A Comparison of Data-Driven Models for Predicting Stream Water Temperature
semanticscholar(2020)
摘要
Changes to the Earth’s climate are expected to negatively impact water resources in the future. It is important to have accurate modelling of river flow and water quality to make optimal decisions for water management. Machine learning and deep learning models have become promising methods for making such hydrological predictions. Using these models, however, requires careful consideration both of data constraints and of model complexity for a given problem. Here, we use machine learning (ML) models to predict monthly stream water temperature records at three monitoring locations in the Northwestern United States with long-term datasets, using meteorological data as predictors. We fit three ML models: a Multiple Linear Regression, a Random Forest Regression, and a Support Vector Regression, and compare them against two baseline models: a persistence model and historical model. We show that all three ML models are reasonably able to predict mean monthly stream temperatures with root mean-squared errors (RMSE) ranging from 0.63-0.91 ◦C. Of the three ML models, Support Vector Regression performs the best with an error of 0.63-0.75 ◦C. However, all models perform poorly on extreme values of water temperature. We identify the need for machine learning approaches for predicting extreme values for variables such as water temperature, since it has significant implications for stream ecosystems and biota.
更多查看译文
AI 理解论文
溯源树
样例
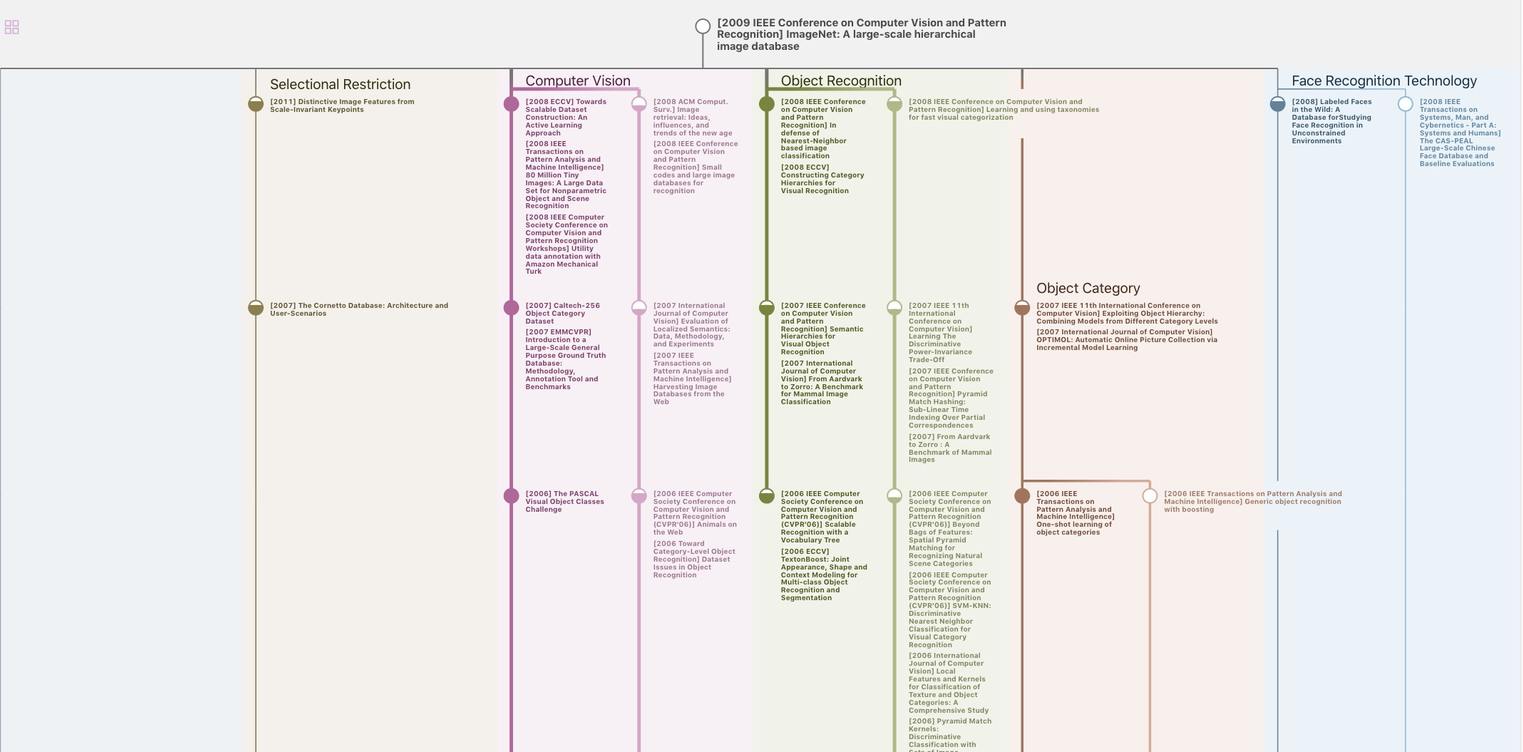
生成溯源树,研究论文发展脉络
Chat Paper
正在生成论文摘要