A Co-Melting Reduction Auto-Encoder
2020 7th International Conference on Information Science and Control Engineering (ICISCE)(2020)
摘要
The auto-encoder has been widely used in deep learning techniques as one of the unsupervised feature extraction algorithms. This paper proposes a Co-Melting Reduction Auto-Encoder (CMRAE) model to enrich extracted and release the burden of model parameters in image feature extraction. Firstly, a co-melting reduction structure is designed to realize feature fusion in the encoder, and it can reduce feature loss and model parameters in the decoder. Secondly, a multi-reconstruction loss function introduces reconstruction loss between feature layers, which can strengthen the connection between the feature layers and avoid the model premature effectively. The experiments prove that CMRAE can improve the feature extraction ability and efficiency of the model. The classification accuracy of features extracted by CMRAE is better than the state-of-the-art results on the datasets including Cats vs. Dogs (CvD), lung CT images, and multi-class weather (MWD).
更多查看译文
关键词
Auto-encoder,Feature extraction,Co-Melting reduction auto-encoder,Co-Melting reduction structure,multi-reconstruction loss function
AI 理解论文
溯源树
样例
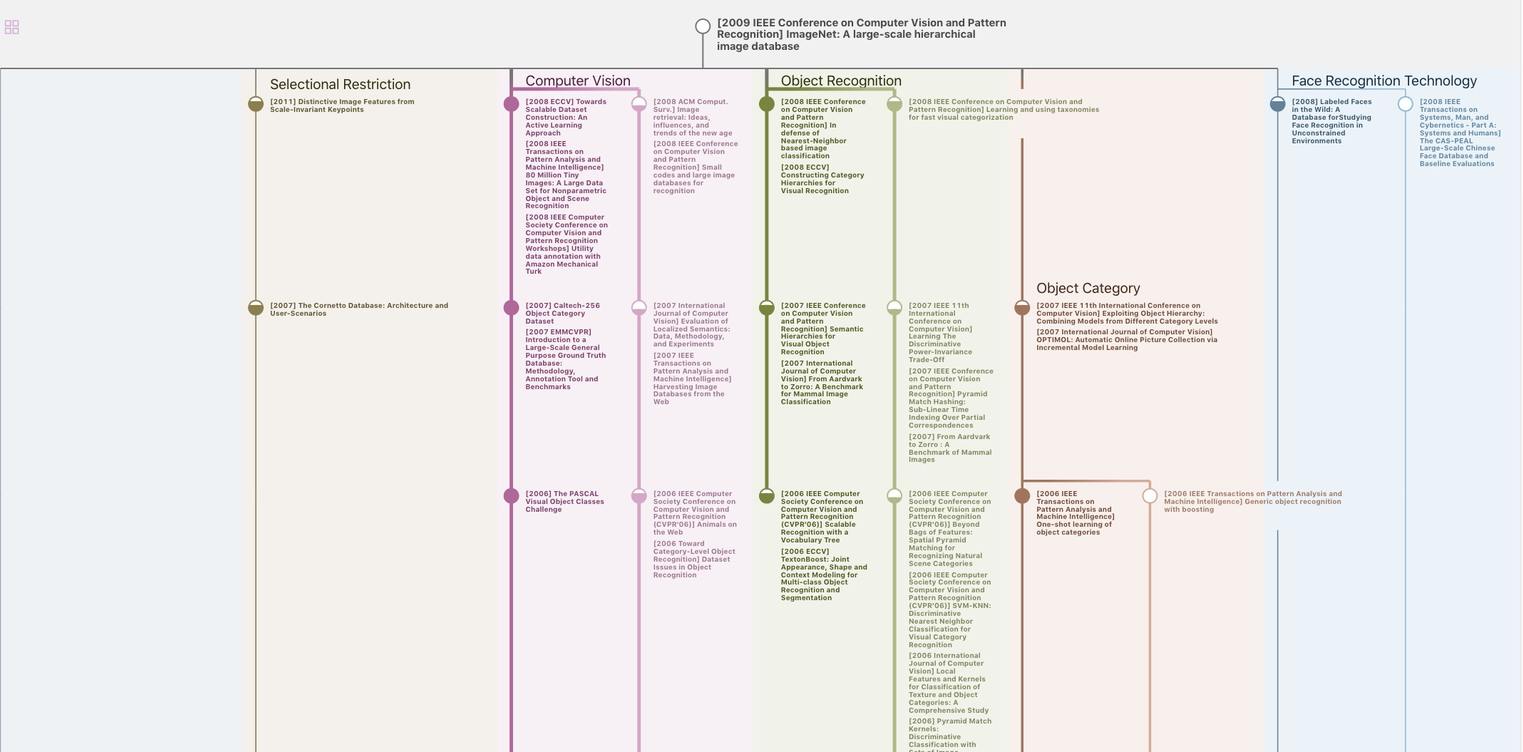
生成溯源树,研究论文发展脉络
Chat Paper
正在生成论文摘要