A Concentric Loop Convolutional Neural Network for Manual Delineation Level Building Boundary Segmentation from Remote Sensing Images
IEEE transactions on geoscience and remote sensing(2022)
摘要
To date, accurate building footprint delineation in the surveying, mapping, and geographic information system (GIS) communities has been dependent on human labor. In this article, to address this issue, we propose a concentric loop convolutional neural network (CLP-CNN) method for the automatic segmentation of building boundaries from remote-sensing images. The proposed method consists of three components: 1) a boundary detector to extract coarse polygonal boundaries of individual regions of interest; 2) a concentric loop-shaped convolutional network with bidirectional pairing loss to fine-tune the vertices of the polygons; and 3) a refinement block, which removes redundant vertices and regularizes the boundaries to polygons at the manual delineation level. We also demonstrate that the proposed CLP-CNN method is applicable to other generic objects in natural images. Experiments on two building datasets confirmed that more than 77%/67% of the building polygons predicted by the proposed method are on par with the manual delineation level, representing a significant saving in the labor cost of manual annotation. In generic object boundary delineation tests performed on the Semantic Boundaries Dataset (SBD), the proposed method outperformed the most recent state-of-the-art methods by at least 3.1% in average precision (AP). Furthermore, compared with other vertex matching methods, the learning process of the proposed method converges faster. The source code will be available at http://gpcv.whu.edu.cn/data .
更多查看译文
关键词
Building boundary extraction,convolutional neural network,instance segmentation,polygon regularization
AI 理解论文
溯源树
样例
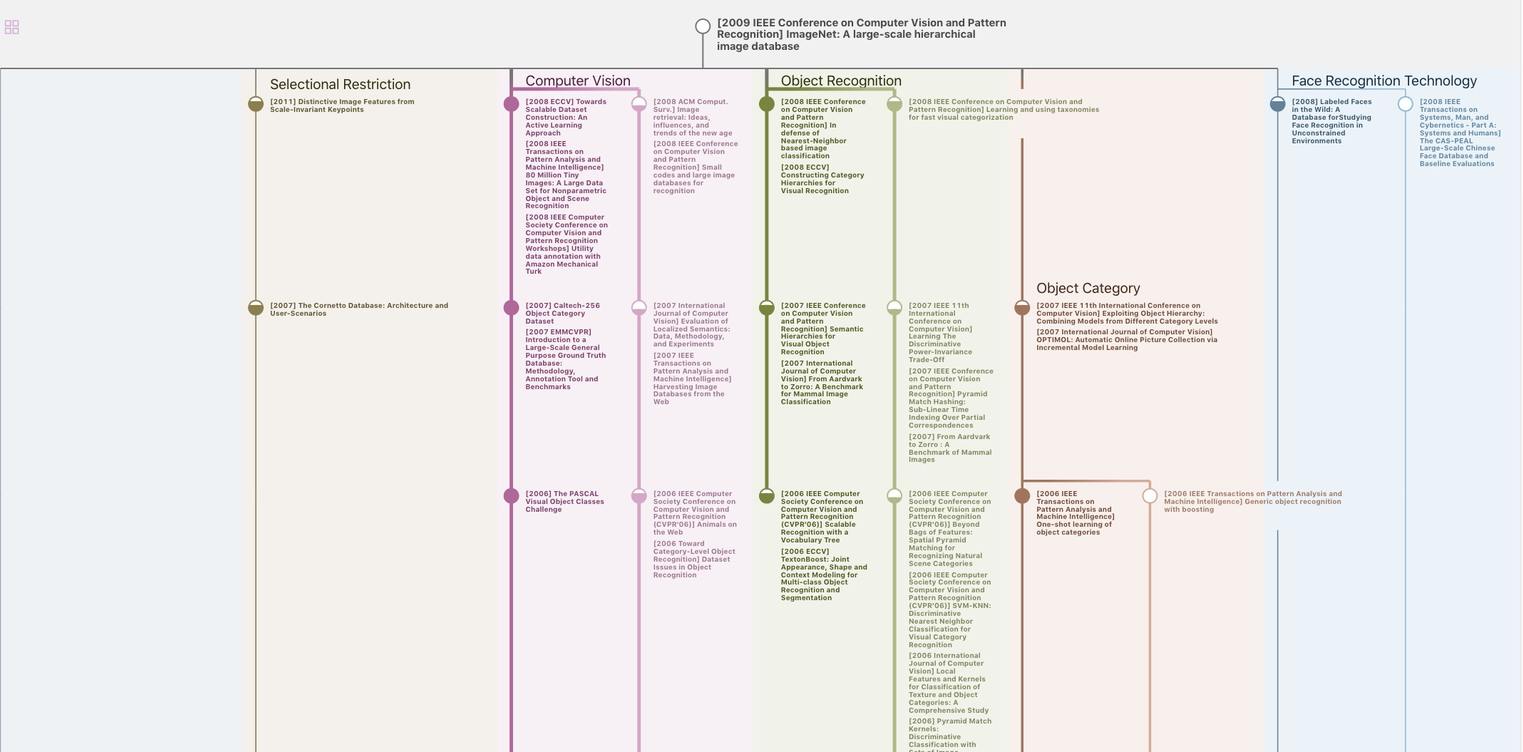
生成溯源树,研究论文发展脉络
Chat Paper
正在生成论文摘要