Feature Studies for PV Fault Classification Using Nonlinear Principal Component Analysis
semanticscholar(2021)
摘要
Solar fault classification neural networks have shown promise in recognizing fault conditions and improving power output of solar arrays in conjunction with Smart Monitoring Devices (SMD). These SMD’s contain sensors for recording 10 solar features on a solar panel and apply dynamic switching to maximize power output under a given fault. [1] The sensor data can be used to classify the common solar array faults soiling, arc faults, degraded modules, and shading. In this study solar feature data from is manipulated using kernel principal component analysis (KPCA) and autoencoder neural networks to reduce its dimension. The modified data is used to train fault classification neural networks with various dimensions of input features to compare classification accuracy. Through this process it was determined that the feature set had a significant amount of redundancy, but no nonlinear relationships that could be manipulated to improve fault classification accuracy.
更多查看译文
AI 理解论文
溯源树
样例
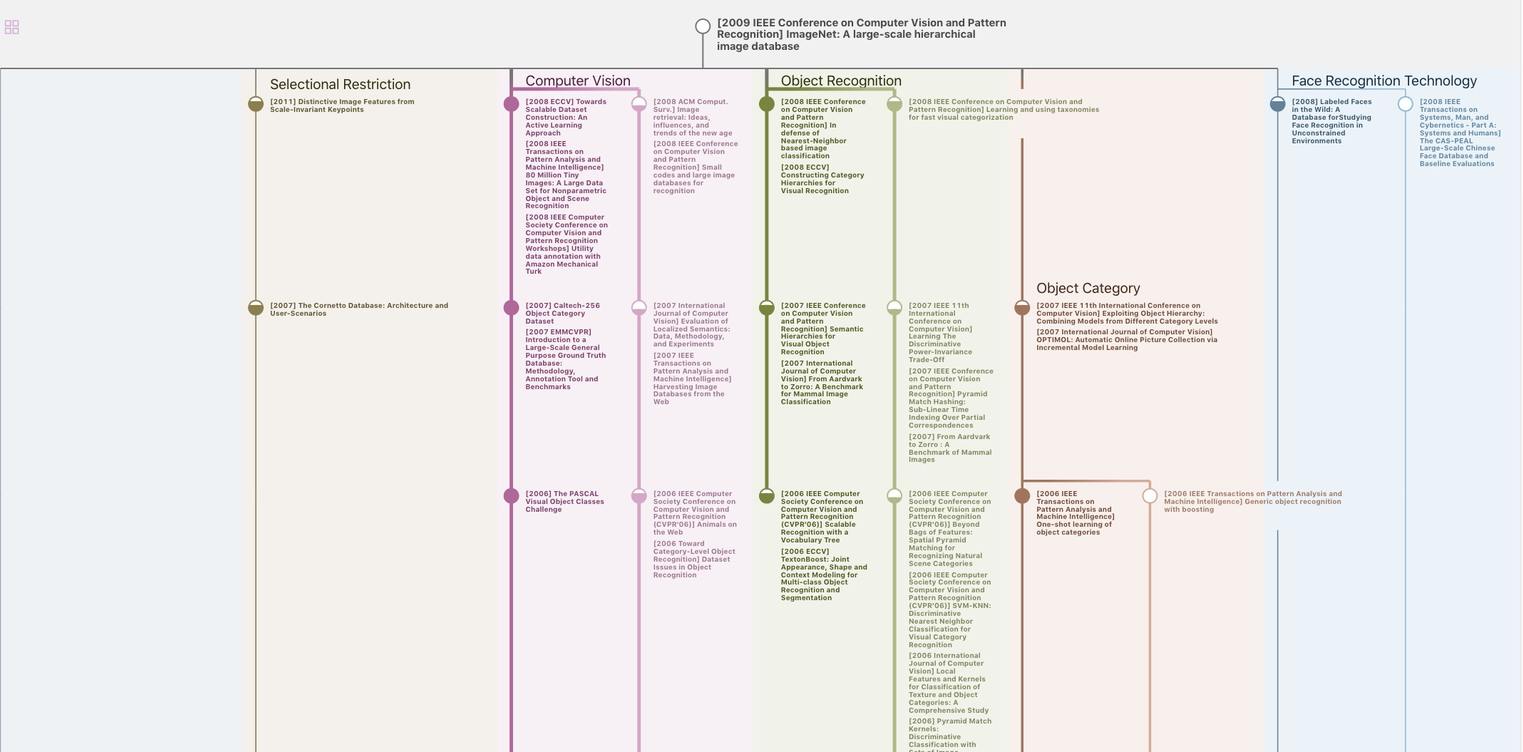
生成溯源树,研究论文发展脉络
Chat Paper
正在生成论文摘要