Weakly Supervised Microscopy Cell Segmentation via Convolutional LSTM Networks
semanticscholar(2020)
摘要
We address individual cells’ segmentation from microscopy sequences. The main challenge in this type of problems is not only foreground-background classification but also the separation of adjacent cells. We apply two orthogonal approaches to overcome the multiple instance problem. From the segmentation perspective, we adopt the three class loss used by [1], [2]. The segmentation representation is designed to enhance individual cells’ delineation by a partitioning of image domain into three classes: foreground, background and cell contours. From the detection perspective, we get our inspiration from [3], and aim to detect rough cell markers. The markers, as opposed to the full segmentation, do not cover the entire cell, but are rather a small ”blob” somewhere within the cell. The markers have two desirable properties. First, they are much smaller than the object and thus are easier to separate between instances. One marker will never overlap or touch boundaries with a neighboring marker. Second, the markers are easy to annotate, as the annotator does not need to be precise, making data acquisition a simpler task. Often, for microscopy image sequences, the only available annotation is in the form of markers or approximate cell centers. We train the proposed network to estimate both the segmentation and the markers and merge the two using the Fast Marching Distance (FMD) [4]. The entire framework is illustrated in Figure 1.
更多查看译文
AI 理解论文
溯源树
样例
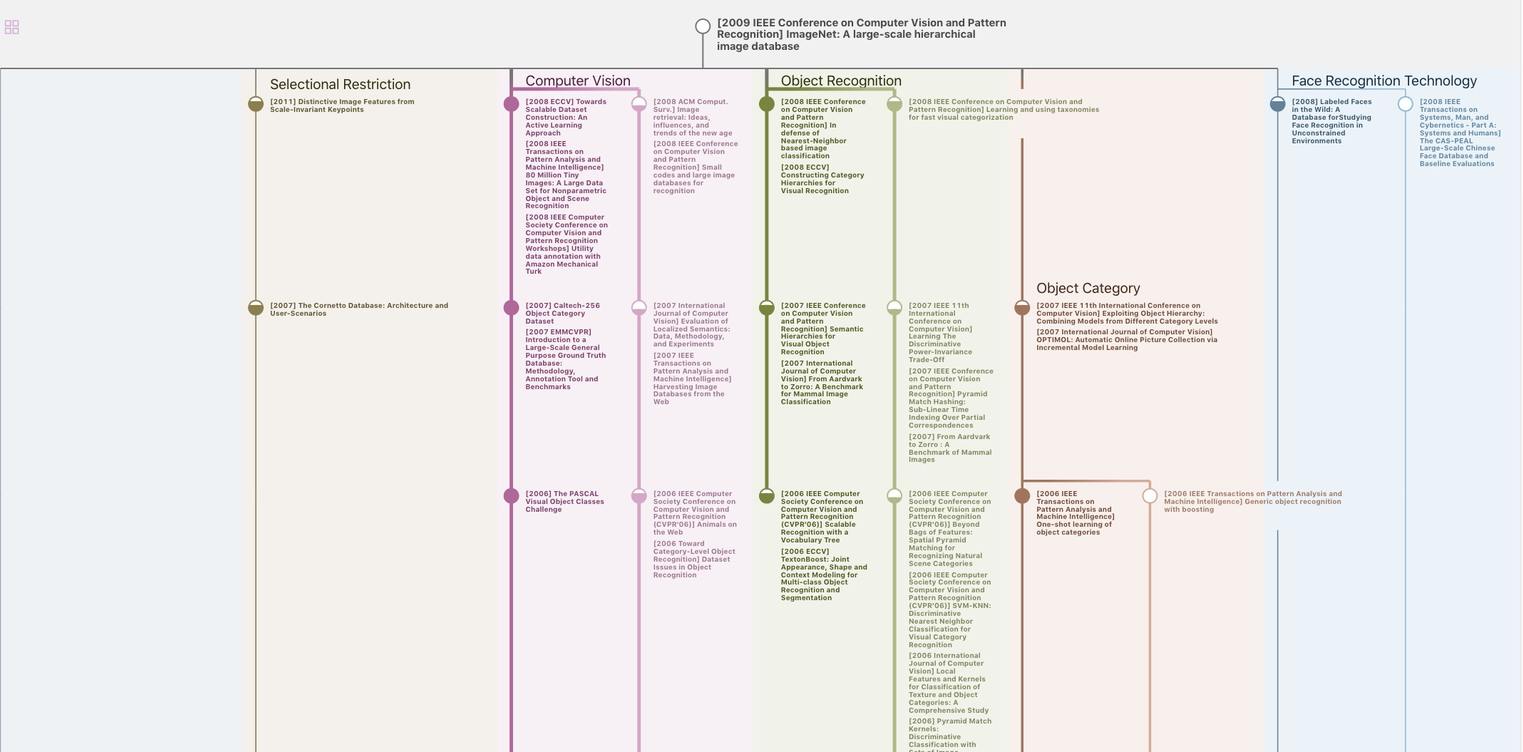
生成溯源树,研究论文发展脉络
Chat Paper
正在生成论文摘要