Deep Learning on medical images: ill-defined labels can be a good thing
semanticscholar(2021)
摘要
It is usual to come across public available datasets that often provide label distributions that do not match the real world distributions (RWD) where the data came from when working on deep learning (DL) algorithms for medical images. On top of that, it is possible to observe that data curation strategies developed for dataset creation could be partially responsible for the distancing of distributions present on said medical image datasets. By data curation we mean all processes of cleaning and transformation of the acquired data following certain semantic conditions and domain-specific knowledge. Although it is expected that the curation stage modify the distribution of the data, we signal that one must tap gently into the cleaning and transformation steps of data curation in order to avoid rendering a dataset worthless from the perspective of supervised learning DL algorithms. In this study, we will explore the experiences we had on the creation of two chest x-ray (CXR) datasets and one DL model for pulmonary tuberculosis (TB) classification. By promoting the discussion of data curation not only as process to filter noises and artifacts related to the data itself, but as a multidisciplinary approach needed to reduce unwanted bias in the process of dataset creation while demanding fidelity to RWD, we hope more attention will be bestowed upon the current limitations of DL models trained with medical image datasets that fall short of maintaining real world applicability.
更多查看译文
AI 理解论文
溯源树
样例
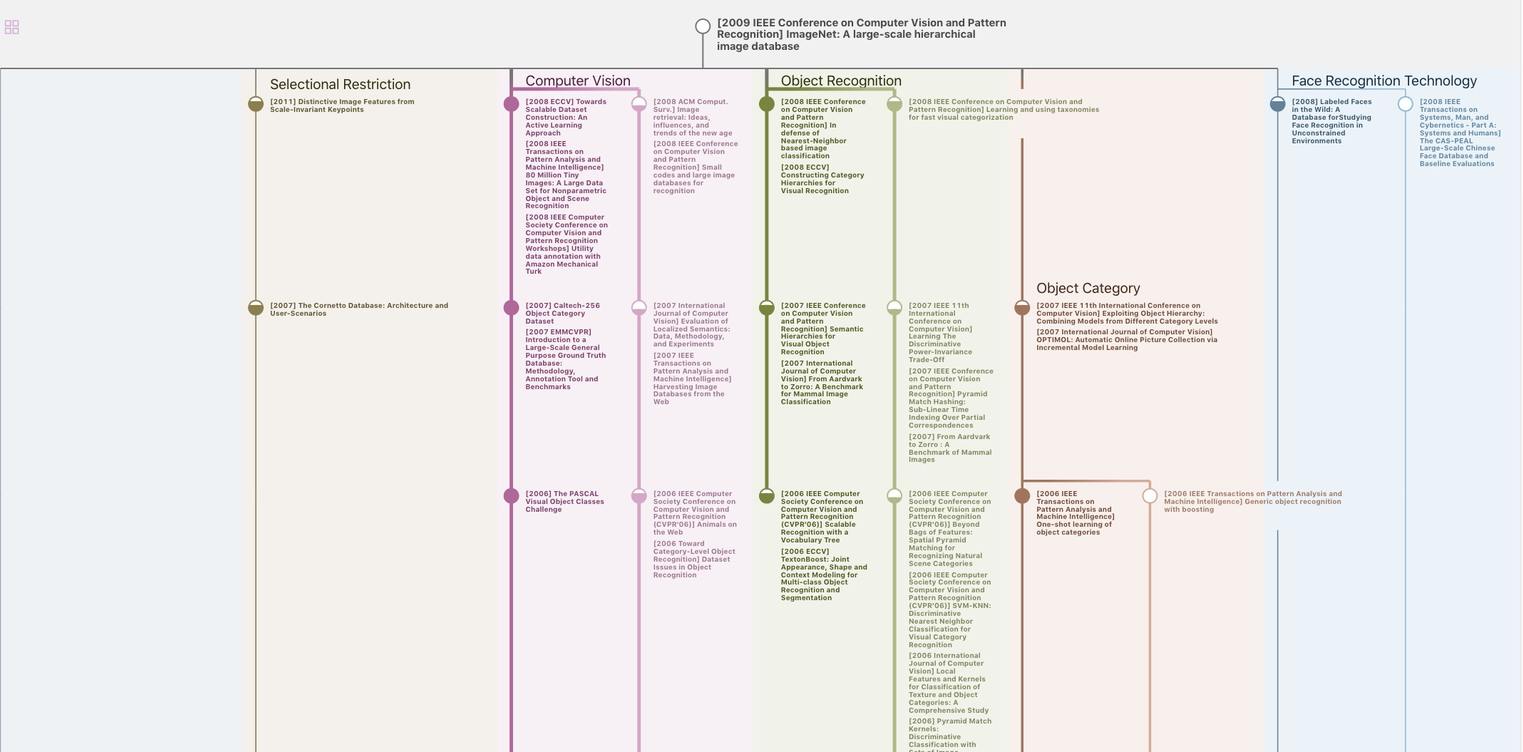
生成溯源树,研究论文发展脉络
Chat Paper
正在生成论文摘要