Cell type-agnostic representation of the human epigenome through a deep recurrent neural network
semanticscholar(2019)
Abstract
Sequencing-based assays such as ChIP-seq and ATAC-seq have recently been used to characterize the epigenome of hundreds of human cell types. These assays detailing a varied number of epigenomic functions like methylation status, local chromatin accessibility, histone modifications, factor binding and chromatin structure are hosted by consortia such as Roadmap Epigenomics [1] and ENCODE [2]. These data sets necessitate integrative methods that summarize them into a useful representation. A popular existing type of method is segmentation and genome annotation (SAGA) algorithms such as Segway [3] and ChromHMM [4], which produce an annotation of the epigenome of a given cell type. Existing SAGA annotations are cell type-specific; that is, they annotate activity in a given cell type. This corresponds poorly to most definitions of genomic elements, which are relative to the genome sequence itself. For example, annotations of protein coding genes are cell type-agnostic: they contain an archetypal set of gene locations where the locations are fixed and only the activity varies across cell types. Moreover, connecting a genetic locus to a phenotype or disease requires a cell type-agnostic understanding of its function. Existing SAGA algorithms cannot be adapted for this task because they use simple discrete or linear models that cannot capture the complexity of the epigenome across all cell types. We propose a method that produces a cell type-agnostic low-dimensional representation of the epigenome. This representation assigns a vector of features to each genomic position that represents that position’s activity across all tissues. We do this using a deep long short-term memory (LSTM) [6] recurrent neural network autoencoder to reduce all existing epigenome data into a single low-dimensional representation. This LSTM uses an autoencoder architecture in which aims to produce a representation that can be used reconstruct the original data as accurately as possible. One similar neural network representation learning method for epigenetics data exists. Like our method, Avocado produces a representation of the epigenome that assigns a low-dimensional vector to each genomic position. Avocado was initially developed for imputation. It uses distinct embeddings for each cell type, assay type and genomic position, and couples this with a feed-forward neural network that imputes unperformed assays. Our method has two advantages relative to Avocado. First, we use a sequential model that captures the spatial relationship of neighboring genomic positions. Second, our method can be applied genomic positions that were not used in training by inputting the relevant data into our encoder, while Avocado must use an expensive iterative optimization to do so. We demonstrate the utility of this representation through several analyses. First, we show that this representation simultaneously captures cell type-specific activity across many cell types, including gene expression, replication timing and chromatin contacts. We do this by demonstrating that all of the above phenomena are accurately predictable using just the latent representation. Second, we show that this latent representation distinguishes functional and non-functional regions by showing that the representation accurately identifies conserved regions. Third, we demonstrate
MoreTranslated text
AI Read Science
Must-Reading Tree
Example
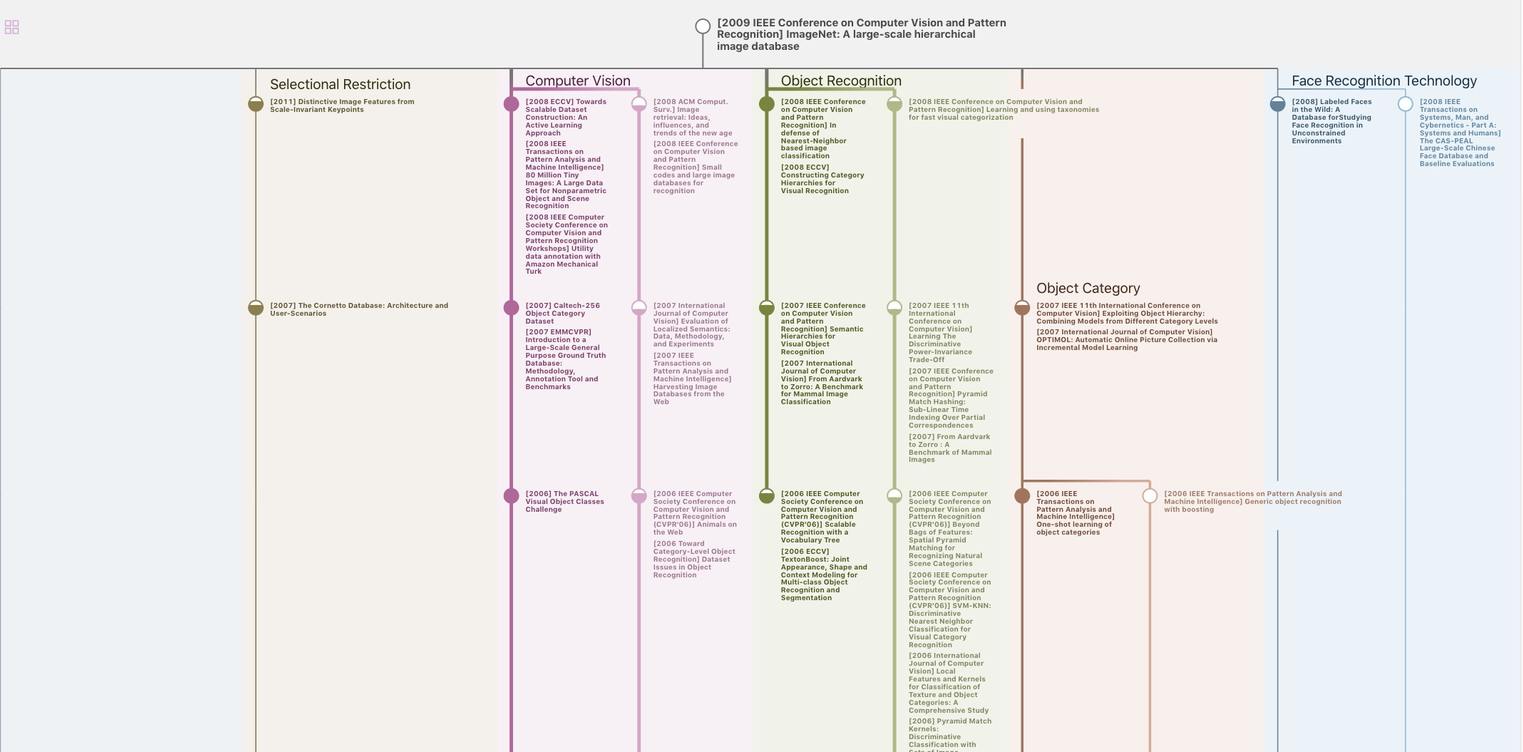
Generate MRT to find the research sequence of this paper
Chat Paper
Summary is being generated by the instructions you defined