Model-Based Deep Learning: Key Approaches and Design Guidelines
2021 IEEE Data Science and Learning Workshop (DSLW)(2021)
摘要
Signal processing, communications, and control have traditionally relied on classical statistical modeling techniques. Such model-based methods tend to be sensitive to inaccuracies and may lead to poor performance when real systems display complex or dynamic behavior. On the other hand, purely data-driven approaches are becoming increasingly popular. Deep neural networks (DNNs) employ a highly flexible function class to learn mappings from data, and demonstrate excellent performance. However, DNNs typically require massive amounts of data and immense computational resources, limiting their applicability for some signal processing scenarios. We consider hybrid techniques that combine principled mathematical models with data-driven systems to benefit from the advantages of both approaches. Such model-based deep learning methods exploit both partial domain knowledge, via mathematical structures designed for specific problems, as well as learning from limited data. Here, we survey leading approaches for studying and designing model-based deep learning systems, along with concrete design guidelines and signal processing oriented examples.
更多查看译文
关键词
deep learning,design guidelines,key approaches,model-based
AI 理解论文
溯源树
样例
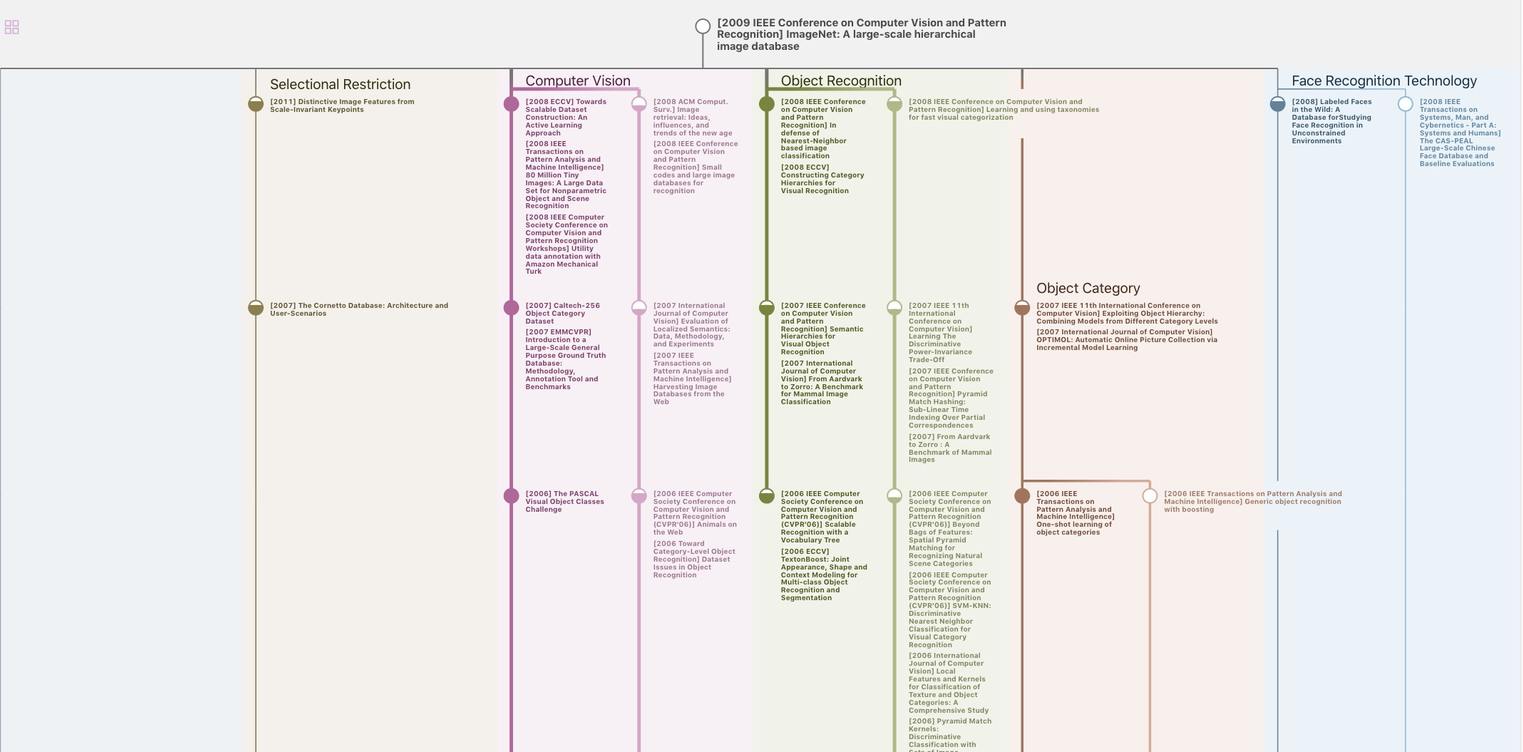
生成溯源树,研究论文发展脉络
Chat Paper
正在生成论文摘要