Online Learning of Feed-Forward Models for Variable Impedance Control in Manipulation Tasks
semanticscholar(2019)
摘要
While performing a new manipulation task, humans tend to be stiffer in the initial trials to improve task accuracy and counter any unforeseen disturbances. After a sufficient number of repetitions, humans are able to perform the task with lower stiffness without causing any significant reduction in task performance. Existing literature in human and animal motor control indicates that learned internal models of the manipulation task and the environment are used to predict the state in response to particular control actions, adapting stiffness on the fly to minimise energy usage. Inspired by these findings, we describe a framework that, in a significant departure from existing work, supports online learning of a time-independent forward model of any given manipulation task in the task space from a small number of samples. Errors in the predictions of the forward model are used to dynamically revise the model and adapt the impedance parameters of the feedback controller. Furthermore, a hybrid force-motion controller supports compliance in certain directions while adapting the stiffness in other directions. We evaluate our framework’s capabilities in the context of continuous-contact manipulation tasks with varying external forces.
更多查看译文
AI 理解论文
溯源树
样例
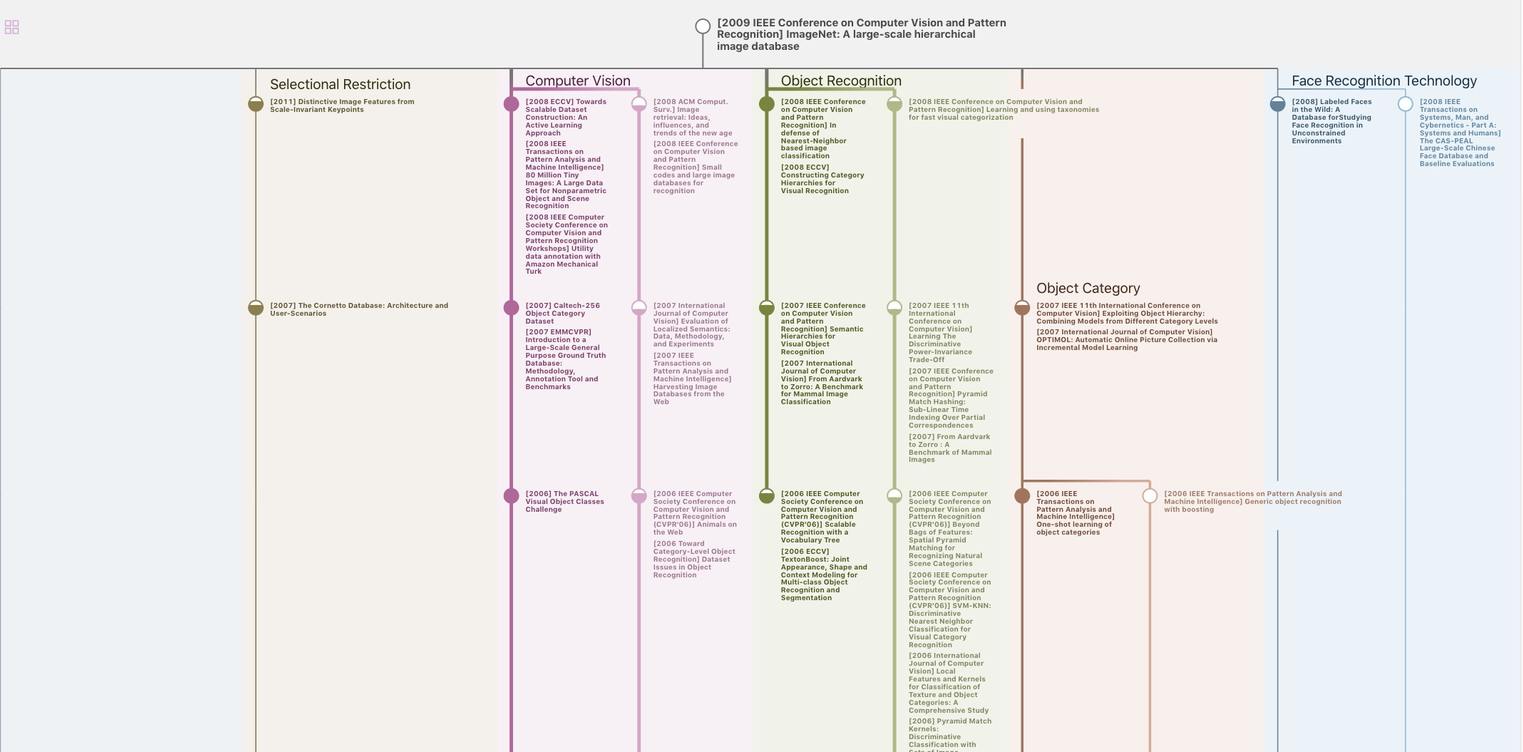
生成溯源树,研究论文发展脉络
Chat Paper
正在生成论文摘要