Unsupervised characterization of lessons according to temporal patterns of teacher talk via topic modeling
Neurocomputing(2022)
摘要
Classroom observation is an essential component to improve the quality of teaching and develop educational research. Nonetheless, traditional observation procedures involve previously trained observers, rendering them expensive and time-consuming. Thus, there is a need for a tool which enables to analyze multiple lessons in short time, represent those lessons consistently, and describe them according to the teaching strategies used over time to give teachers timely and continuous feedback. In this work, we propose two unsupervised approaches to represent the evolution of lessons based on topic modeling: textbook- and BERT-based. This way, we can improve the generalization capability of these tools and avoid the need of previously annotated data. Subsequently, we collapsed the derived topics into two super-topics to ease interpretability, and clustered the time series they define to characterize them according to the teaching strategies used. We applied our methodology to 195 science lessons revealing three patterns related to different strategies. Lastly, we compare the two approaches using different metrics for clustering assessment and discuss their benefits and detriments. We expect these models can become useful tools for teachers to obtain an automated and objective representation of their lessons, but also to other stakeholders who want to analyze teaching practices at a large scale.
更多查看译文
关键词
Time series clustering,Topic modeling,Latent Dirichlet allocation,BERT,Teacher’s talk automatic analysis
AI 理解论文
溯源树
样例
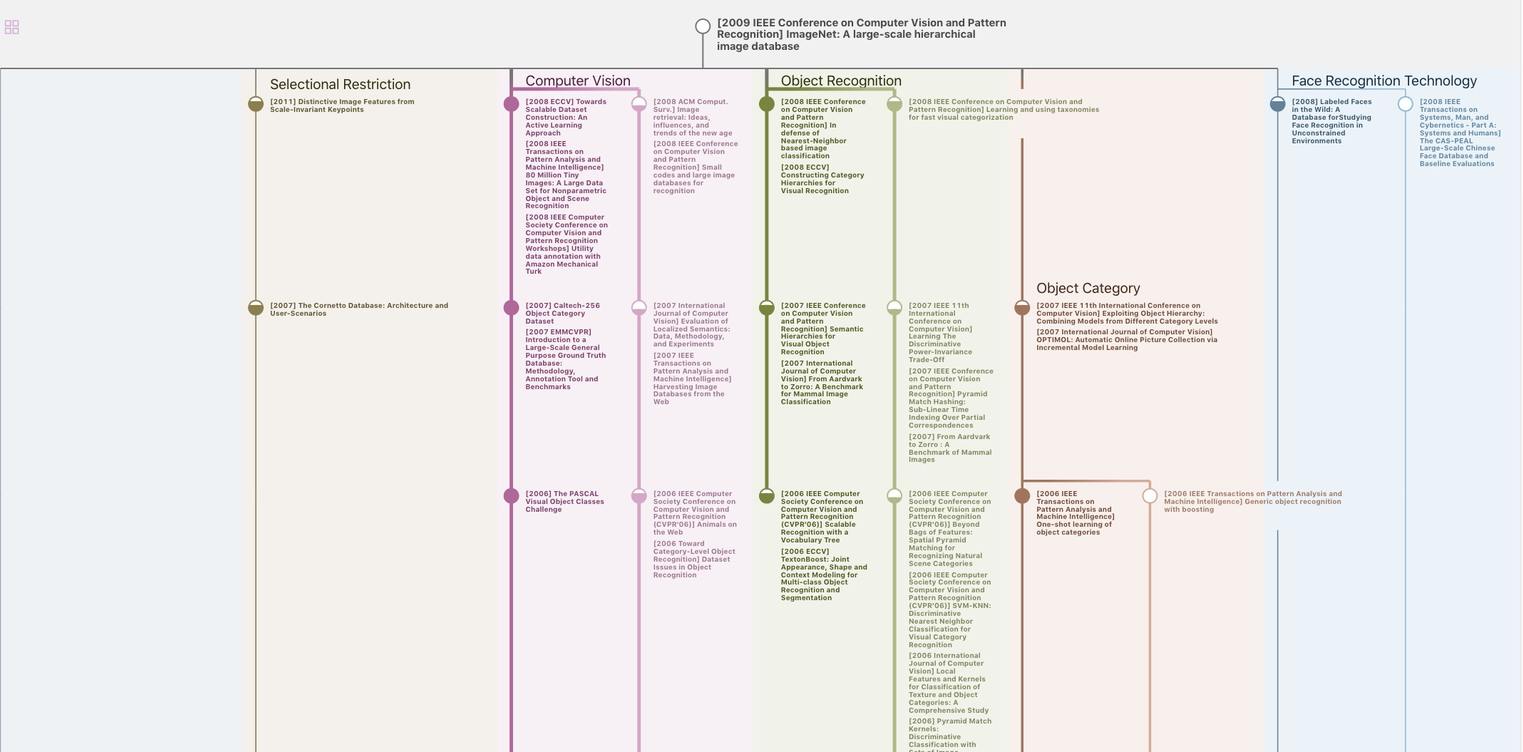
生成溯源树,研究论文发展脉络
Chat Paper
正在生成论文摘要