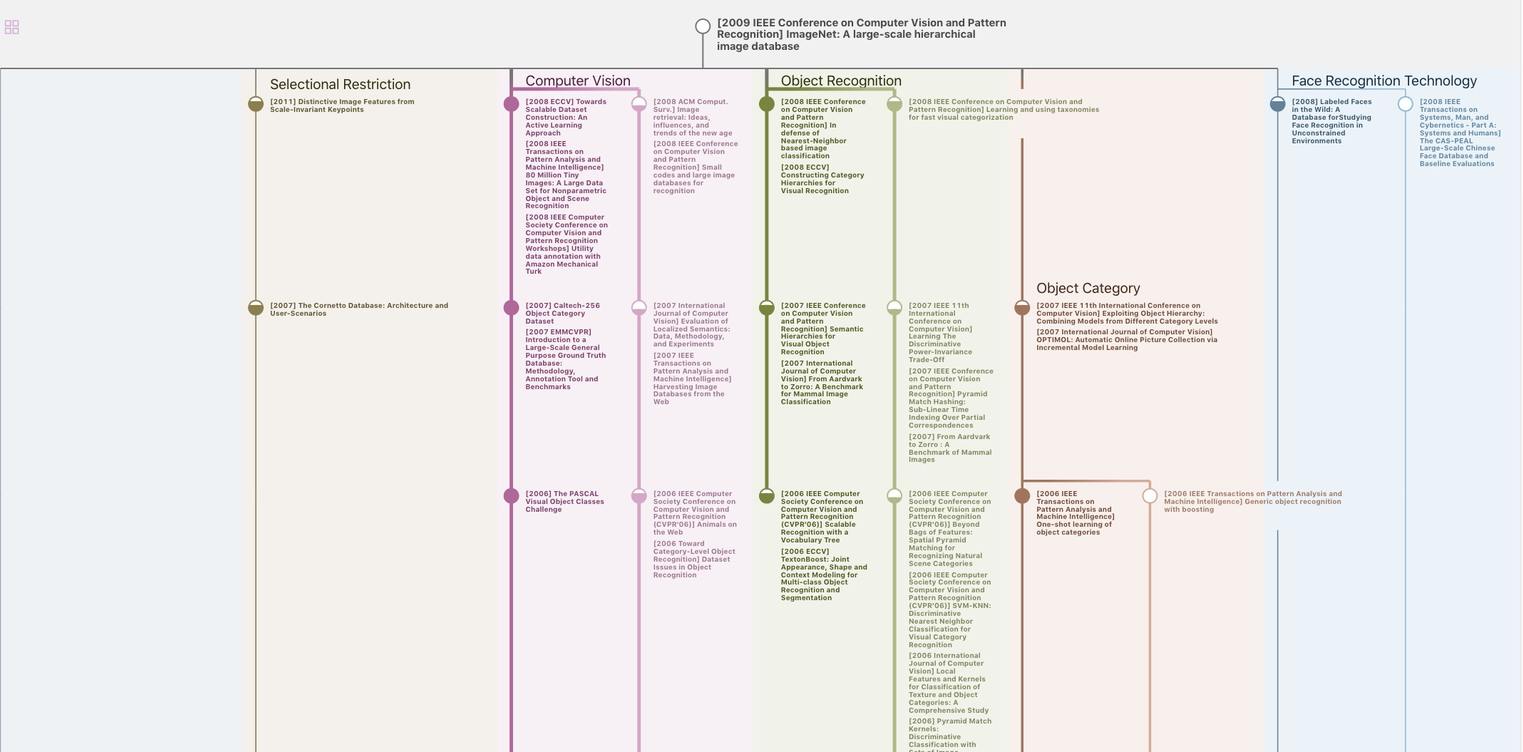
生成溯源树,研究论文发展脉络
Chat Paper
正在生成论文摘要