Overcoming Underrepresentation in Clinical Datasets for Accurate Subpopulation-specific Prognosis
medRxiv(2021)
摘要
Clinical datasets are intrinsically imbalanced, dominated by overwhelming majority groups. Off-the-shelf machine learning models optimize the prognosis of majority patient types (e.g., healthy class), causing substantial errors on the minority prediction class (e.g., disease class) and minority subpopulations (e.g., Black or young patients). For example, missed death prediction is 36.6 times higher than non-death cases in a mortality benchmark. Racial and age disparities also exist. Conventional metrics such as AUC-ROC do not reflect these deficiencies. We design a double prioritized (DP) sampling technique to improve the accuracy for underrepresented subpopulations. We report our findings on four prediction tasks over two clinical datasets, and comparisons with eight existing sampling solutions. With DP, the recall of minority classes shows 35.4-130.4% improvement. Compared to the state-of-the-arts, DP sampling gives 1.2-58.8 times more balanced recalls and precisions. Our method trains customized models for specific race or age groups, a departure from the one-model-fits-all-demographics paradigm. As underrepresented groups in clinical medicine are a daily occurrence, our contributions likely have broad implications.
更多查看译文
AI 理解论文
溯源树
样例
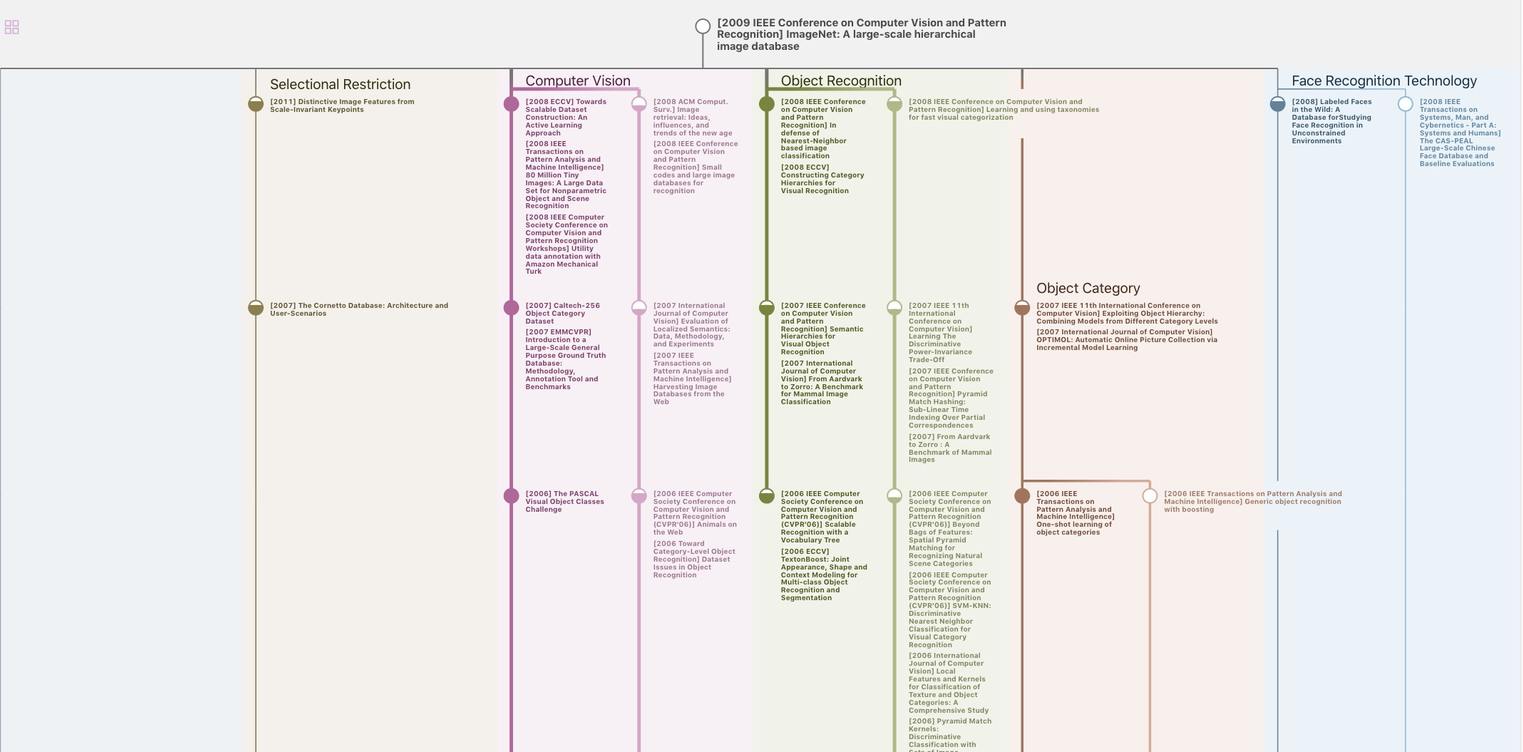
生成溯源树,研究论文发展脉络
Chat Paper
正在生成论文摘要