Improving Domain Generalization for Question Answering
semanticscholar(2021)
摘要
Domain generalization remains a major challenge for NLP systems. Our goal in this project is to build a question answering system that can adapt to new domains with very few training data from the target domain. We conduct experiments on three different techniques: 1) data augmentation, 2) task-adaptive pretraining (TAPT), and 3) multi-task finetuning. We find that while each individual approach improved out-of-domain question answering compared to the baseline DistiIBERT model, their combinations did not necessarily yield additional gains. Our best model implements data augmentation on both in-domain and out-of-domain train datasets with the DistiIBERT base model and achieved EM/F1 scores of 35.34/51.58 on the out-of-domain dev set and 42.32/60.17 on the held-out test set. We infer that we’ve comfortably met our goal of beating the baseline model’s performance as the baseline model achieved 32.98/48.14 on the out-of-domain dev set.
更多查看译文
AI 理解论文
溯源树
样例
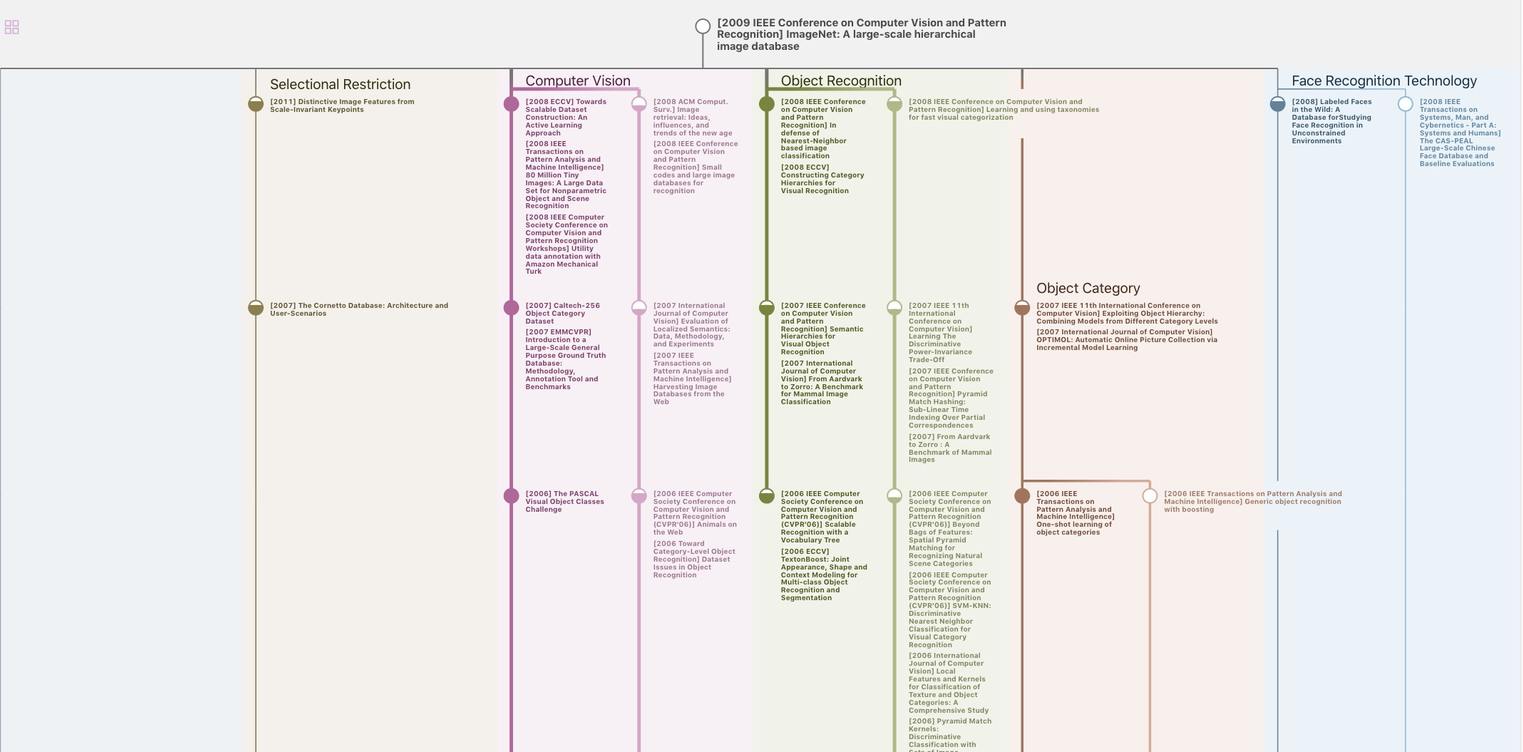
生成溯源树,研究论文发展脉络
Chat Paper
正在生成论文摘要