Deep Learning-Based Point-Scanning Super-Resolution Microscopy
Microscopy and Microanalysis(2021)
摘要
Point-scanning imaging systems (e.g. scanning electron or laser scanning confocal microscopes) are perhaps the most widely used tools for high-resolution cellular and tissue imaging, benefitting from an ability to use arbitrary pixel sizes. Like all other imaging modalities, the resolution, speed, sample preservation, and signal-to-noise ratio (SNR) of point-scanning systems are difficult to optimize simultaneously. In particular, point-scanning systems are uniquely constrained by an inverse relationship between imaging speed and pixel resolution. Here we show these limitations can be mitigated via the use of Deep Learning-based super-sampling of undersampled images acquired on a point-scanning system, which we termed point-scanning super-resolution (PSSR) imaging. To facilitate the generation of large amounts of training data from live cell experiments that are impossible to simultaneously image at low and high resolution, we designed a “crappifier” that computationally degrades high SNR, high pixel resolution ground truth images to simulate low SNR, low-resolution acquisitions. We then used these semi-synthetic training pairs to generate PSSR models that reliably restore real-world undersampled images to pixel resolution and SNR values equivalent to or greater than the ground truth data. Remarkably, our EM PSSR model could restore undersampled images acquired with different optics, detectors, samples, or sample preparation methods in other labs, and PSSR enabled previously unattainable 2nm resolution images with our serial block face scanning electron microscope system. In conclusion, PSSR facilitates point-scanning image acquisition with otherwise unattainable resolution, speed, and sensitivity.
更多查看译文
AI 理解论文
溯源树
样例
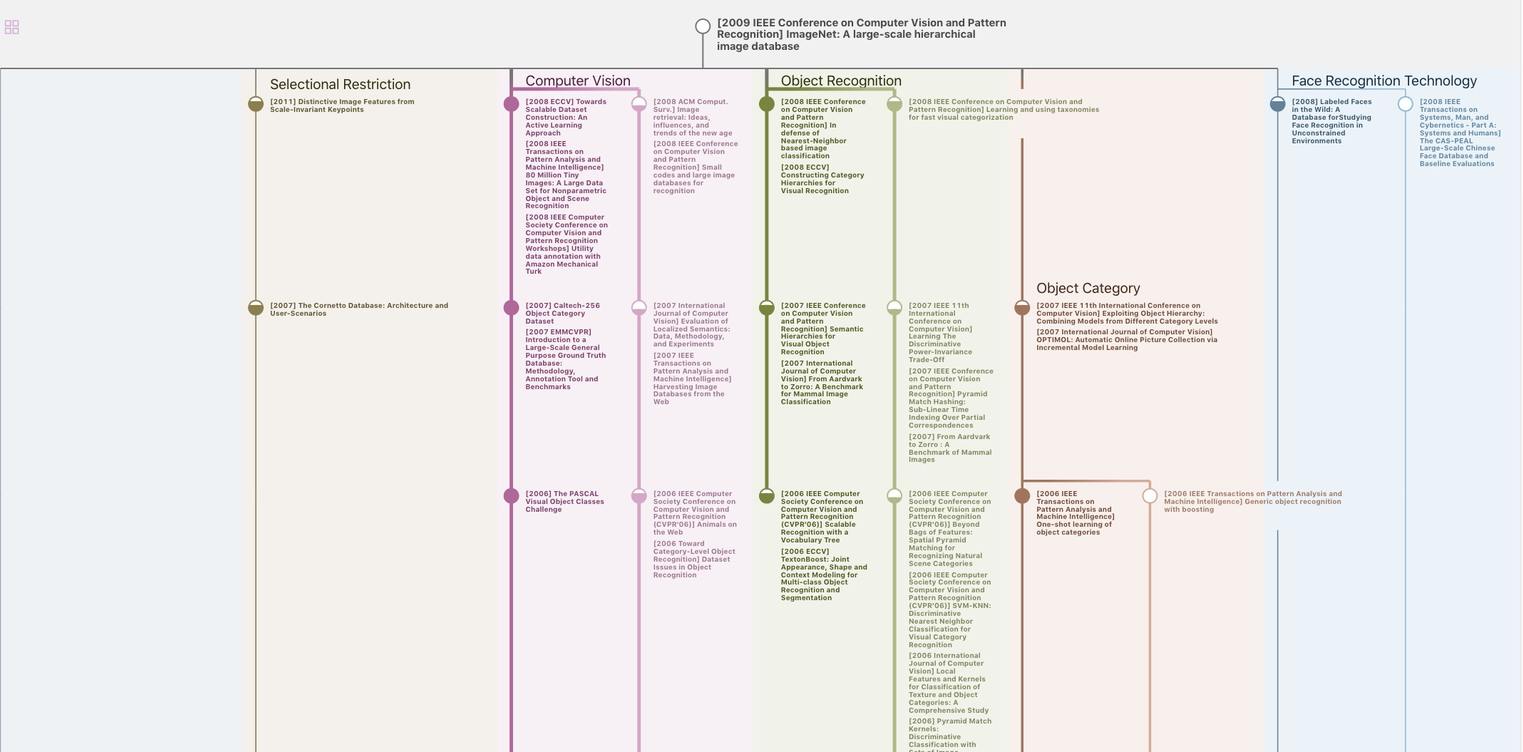
生成溯源树,研究论文发展脉络
Chat Paper
正在生成论文摘要