When FastText Pays Attention: Efficient Estimation of Word Representations using Constrained Positional Weighting.
CoRR(2021)
摘要
Since the seminal work of Mikolov et al. (2013a) and Bojanowski et al. (2017), word representations of shallow log-bilinear language models have found their way into many NLP applications. Mikolov et al. (2018) introduced a positional log-bilinear language model, which has characteristics of an attention-based language model and which has reached state-of-the-art performance on the intrinsic word analogy task. However, the positional model has never been evaluated on qualitative criteria or extrinsic tasks and its speed is impractical. We outline the similarities between the attention mechanism and the positional model, and we propose a constrained positional model, which adapts the sparse attention mechanism of Dai et al. (2019). We evaluate the positional and constrained positional models on three novel qualitative criteria and on the extrinsic language modeling task of Botha and Blunsom (2014). We show that the positional and constrained positional models contain interpretable information about word order and outperform the subword model of Bojanowski et al. (2017) on language modeling. We also show that the constrained positional model outperforms the positional model on language modeling and is twice as fast. “Words do not mean, people do.” (Wright, 2008)
更多查看译文
AI 理解论文
溯源树
样例
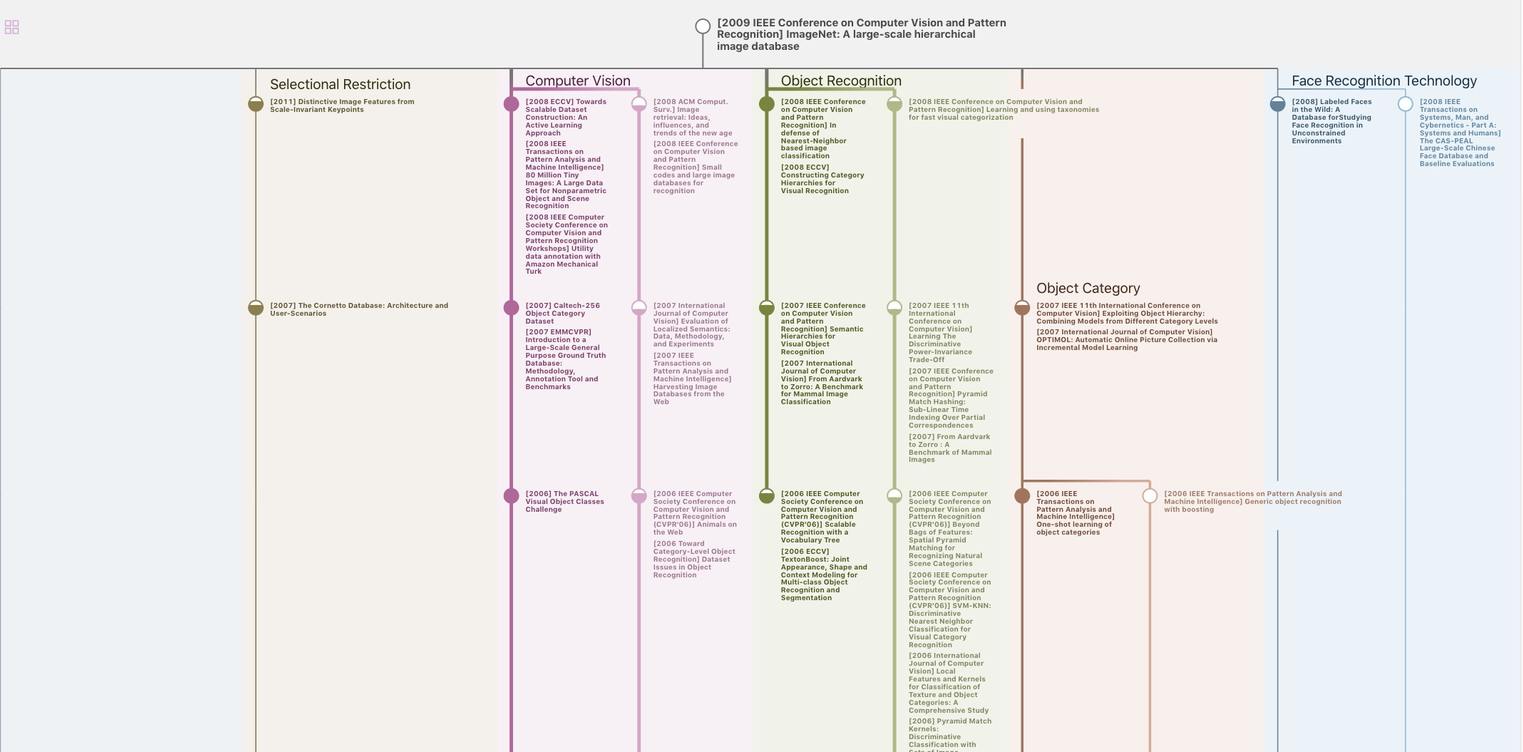
生成溯源树,研究论文发展脉络
Chat Paper
正在生成论文摘要