Using SARIMA–CNN–LSTM approach to forecast daily tourism demand
Journal of Hospitality and Tourism Management(2021)
摘要
Timely tourist demand forecasting is essential for the operation of the tourism industry; however, most studies focus on quarterly- or monthly-basis data, whose low-frequency nature makes it less informative than data at higher frequencies. In this article, we introduced a SARIMA–CNN–LSTM model to forecast tourist demand data at daily frequency, whose movement demonstrates the mixture of linear and nonlinear data features, difficult to model in the traditional framework. The SARIMA–CNN–LSTM model employs the SARIMA model and the deep neural network structure that combines the CNN and LSTM layers to capture linear and nonlinear data features. In the SARIMA–CNN–LSTM model structure, the SARIMA is used to capture the linear features. The Convolutional Neural Network (CNN) is used to capture the hierarchical data structure, while the Long Short Term Memory network (LSTM) is used to capture the long-term dependencies in the data. Our results confirmed that the SARIMA–CNN–LSTM model yields greater forecast accuracy than the individual models. The subtle nonlinear details in the residual are modeled better using the deep learning model. We found that the SARIMA–CNN–LSTM model can take advantage of the rich information in the high-frequency data better in the forecasting process.
更多查看译文
关键词
LSTM,Tourist arrivals,Deep neural network,Time-series model,CNN
AI 理解论文
溯源树
样例
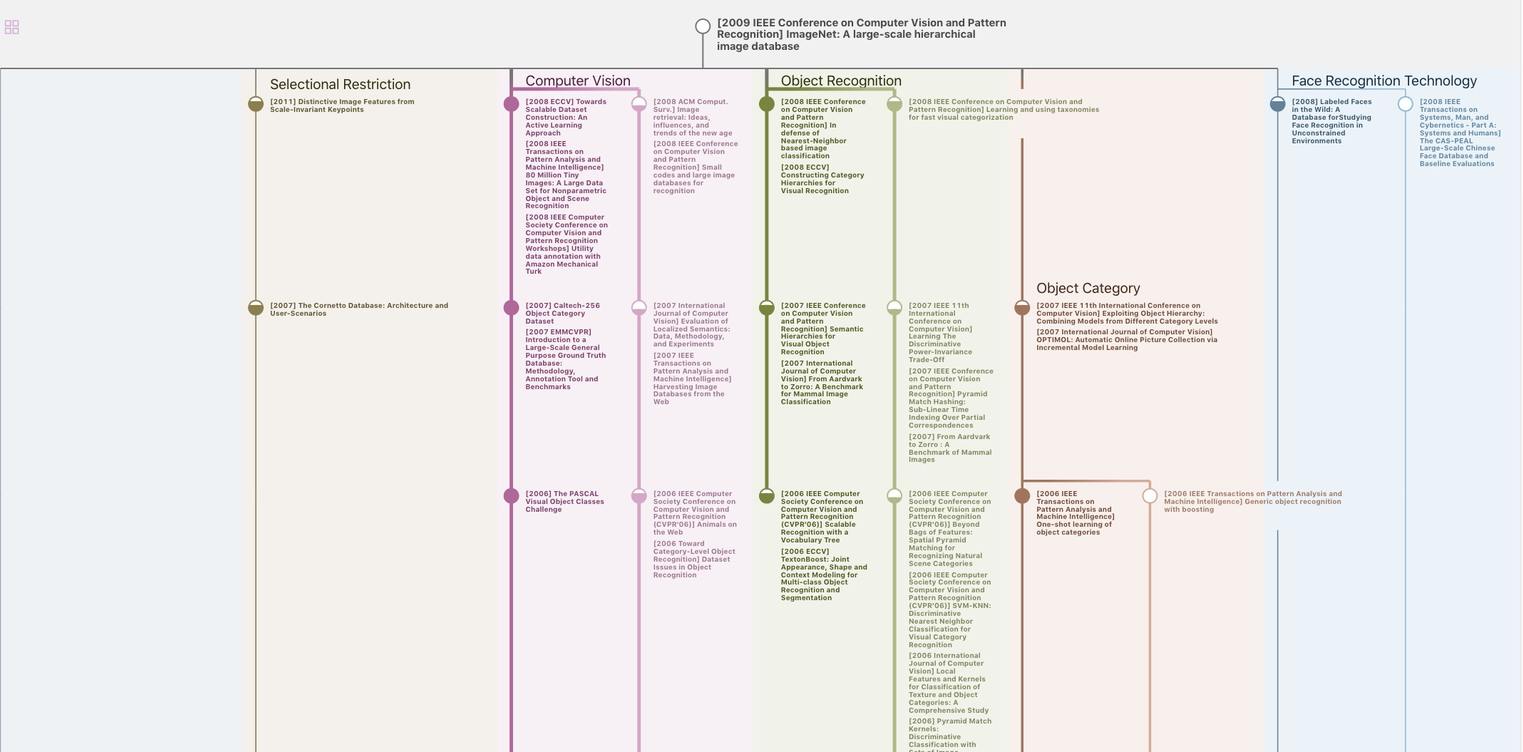
生成溯源树,研究论文发展脉络
Chat Paper
正在生成论文摘要