Low-cost Graphene-based Flexible Strain Sensor with a Novel Transfer Method
semanticscholar(2019)
摘要
Flexible strain sensors, as one of the important members of intelligent electronic devices, have attracted a lot of attention in areas such as structural health monitoring, smart wearable devices, and electronic skin. In structural health monitoring, the sensor is required to be able to adapt to the complex structure surface, and has a high sensitivity so that the micro cracks can be found in time. At present, the traditional metal strain sensors have short plates with large rigidity and low sensitivity, prompting the exploration of new strain sensitive mechanisms and materials. Graphene, as a novel material, has been continuously explored and found its good flexibility and sensitive strain piezoresistive effect. The sensor made of graphene has high sensitivity, but the high production and transfer cost of high-quality thin-layer graphene limits its application. In this paper, we report a new flexible strain sensor based on thick CVD graphene which is produced by a low-cost graphene film transfer strategy. A 150nm-thick nickel-based CVD graphene film was used as a strain-sensitive material, the poly (dimethylsiloxane) (PDMS) that was not completely dried was used to transfer graphene, and the dried PDMS was considered as the flexible substrate, which simplified the process and reduced costs. A tensile strain test with a range of up to 10% was performed and the sensor achieved a gauge factor (GF) of 130 under high strain. The conductive paths model based on the percolation network theory fit the experimental date accurately. Through the experiments above, we have obtained a low-cost graphene flexible strain sensor, which is able to monitor micro cracks and it has a suitable sensitivity and range. The sensor has important reference significance for the future research of complex structural health monitoring system, and has great potential value in the field of commercial applications.
更多查看译文
AI 理解论文
溯源树
样例
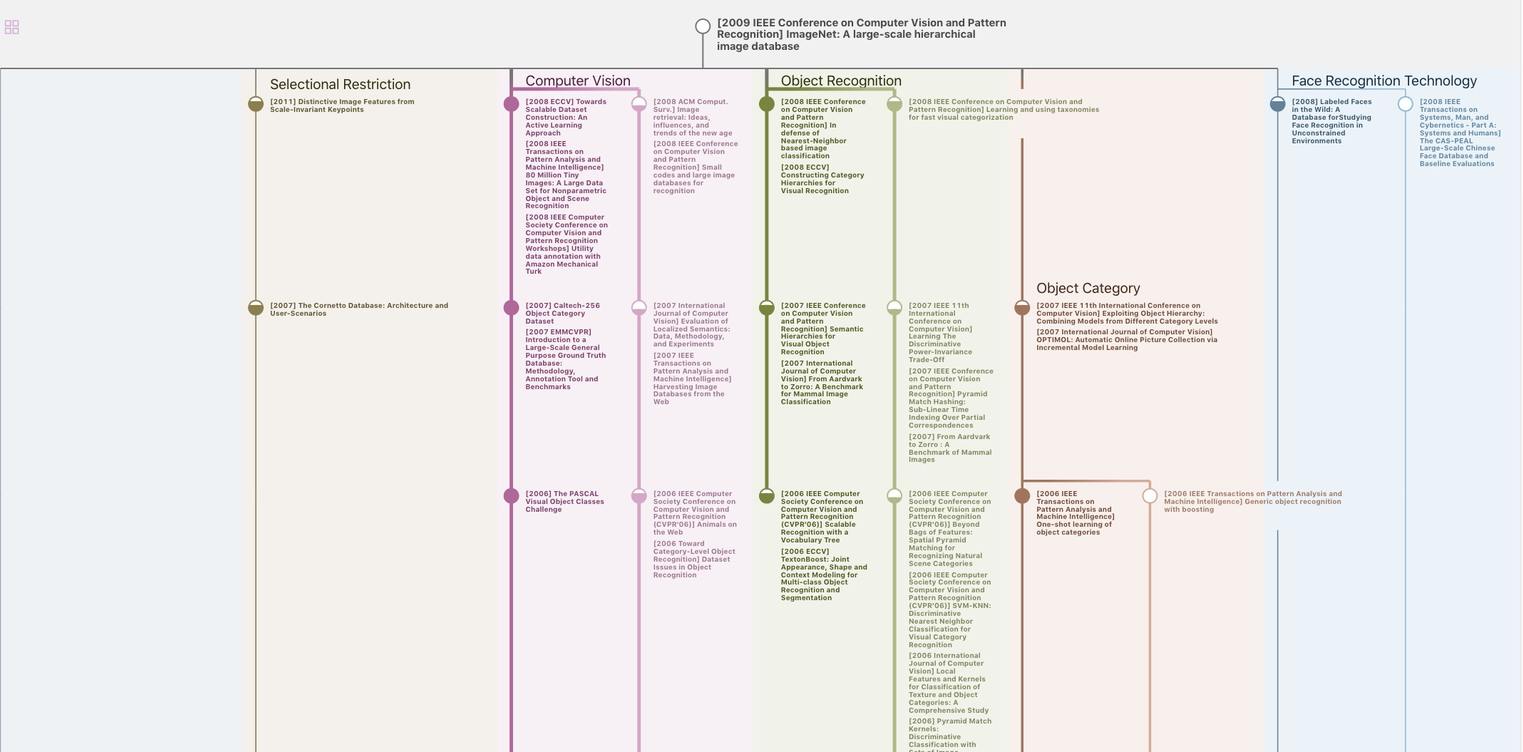
生成溯源树,研究论文发展脉络
Chat Paper
正在生成论文摘要