Machine learning prediction of the Madden-Julian oscillation
NPJ CLIMATE AND ATMOSPHERIC SCIENCE(2021)
摘要
The socioeconomic impact of weather extremes draws the attention of researchers to the development of novel methodologies to make more accurate weather predictions. The Madden–Julian oscillation (MJO) is the dominant mode of variability in the tropical atmosphere on sub-seasonal time scales, and can promote or enhance extreme events in both, the tropics and the extratropics. Forecasting extreme events on the sub-seasonal time scale (from 10 days to about 3 months) is very challenging due to a poor understanding of the phenomena that can increase predictability on this time scale. Here we show that two artificial neural networks (ANNs), a feed-forward neural network and a recurrent neural network, allow a very competitive MJO prediction. While our average prediction skill is about 26–27 days (which competes with that obtained with most computationally demanding state-of-the-art climate models), for some initial phases and seasons the ANNs have a prediction skill of 60 days or longer. Furthermore, we show that the ANNs have a good ability to predict the MJO phase, but the amplitude is underestimated.
更多查看译文
关键词
Atmospheric science,Mathematics and computing,Earth Sciences,general,Climate Change/Climate Change Impacts,Atmospheric Sciences,Climatology,Atmospheric Protection/Air Quality Control/Air Pollution
AI 理解论文
溯源树
样例
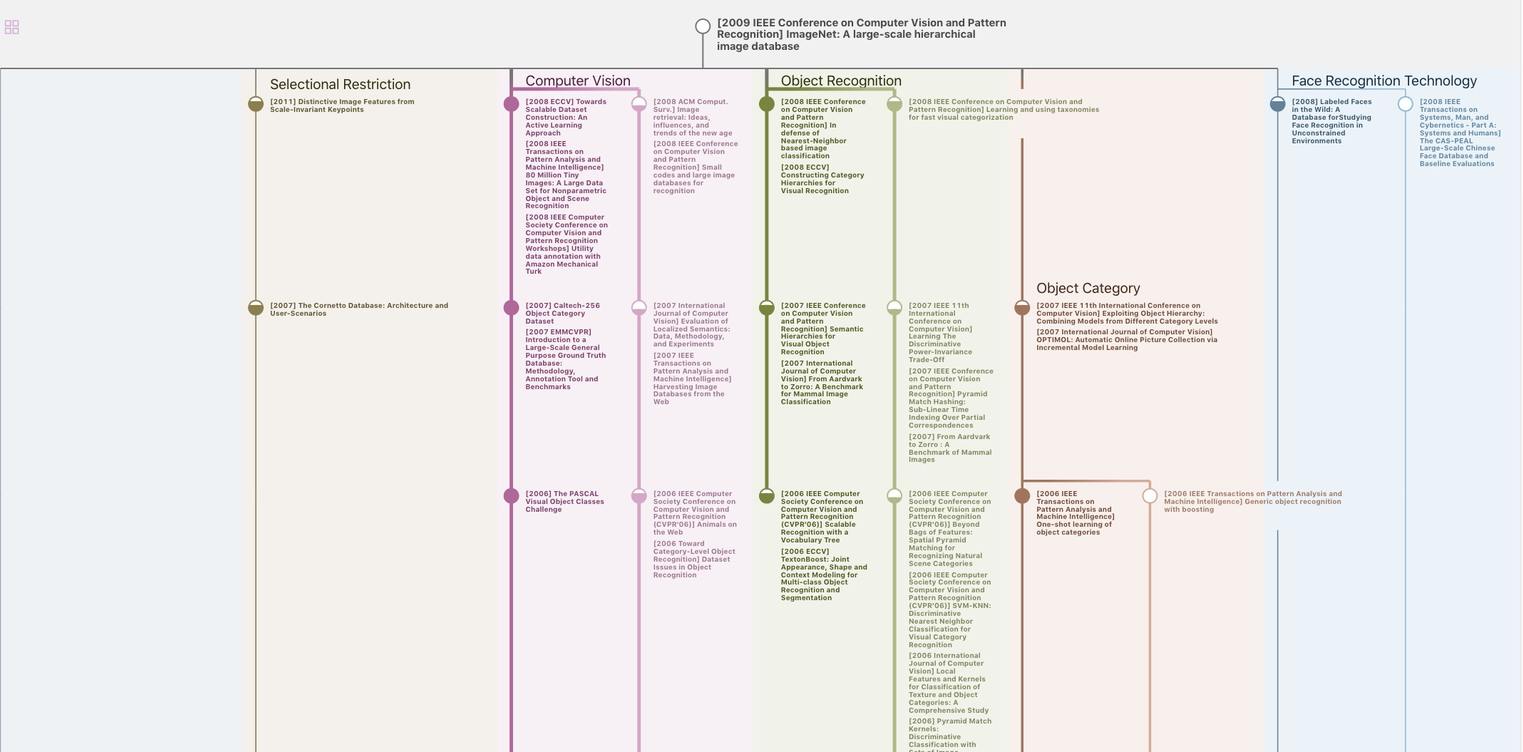
生成溯源树,研究论文发展脉络
Chat Paper
正在生成论文摘要