scSemiAE: a deep model with semi-supervised learning for single-cell transcriptomics
BMC Bioinformatics(2022)
摘要
Background With the development of modern sequencing technology, hundreds of thousands of single-cell RNA-sequencing (scRNA-seq) profiles allow to explore the heterogeneity in the cell level, but it faces the challenges of high dimensions and high sparsity. Dimensionality reduction is essential for downstream analysis, such as clustering to identify cell subpopulations. Usually, dimensionality reduction follows unsupervised approach. Results In this paper, we introduce a semi-supervised dimensionality reduction method named scSemiAE, which is based on an autoencoder model. It transfers the information contained in available datasets with cell subpopulation labels to guide the search of better low-dimensional representations, which can ease further analysis. Conclusions Experiments on five public datasets show that, scSemiAE outperforms both unsupervised and semi-supervised baselines whether the transferred information embodied in the number of labeled cells and labeled cell subpopulations is much or less.
更多查看译文
关键词
Semi-supervised, Dimensionality reduction, Autoencoder, Fine-tuning
AI 理解论文
溯源树
样例
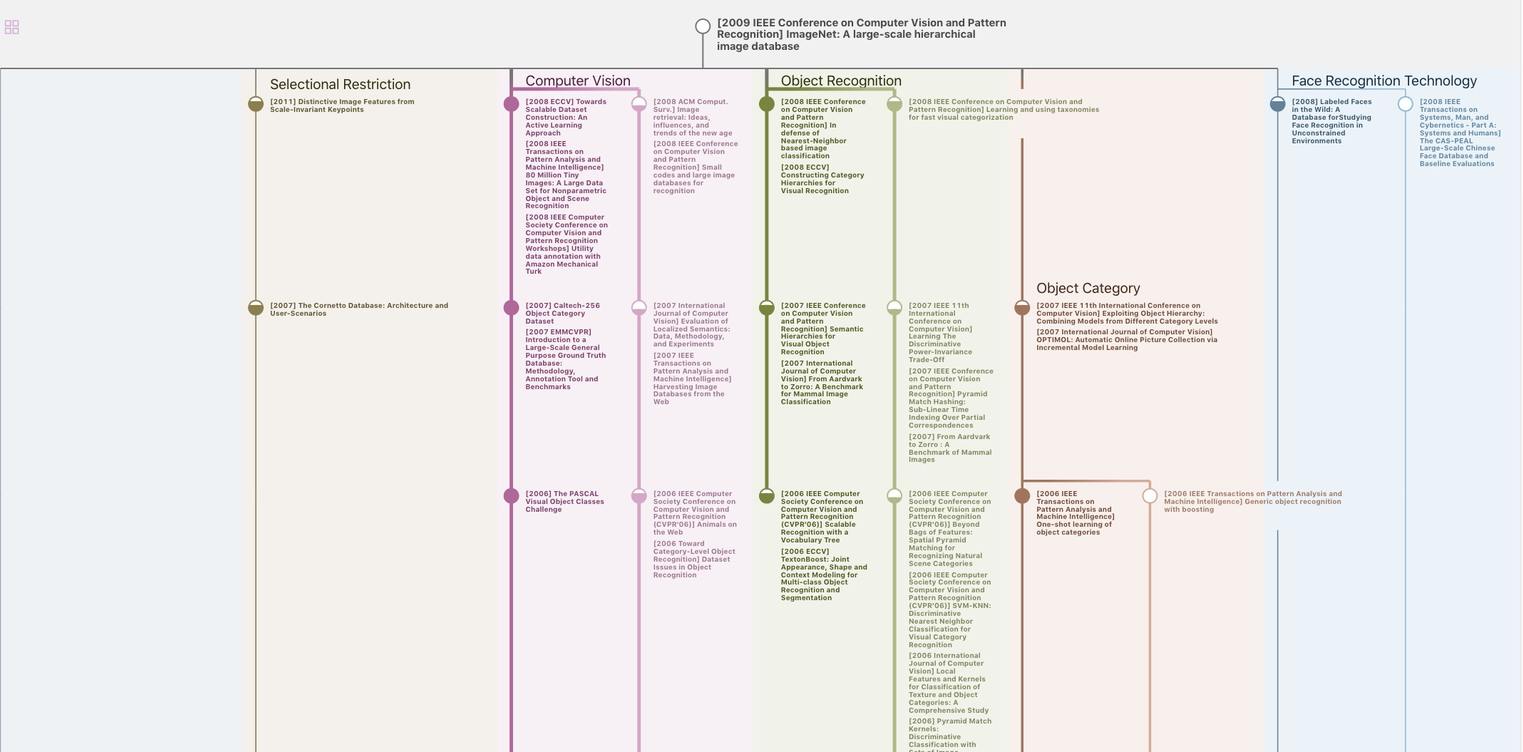
生成溯源树,研究论文发展脉络
Chat Paper
正在生成论文摘要