Machine learning-based direct solver for one-to-many problems of temporal shaping of electron bunches
semanticscholar(2021)
摘要
To control the temporal profile of a charged beam to meet requirements of various accelerator applications, a widely-used technique is bunch compression via 4-dipole chicanes that may sometimes have a one-to-many map. Current approaches based on stochastic optimization or supervised learning can be limited because of the one-to-many properties. Here we demonstrate how to construct a direct and real-time solver with the aid of a semi-supervised machine learning method, the conditional generative adversarial network (CGAN), to solve one-to-many problems of temporal shaping. Unlike supervised learning that can only learn one-to-one maps, the CGAN solver can learn the one-to-many dynamics and accurately predict required longitudinal dispersion terms for a chicane to realize desired custom temporal profiles without any priori knowledge. Besides, the CGAN solver can simultaneously give multiple different solutions for a one-to-many problem, which breaks the limitation of stochastic optimization methods of finding one solution instead of many.
更多查看译文
AI 理解论文
溯源树
样例
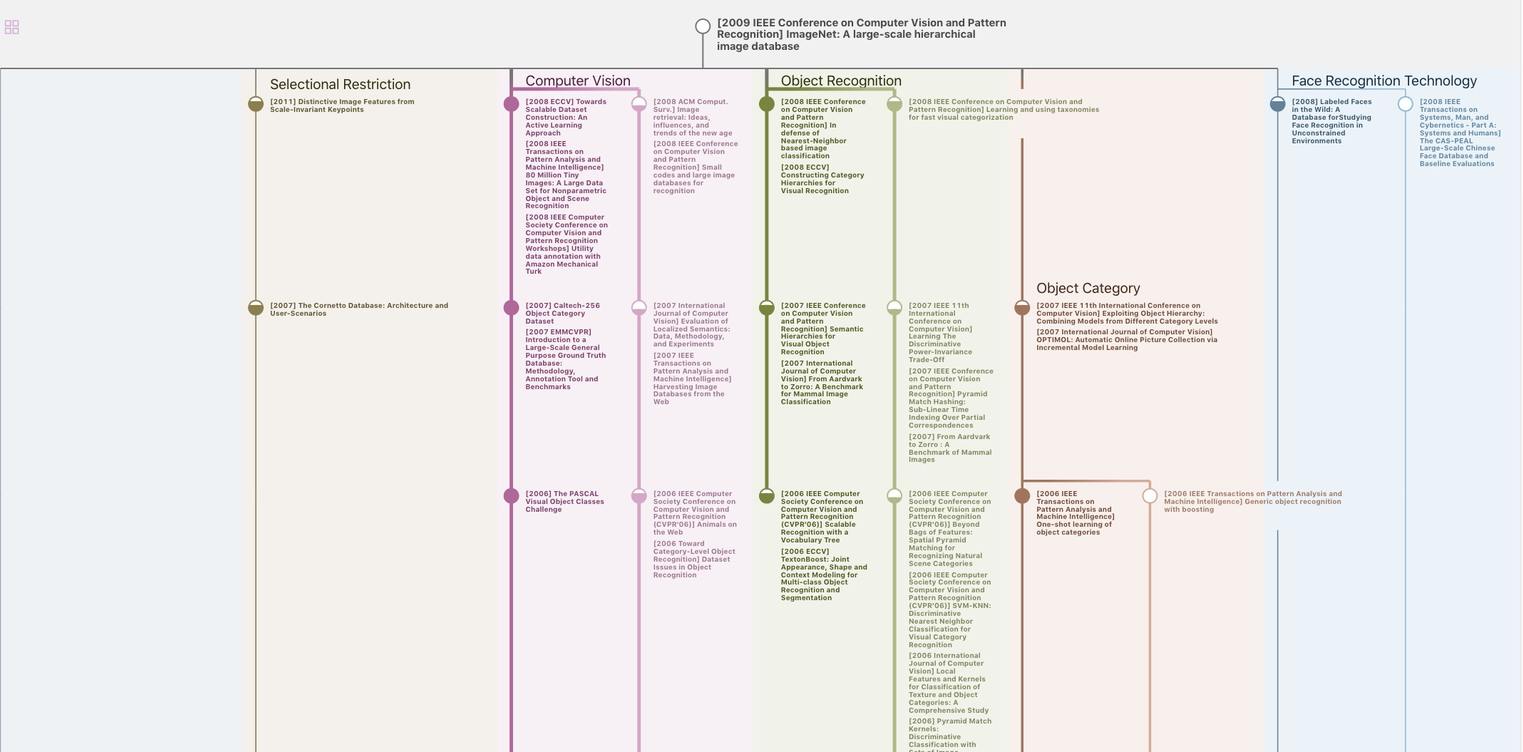
生成溯源树,研究论文发展脉络
Chat Paper
正在生成论文摘要