Populations of unlabelled networks: graph space geometry and generalized geodesic principal components
BIOMETRIKA(2024)
摘要
Statistical analysis for populations of networks is widely applicable, but challenging, as networks have strongly non-Euclidean behaviour. Graph space is an exhaustive framework for studying populations of unlabelled networks that are weighted or unweighted, uni- or multilayered, directed or undirected. Viewing graph space as the quotient of a Euclidean space with respect to a finite group action, we show that it is not a manifold, and that its curvature is unbounded from above. Within this geometrical framework we define generalized geodesic principal components, and we introduce the align-all-and-compute algorithms, all of which allow for the computation of statistics on graph space. The statistics and algorithms are compared with existing methods and empirically validated on three real datasets, showcasing the potential utility of the framework. The whole framework is implemented within the geomstats Python package.
更多查看译文
关键词
Some key words,Computational geometric statistic,Geodesic principal component,Graph space,Network data,Object data,Quotient space
AI 理解论文
溯源树
样例
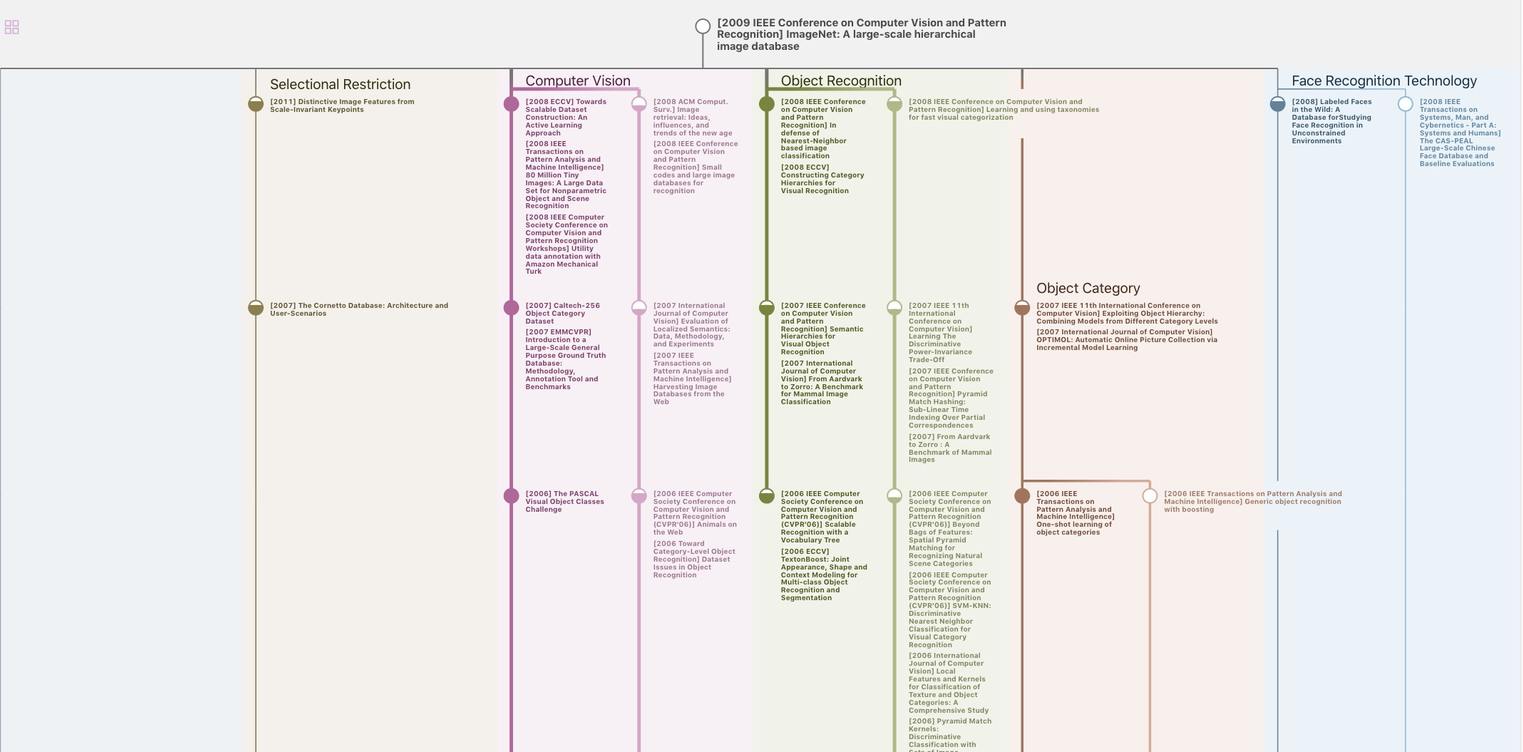
生成溯源树,研究论文发展脉络
Chat Paper
正在生成论文摘要