Deterministic Variational Inference for Neural SDEs
semanticscholar(2020)
摘要
Neural Stochastic Differential Equations (NSDEs) model the drift and diffusion functions of a stochastic process as neural networks. While NSDEs are known to predict time series accurately, their uncertainty quantification properties remain unexplored. Currently, there are no approximate inference methods, which allow flexible models and provide at the same time high quality uncertainty estimates at a reasonable computational cost. Existing SDE inference methods either make overly restrictive assumptions, e.g. linearity, or rely on Monte Carlo integration that requires many samples at prediction time for reliable uncertainty quantification. However, many real-world safety critical applications necessitate highly expressive models that can quantify prediction uncertainty at affordable computational cost. We introduce a variational inference scheme that approximates the posterior distribution of a NSDE governing a latent state space by a deterministic chain of operations. We approximate the intractable data fit term of the evidence lower bound by a novel bidimensional moment matching algorithm: vertical along the neural net layers and horizontal along the time direction. Our algorithm achieves uncertainty calibration scores that can be matched by its sampling-based counterparts only at significantly higher computation cost, while providing as accurate forecasts on system dynamics.
更多查看译文
关键词
inference
AI 理解论文
溯源树
样例
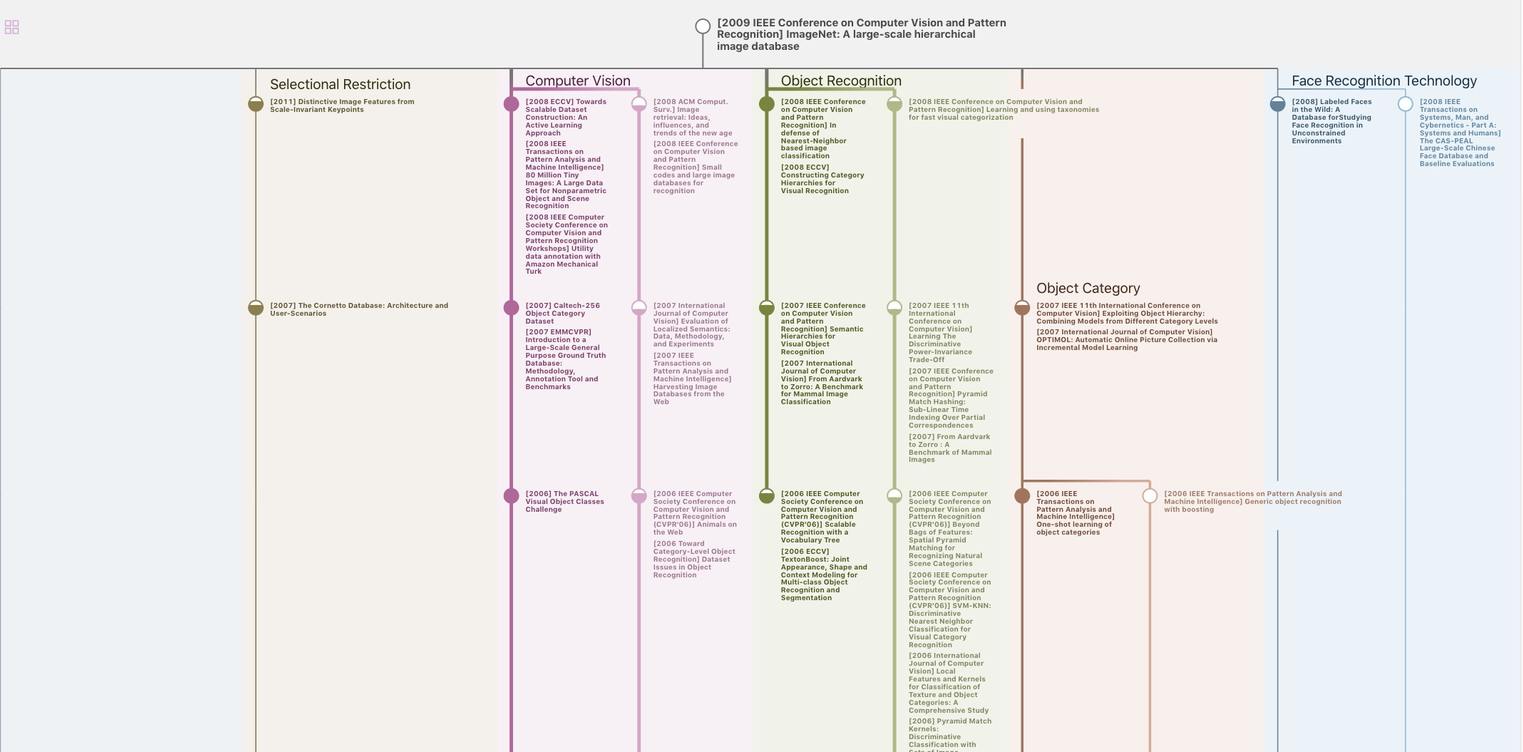
生成溯源树,研究论文发展脉络
Chat Paper
正在生成论文摘要