Prostate Cancer Detection Using Temporal Enhanced Ultrasound
semanticscholar(2020)
摘要
Introduction and overall goal: Prostate Cancer (PCa) detection under transrectal ultrasound (TRUS) guidance is blind to intraprostatic pathology, and can miss clinically significant disease. Multi-parametric MRI (mp-MRI) and its fusion with TRUS show promising results to target potential cancer lesions. However, mp-MRI has a high false positive rate, and can miss smaller foci of high-grade disease [1]. Ultrasoundbased tissue typing has been investigated extensively to enable more accurate targeted biopsy [2]. Rationale and background: Our group has been investigating a new ultrasound (US)-based paradigm for tissue characterization referred to as Temporal Enhanced Ultrasound (TeUS) [2]. TeUS is defined as the time-series of US frames captured by insonation of a fixed tissue location over time. We have previously demonstrated that spectral analysis of TeUS data can be deployed for detection of PCa in US images [2]. Specific aim: In this work, we aim to explicitly model the temporal relation of time series of US images for PCa detection instead of their spectral analysis [3]. Using a deep learning architecture, we demonstrate that this time-domain analysis leads to improved detection of PCa. Furthermore, the approach can be implemented in real time on commodity computer hardware. Methods and materials: Here, we use TeUS data obtained in a mp-MRI-US fusion biopsy clinical study with 200 biopsy cores from 157 patients. The temporal dependency of US time series images is modeled directly using Recurrent Neural Networks (RNNs). Specifically, we use Long Short-Term Memory (LSTM) networks, a special flavour of RNNs, to effectively learn long-term dependencies. We use the TensorFlow deep learning library (Google Corp., CA) to deploy these networks on computer graphics hardware in real time. Results: We achieved an area under the curve (AUC) of 0.80 and 0.93 for detection of PCa for all targets in mp-MRI and those identified as high risk, respectively. Discussion and conclusion: Our result suggests that TeUS can be potentially an effective approach for the detection of PCa in US images.
更多查看译文
AI 理解论文
溯源树
样例
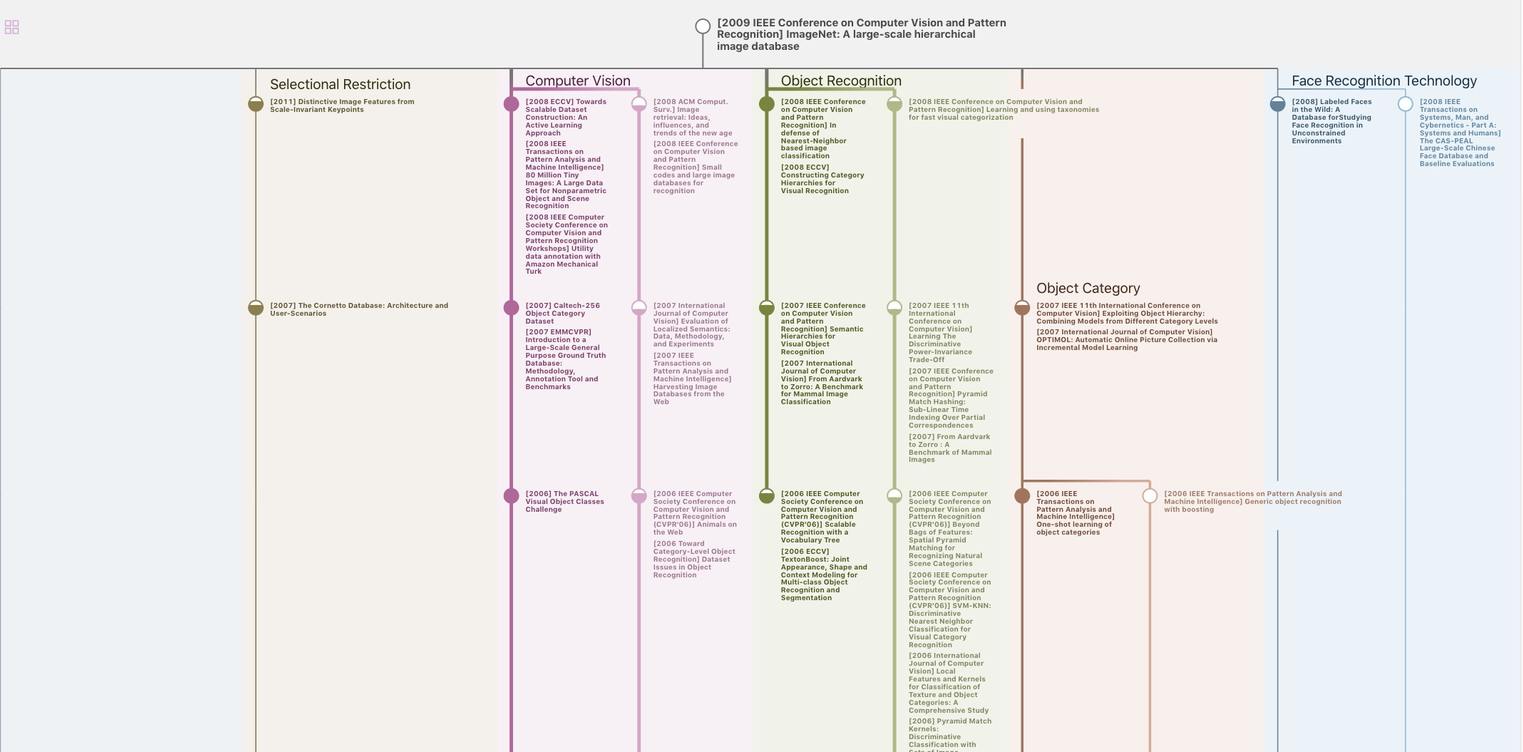
生成溯源树,研究论文发展脉络
Chat Paper
正在生成论文摘要