Can we use machine learning to discover risk factors? Testing the proof of principle using data on >11,000 predictors and mortality in the UK Biobank
medRxiv(2021)
摘要
Background: Machine learning (ML) can harness information from large databases with complex structures. We present a simple and fast hypothesis-free machine learning pipeline for risk factor discovery that accounts for non-linearity and interaction in large biomedical databases with minimal variable pre-processing. Methods: Mortality models were built using gradient boosting decision trees (GBDT) and important predictors were identified using SHAP values. Cox models controlled for false discovery rate were used for interpretability and further validation. The pipeline was tested using information from 502,506 UK Biobank participants, aged 37 to 73 years at recruitment and followed over seven years for mortality registrations. Results: From the 11,639 predictors included in GBDT, 193 potential risk factors had SHAP values 0.05 or greater and were selected for further modelling. Of the total variable importance summed up, 60% was directly health related, and baseline characteristics, sociodemographics, and lifestyle factors each contributed about 10%. Cox models adjusted for baseline characteristics, showed evidence for an association with mortality for 166 out of the 193 predictors. For 19 predictors we saw evidence for an association in the unadjusted but not adjusted analyses, suggesting confounding by basic characteristics. Identified "important" predictors included traditional risk factors such as age, sex, ethnicity, education, material deprivation, smoking, physical activity, self-rated health, BMI, hypertension, cardio-vascular diseases, cancer diagnoses and type 2 diabetes, as confirmed by previous studies. Conclusion: Our approach provides a fast and pragmatic solution for hypothesis free risk factor identification.
更多查看译文
AI 理解论文
溯源树
样例
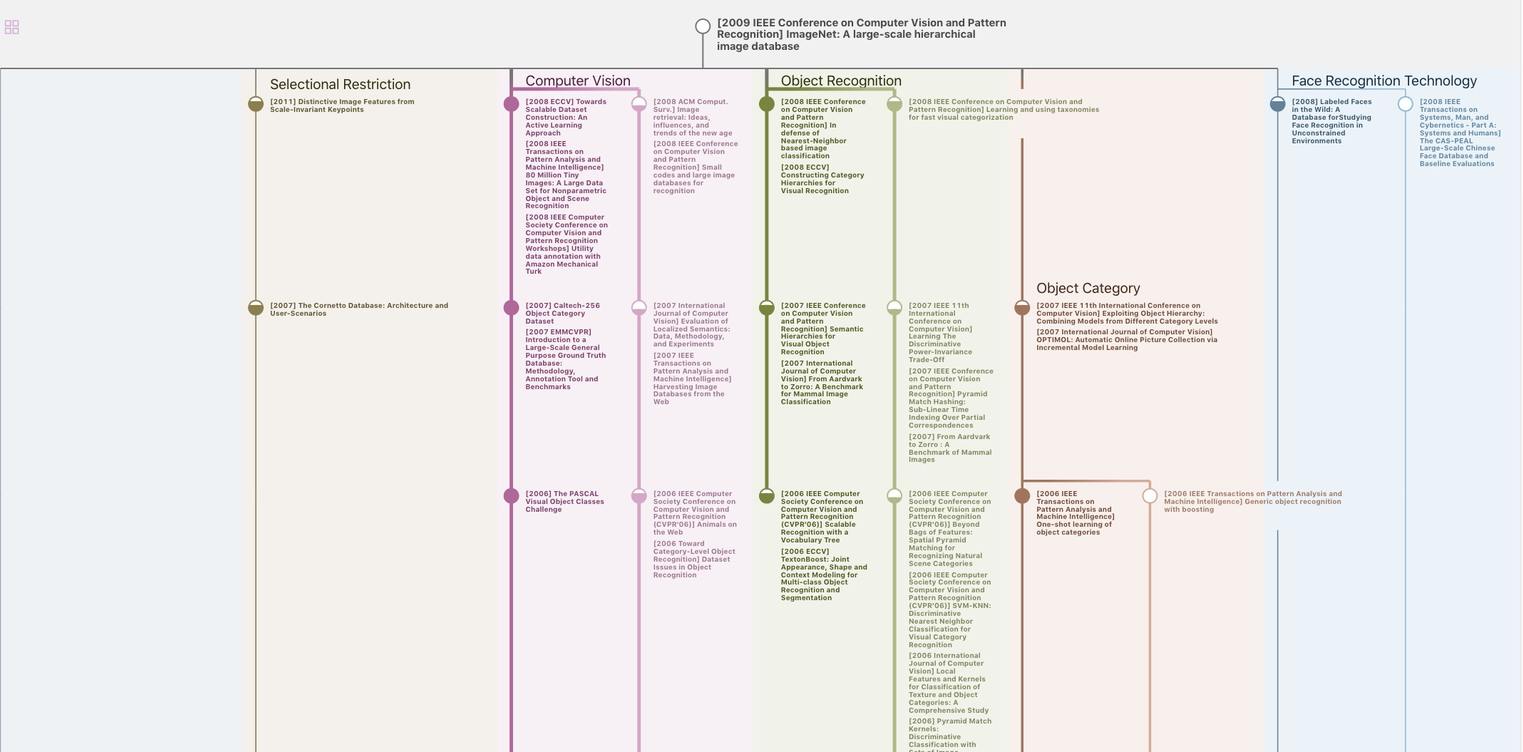
生成溯源树,研究论文发展脉络
Chat Paper
正在生成论文摘要