NPCFace: Negative-Positive Collaborative Training for Large-scale Face Recognition
semanticscholar(2020)
摘要
The training scheme of deep face recognition has greatly evolved in the past years, yet it encounters new challenges in the large-scale data situation where massive and diverse hard cases occur. Especially in the range of low false accept rate (FAR), there are various hard cases in both positives (intra-class) and negatives (inter-class). In this paper, we study how to make better use of these hard samples for improving the training. The literature approaches this by margin-based formulation in either positive logit or negative logits. However, the correlation between hard positive and hard negative is overlooked, and so is the relation between the margins in positive and negative logits. We find such correlation is significant, especially in the large-scale dataset, and one can take advantage from it to boost the training via relating the positive and negative margins for each training sample. To this end, we propose an explicit collaboration between positive and negative margins sample-wisely. Given a batch of hard samples, a novel Negative-Positive Collaboration loss, named NPCFace, is formulated, which emphasizes the training on both negative and positive hard cases via the collaborativemargin mechanism in the softmax logits, and also brings better interpretation of negative-positive hardness correlation. Besides, the emphasis is implemented with an improved formulation to achieve stable convergence and flexible parameter setting. We validate the effectiveness of our approach on various benchmarks of large-scale face recognition, and obtain advantageous results especially in the low FAR range.
更多查看译文
AI 理解论文
溯源树
样例
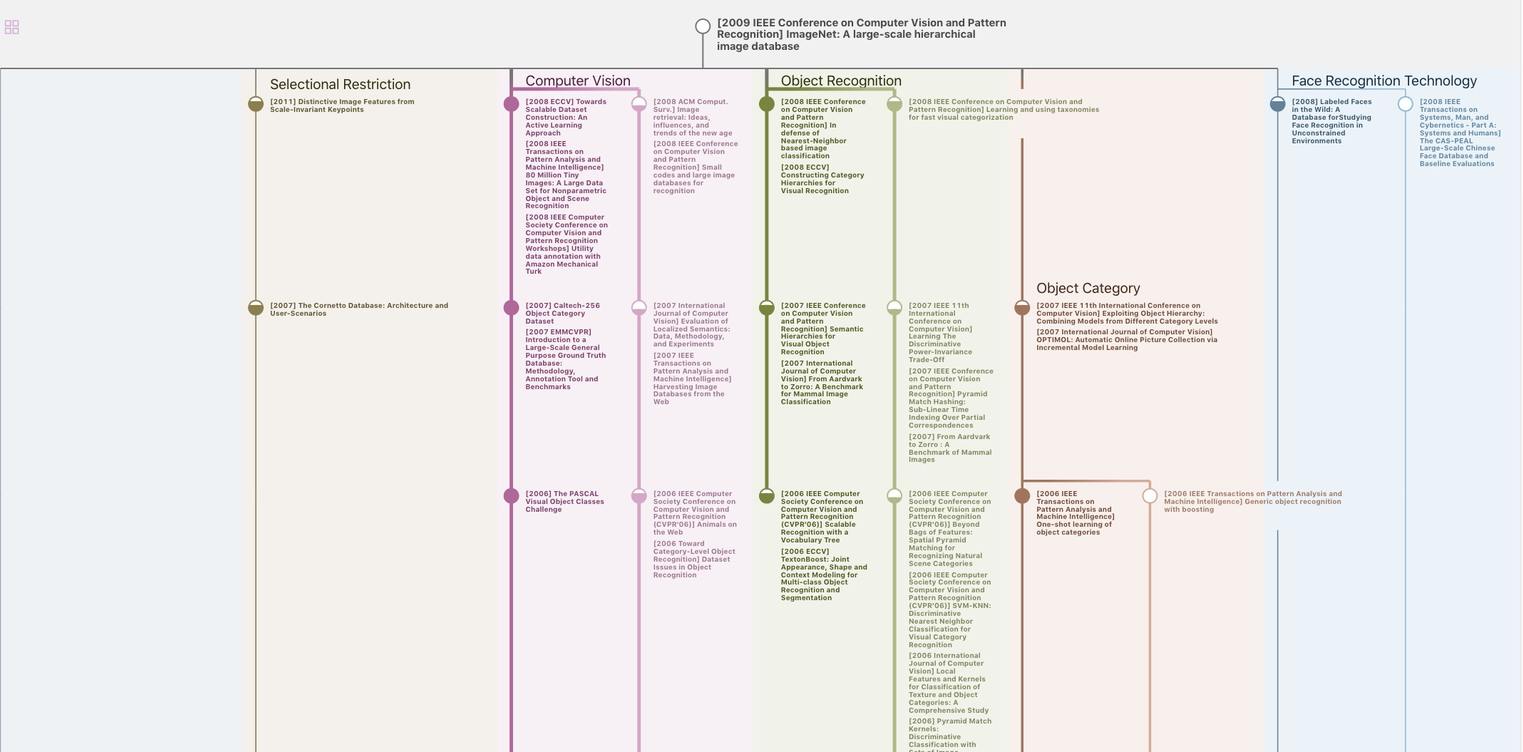
生成溯源树,研究论文发展脉络
Chat Paper
正在生成论文摘要