Data Disclosure With Non-Zero Leakage and Non-Invertible Leakage Matrix
IEEE Transactions on Information Forensics and Security(2022)
摘要
We study a statistical signal processing privacy problem, where an agent observes useful data
$Y$
and wants to reveal the information to a user. Since the useful data is correlated with the private data
$X$
, the agent employs a privacy mechanism to generate data
$U$
that can be released. We study the privacy mechanism design that maximizes the revealed information about
$Y$
while satisfying a strong
$\ell _{1}$
-privacy criterion. When a sufficiently small leakage is allowed, we show that the optimizer distributions of the privacy mechanism design problem have a specific geometry, i.e., they are perturbations of fixed vector distributions. This geometrical structure allows us to use a local approximation of the conditional entropy. By using this approximation the original optimization problem can be reduced to a linear program so that an approximate solution for the optimal privacy mechanism can be easily obtained. The main contribution of this work is to consider a non-invertible leakage matrix with non-zero leakage. In our first example, inspired by a watermark application, we first demonstrate the accuracy of the approximation. Then, we employ different measures for utility and privacy leakage to compare the privacy-utility trade-off using our approach with other methods. In particular, we show that by allowing small leakage, significant utility can be achieved using our method compared to the case where no leakage is allowed. In the second and third examples which are based on the MNIST data set and medical applications, we illustrate the suggested design for disclosed data
$U$
. It has been shown that the letters of
$Y$
which are disclosing more information about
$X$
are combined (randomized) to produce a new letter of
$U$
.
更多查看译文
关键词
ℓ₁-privacy criterion,local approximation,privacy mechanism design,statistical signal processing
AI 理解论文
溯源树
样例
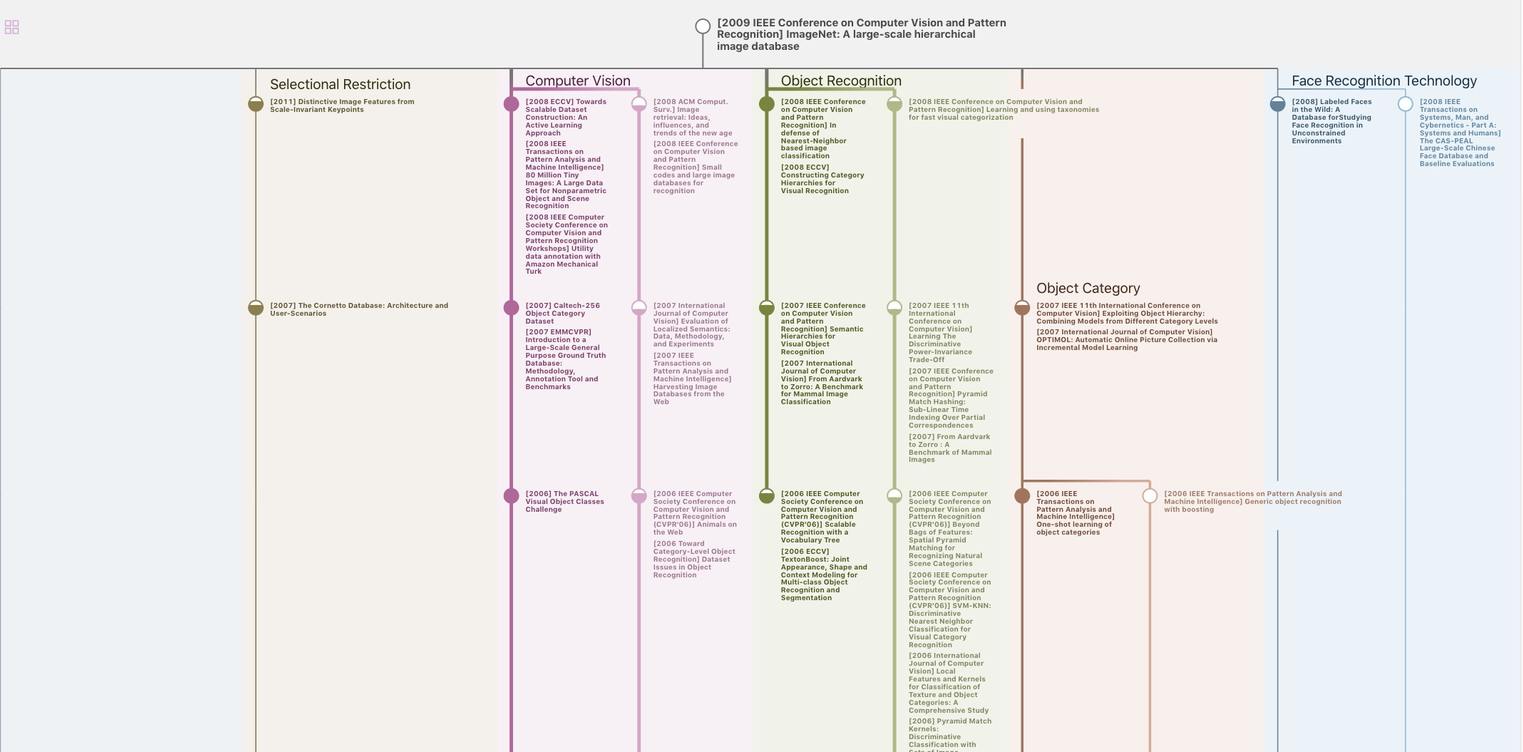
生成溯源树,研究论文发展脉络
Chat Paper
正在生成论文摘要