Diversification of Complementary Item Recommendations with User Preference in Online Grocery
semanticscholar(2020)
摘要
Complementary item recommendations play an important role in surfacing the relevant items to the customers in online grocery platform. Many research works focus on improving the recommendations through post-processing techniques such as user-item-level personalization and diversification of recommendations. In online grocery, complementary recommendations can fall into two types, cross-domain and within-domain. Cross-domain complementary recommendations refer to products that belong to two distinct categories, for instance, for query item hot dog bun, steak and barbecue sauce are cross-domain complementary items. On the other hand, for the same query item, chicken sausage and beef sausage form within-domain complementary recommendations as they are from delicatessen. Although diversification of recommendations can promote cross-domain complementary recommendations, it can’t capture the within-domain complementary recommendations. Within-domain complementary recommendations can not be replaced by similarity. Users also show their preferences on cross-domain or within-domain complementary items during online shopping, which can be indicated by their shopping behavior. However, many user-item-level personalization methods can’t explicitly model the preference of users on cross-domain and within-domain complementary recommendations. To stress the requirement of modeling cross-domain and within-domain complementary recommendations with personalization, we propose our re-ranking solution to provide fine-grained control of diversification on the complementary recommendations. We use transaction history to estimate users’ preference for the level of diversity in the complementary recommendations and combine it to our re-ranking solution. We demonstrate the effectiveness of our re-ranking algorithm on the publicly available Instacart dataset. Permission to make digital or hard copies of all or part of this work for personal or classroom use is granted without fee provided that copies are not made or distributed for profit or commercial advantage and that copies bear this notice and the full citation on the first page. Copyrights for components of this work owned by others than ACM must be honored. Abstracting with credit is permitted. To copy otherwise, or republish, to post on servers or to redistribute to lists, requires prior specific permission and/or a fee. Request permissions from permissions@acm.org. © 2018 Association for Computing Machinery. ACM ISBN 978-x-xxxx-xxxx-x/YY/MM. . . $15.00 https://doi.org/10.1145/1122445.1122456 CCS CONCEPTS • Information systems→ Information retrieval diversity; Similarity measures.
更多查看译文
AI 理解论文
溯源树
样例
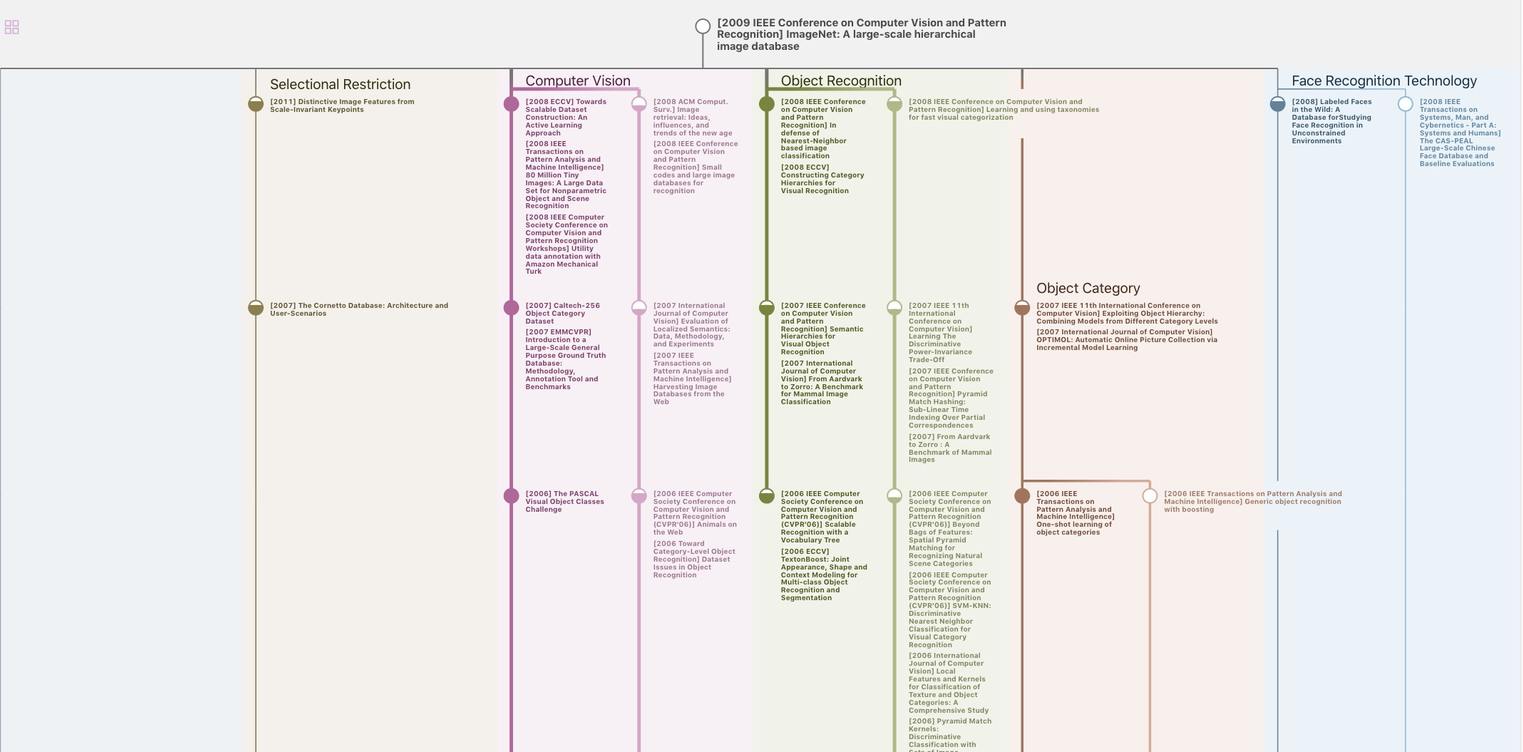
生成溯源树,研究论文发展脉络
Chat Paper
正在生成论文摘要