Model-Based Novelty Adaptation for Open-World AI
semanticscholar(2020)
摘要
One hallmark of human cognition is our ability to function in an open-world. People navigate to previously unseen places, perform new tasks, and integrate new technology into their lives. In games, human flexibility supports inventing new strategies along with adapting to changing rules (e.g., consider chess players who play bughouse1). While current AI systems perform superhuman in many game domains, each of these domains is a closed-world, and minor perturbations of the game can lead to significant drops in performance. Witty et al. demonstrated that even changes which made the game easier could cause catastrophic results for superhuman performing deep Qlearning agents (Witty et al., 2018). This mismatch between human cognitive abilities and machine capabilities indicates that adapting to novelty is a cognitive systems problem. In this paper, we introduce Hypothesis-Guided Model Revision over Multiple Aligned Representations (HYDRA), our approach to model-based novelty response. We take the cognitive systems view that learning is a goal-oriented activity undertaken when predictions from models differ from observations in the environment. We define the novelty problem in the Science Birds domain and outline our system design. Central to our design is the use of mixed continuous-discrete planning formalism, namely PDDL+ (Fox & Long, 2006), to model the Science Birds domain. We demonstrate how this enables HYDRA to play the game as well as adapt to many types of novelty by making localized modifications to the domain theory. Next, we present a case study demonstrating how HYDRA adapts its domain theory to changing dynamics in ballistic flight. We close with a discussion of different issues we expect to address in the course of this project.
更多查看译文
AI 理解论文
溯源树
样例
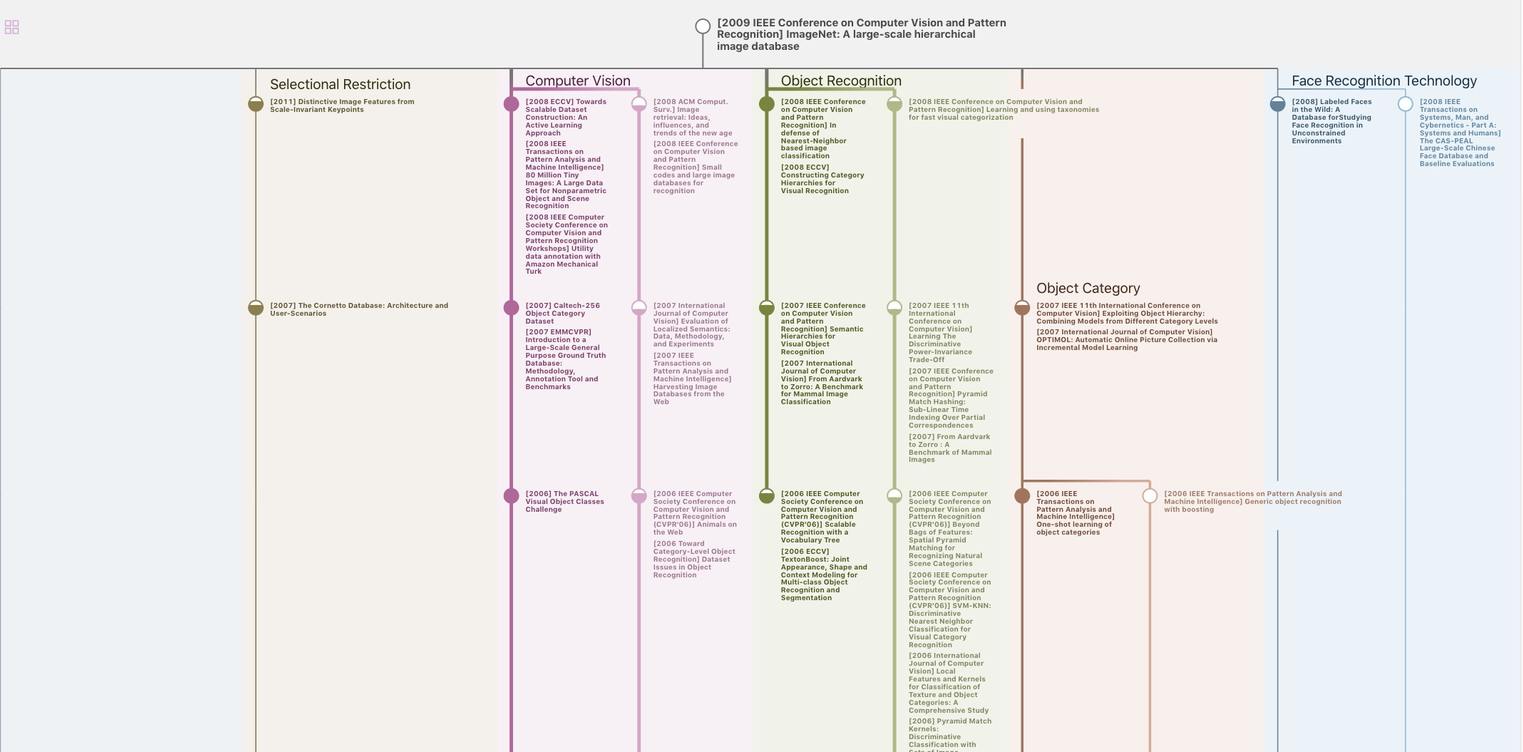
生成溯源树,研究论文发展脉络
Chat Paper
正在生成论文摘要