Feed Forward Back Propagation Neural Networks to Classify Freeway Traffic Flow State
mag(2013)
摘要
Analyses on traffic flow require accurate time-varying local traffic density information in order to effectively determine inflows to and outflows from freeway segments in several aspects of network traffic control. It is essential to specify the flow state, equivalently to derive density variable with the corresponding flow-rate measure, from primary traffic variables in order to provide accurate input to real-time traffic management strategies. In this paper, a study on the flow state specification process that employs feed forward back propagation neural network method to map sectional lane based density measure with raw traffic data collected from successive remote microwave sensor units mounted along a segment existing on the freeway network of Istanbul, is summarized. Classification of traffic flow states and matching the corresponding real-time flow state is obtained dynamically inputting raw flow measures simultaneously to neural density mapping and traffic flow modeling processes. The approach is promising in capturing instantaneous changes on flow states and may be utilized within intelligent management strategies such as incident control.
更多查看译文
关键词
Traffic Flow, Fundamental Diagram, Occupancy Data, Frequency Modulate Continuous Wave, Cell Transmission Model
AI 理解论文
溯源树
样例
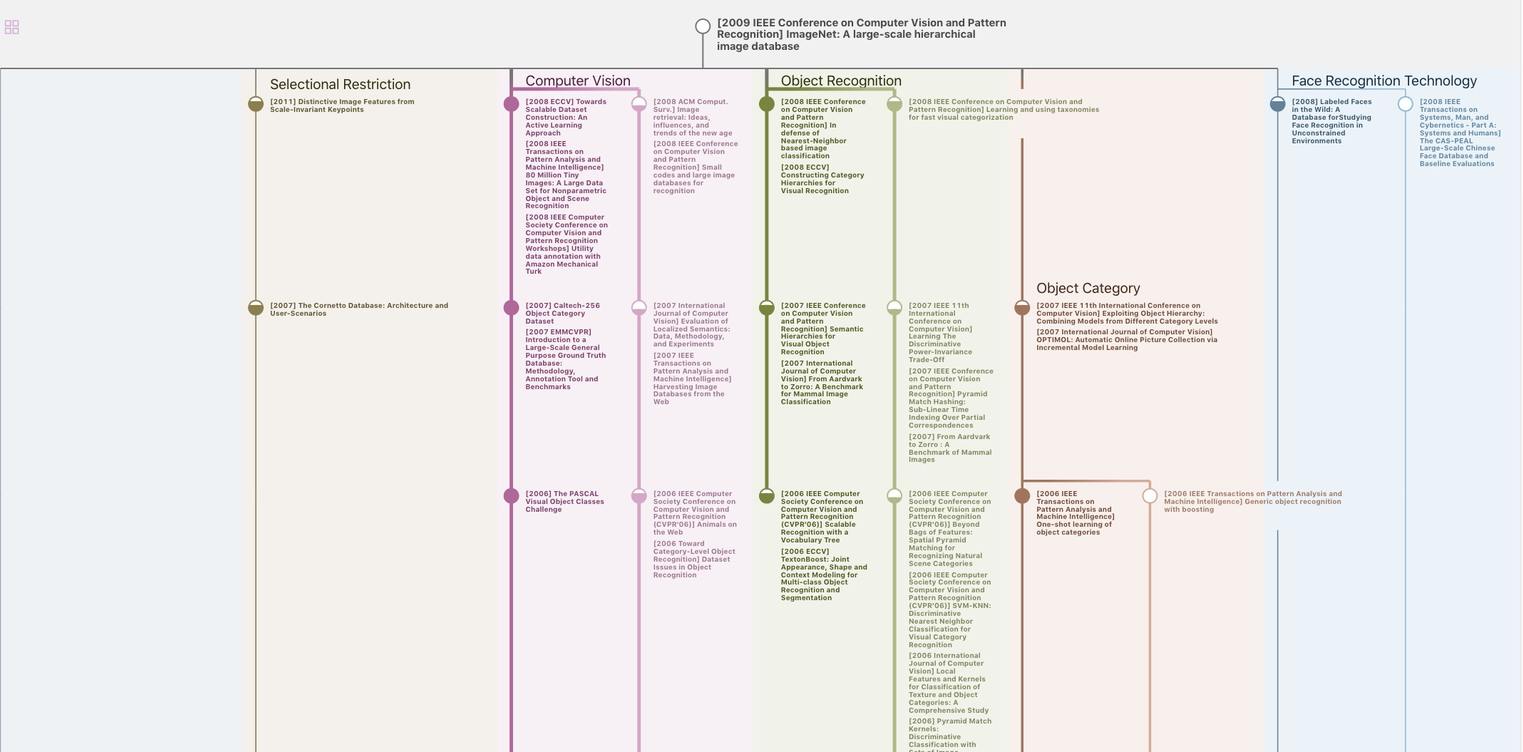
生成溯源树,研究论文发展脉络
Chat Paper
正在生成论文摘要