Advances in Computational Collective Intelligence: 12th International Conference, ICCCI 2020, Da Nang, Vietnam, November 30 – December 3, 2020, Proceedings
Advances in Computational Collective Intelligence(2020)
摘要
For industrial designers, how to classify vehicle styles was a challenge, and at a large depends on the designer’s internal knowledge. Although data mining and machine learning technologies had become mature and affordable today, the applications using machine learning in car styling were few. This investigation focuses on using the decision tree method to discuss the relationship between automotive styles and the design features of 35 cars produced by the automotive manufacturer, Dodge between 1942 and 2017. The study summarized 8 design features from previous literature: the length, fender design, number of headlamps, rear form, the position of the quarter glass, engine hood scoop, rocket tail, and side decoration designwhile the styles are chosenwere streamlined style, popular style, and modern style. The decision tree algorithm (C5.0) was employed to obtain the optimal rule of decision tree to compare the historic design styles from ten sets of decision tree rules. The result showed that there was a clear relationship between the key design features and the historic style of vehicles. The average accuracy of the ten sets of decision trees is 90.6%. The highest accuracy of the optimal model is 97%. However, the variation between the predicted accuracies of decision tree models calculated is high, ranging from 80% to 97%. Based on the decision tree and statistics method, the design features include the length, fender design, rocket tail design, rear form, and position of the quarter glass was more important than the others. This method had the potential to identify automotive historic styles based on key features.
更多查看译文
AI 理解论文
溯源树
样例
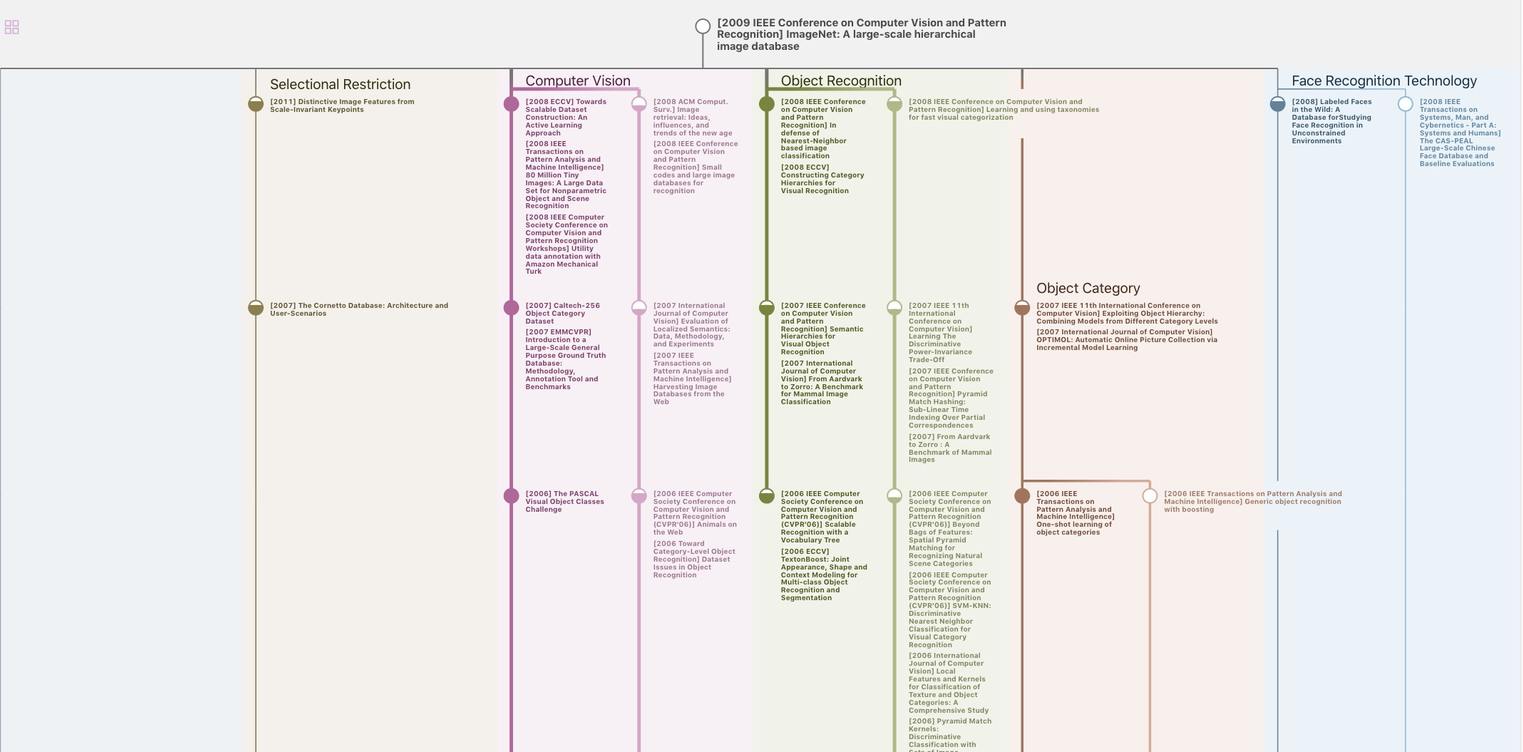
生成溯源树,研究论文发展脉络
Chat Paper
正在生成论文摘要