Learning Equilibrium Mean-Variance Strategy
Capital Markets: Asset Pricing & Valuation eJournal(2020)
摘要
We study a dynamic mean-variance portfolio optimization problem under the reinforcement learning framework, where an entropy regularizer is introduced to induce exploration. Due to the time-inconsistency involved in a mean-variance criterion, we aim to learn an equilibrium strategy. Under an incomplete market setting, we obtain a semi-analytical, exploratory, equilibrium mean-variance strategy that turns out to follow a Gaussian distribution. We then focus on a Gaussian mean return model and propose an algorithm to find the equilibrium strategy using the reinforcement learning technique. Thanks to a thoroughly designed policy iteration procedure in our algorithm, we can prove our algorithm's convergence under mild conditions, despite that dynamic programming principle and the usual policy improvement theorem fail to hold for an equilibrium solution. Numerical experiments are given to demonstrate our algorithm.
更多查看译文
关键词
equilibrium,strategy,learning
AI 理解论文
溯源树
样例
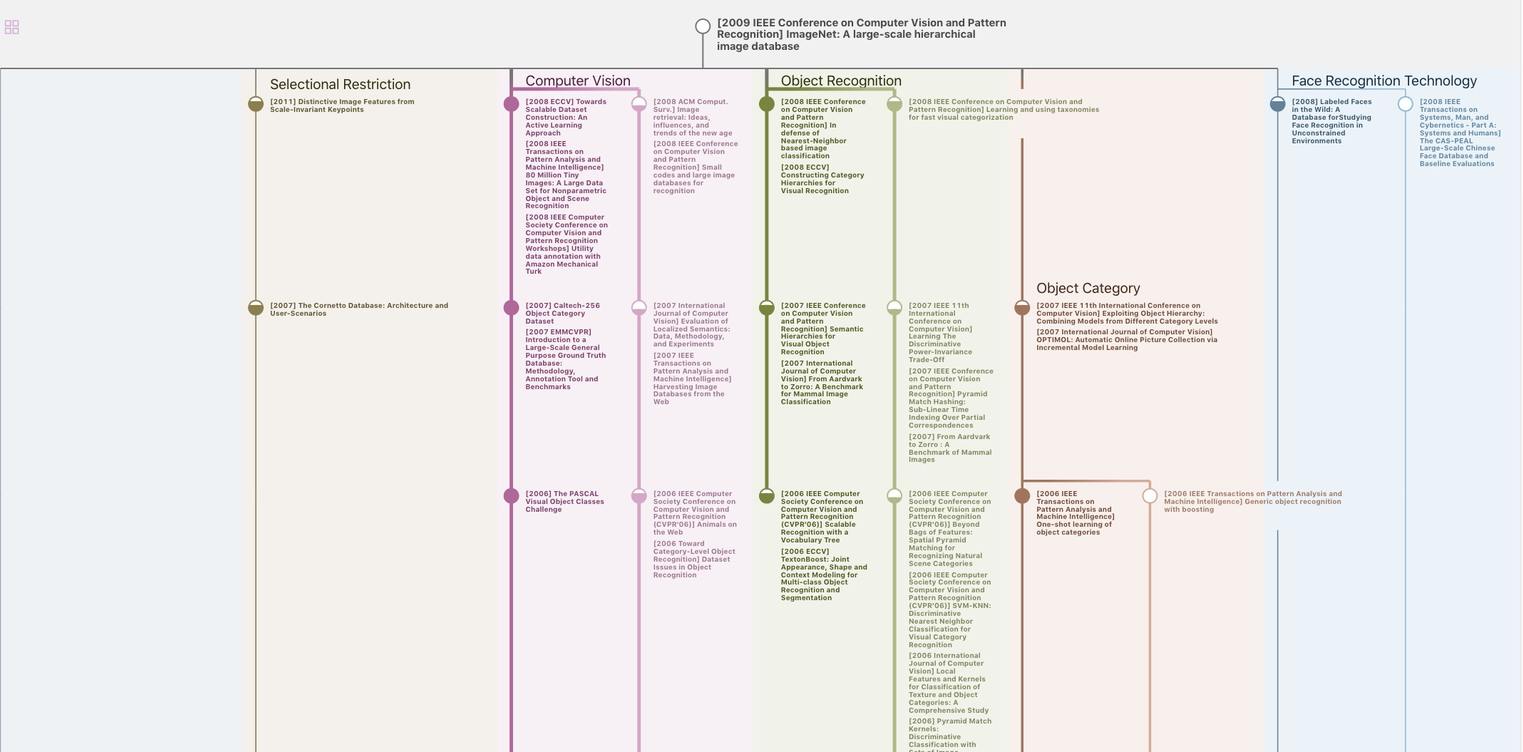
生成溯源树,研究论文发展脉络
Chat Paper
正在生成论文摘要