Safe and shorter path planning for autonomous mobile robots by multi-objective island-based parallel genetic algorithm with dominating pool
semanticscholar(2018)
摘要
This paper studies the application of evolutionary multi-objective optimization to path planning for mobile robots to move smoothly and safely along a shorter curvature-constrained path in completely known, planar static environments. The cost of travel is bi-objective: a new intrinsic cost of obstacle avoidance, which is designed as a weighted penetration depth to vertices of polygonal obstacles, and a length cost. The path is composed of a pre-specified number of control points, which are points of smooth turning, connected by three sub-paths composed of cubic spiral segments, where the intermediate configurations (locations and orientations) of these control points are design variables, subject to path smoothness constraint. We develop a Pareto-based evolutionary multi-objective optimization using island-based parallel genetic algorithm (IPGA) with nonsmoothness handling, aiming for searching smooth and shorter collision-free paths. To highlight the relative merit of IPGA in robustness to variations of environments, a comparative study on path planning performance based on simulations is conducted with two popular evolutionary multi-objective optimizers NSGA-II, SPEA-2 in terms of success rate in multiple runs and the shortest path length whenever a collision-free path can be successfully found. Results are presented for planar simulated rectangular environments composed of three distinct types of obstacles: polygons, walls as well as the combination of both. Our comparative study based on simulations shows that IPGA is more robust in all testing environments, while NSGA-II and SPEA-2 has a better distributed approximation to Pareto-front but sometimes the performance degrades greatly to be able to find a feasible path, especially in the environments containing wall-like obstacles.
更多查看译文
AI 理解论文
溯源树
样例
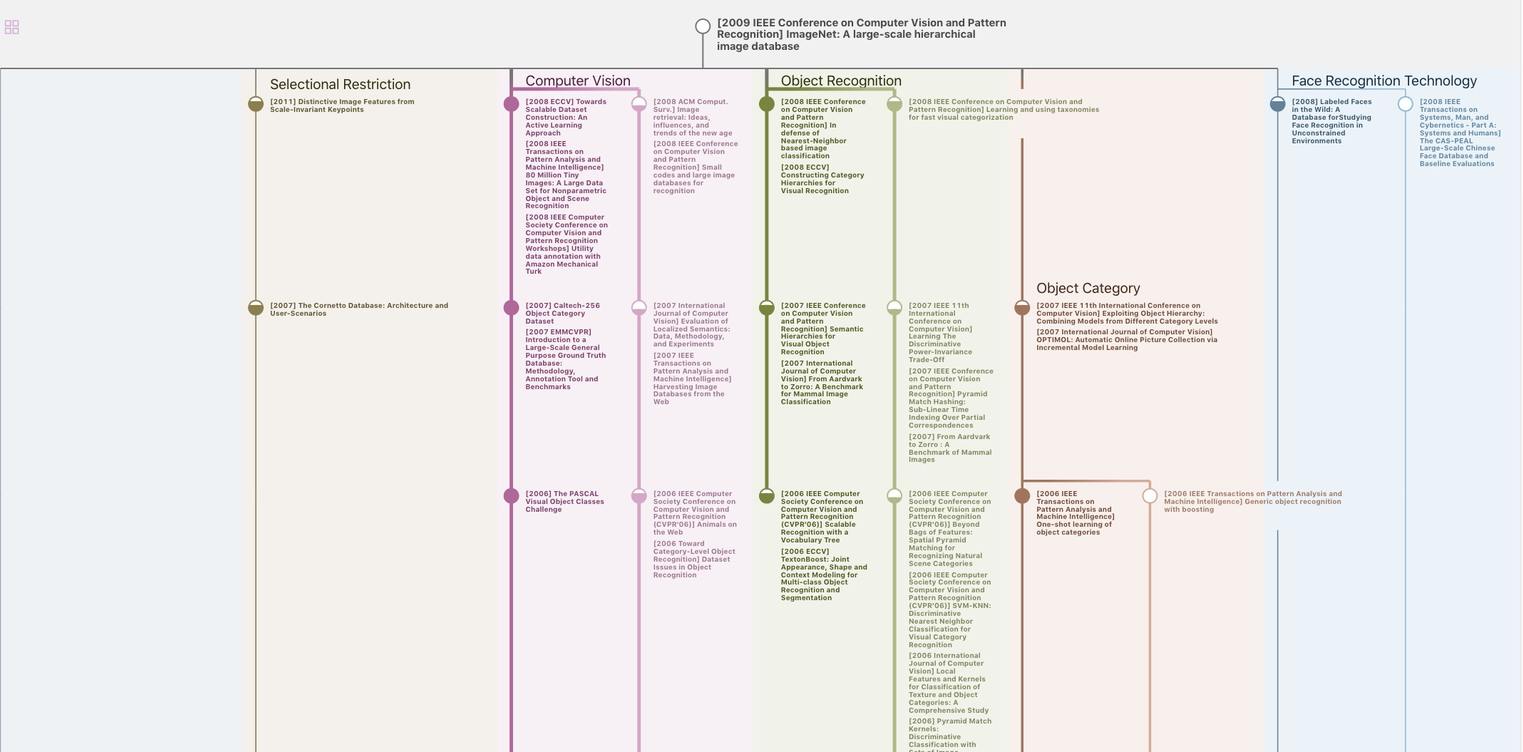
生成溯源树,研究论文发展脉络
Chat Paper
正在生成论文摘要