Biomedical Question Answering
semanticscholar(2020)
摘要
Question Answering and Machine Reading Comprehension (MRC) are crucial and complex tasks in the eld of Natural Language Processing (NLP). In this thesis, we rst introduce BioMRC, a novel biomedical dataset for cloze-type Question Answering, based on previous work of the BioRead dataset, implementing the same baselines and models for comparison. We then develop two new models based on the SciBert model from AllenAI for solving the task of BioMRC. We use these pre-trained models as a transfer learning approach for the BioASQ Task 8B Phase B, in a modi ed architecture, to investigate whether our dataset can be used for improving exact answer Question Answering tasks. In addition, we experiment with other BERT-based models for solving the BioASQ task, which use the SpanBert and BioBert models, as well as the Text-to-Text Transfer Transformer (T5) model, a generative Transformer-based model, which achieved the best results for the task. Moreover, we create a cloze-type version of the BioASQ Task 8B Phase B factoid instances subset, which is used to boost the T5’s results when pre-trained on the BioMRC dataset, but can also be used in future work for automatic transformation of question-answer instances to cloze-type question instances. Lastly, we perform error analysis of our best model for the BioASQ task for exact answers, where we point out the shortcomings of the task evaluation measures and some mistakes, that could be xed by the BioASQ organizers, as an improvement of the task.
更多查看译文
AI 理解论文
溯源树
样例
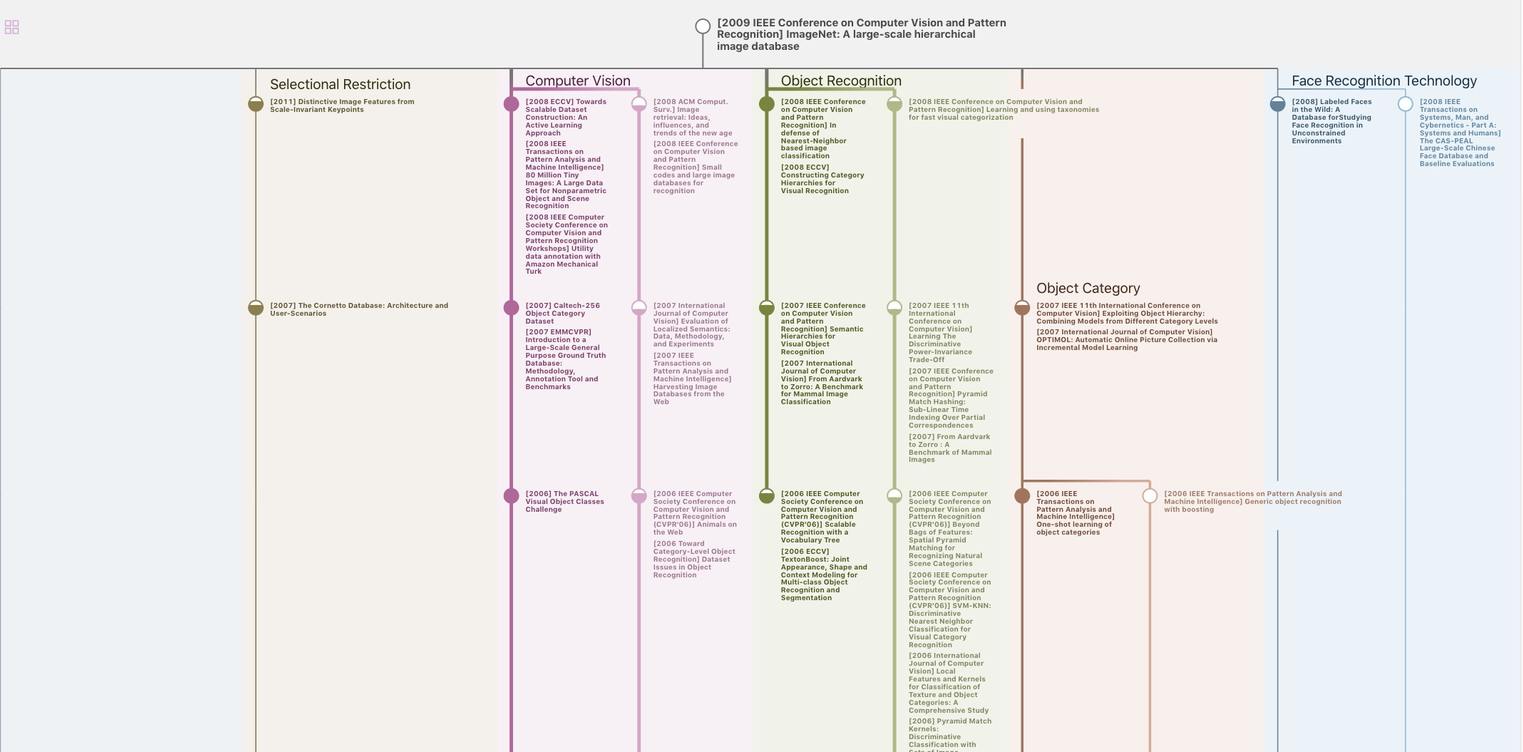
生成溯源树,研究论文发展脉络
Chat Paper
正在生成论文摘要